37% Better Output with 15 Lines of Code - Llama 3 8B (Ollama) & 70B (Groq)
Summary
TLDRفي هذا النص، يُعرض مستخدم تقنية الذكاء الاصطناعي لتحسين تجربة استعلام المستخدمين عن ملفات الوثائق. يُظهر مستخدم كيفية استبدال استعلامات غير محددة باستعلامات محددة بشكل أفضل من خلال إعادة كتابة السؤال الأصلي بإضافة سياق من محادثة سابقة. يُظهر هذا التحسين في استجابة نظام الذكاء الاصطناعي، حيث يُظهر نسخة ثانية من النموذج يحتوي على حل تحسيني. يُظهر مستخدم أيضًا مدى فائدة استخدام JSON في تحسين الإخراج المطلوب. يُشير إلى أن التحسين قد أدى إلى تحسين الاستجابة بنسبة تتراوح بين 30% إلى 50%، وفقًا لتقييمات GPT-4. يُشير إلى وجود تحديثات جديدة في مشروع GitHub للغة الذكاء الاصطناعي (AMA)، ويُتوقع مشاركة مستخدم في مقاطع الفيديو القادمة لمناقشة Gro ونموذج Llama 70B.
Takeaways
- 🚀 **使用RAG系统提问**: 视频展示了如何通过RAG系统向文档提问,例如询问Meta的AI模型Llama 3的训练细节。
- 🔍 **处理模糊问题**: 讨论了如何处理模糊或不具体的问题,如“这是什么意思?”,并展示了如何改进以获得更好的答案。
- 💡 **查询重写解决方案**: 实现了一个查询重写的解决方案,通过增加上下文来改善问题,从而获得更丰富的答案。
- 📈 **模型性能对比**: 展示了在8B和70B版本的Llama模型上运行相同查询的结果,并比较了它们的性能。
- 📚 **赞助商介绍**: 视频中提到了赞助商Brilliant.org,这是一个学习平台,提供数学、编程、AI和数据分析的互动课程。
- 🔧 **代码和逻辑解释**: 视频中深入探讨了实现查询重写的代码和逻辑,提供了详细的步骤和解释。
- 📝 **JSON的使用**: 为了确保输出的结构化,视频中使用了JSON来组织和传递查询信息。
- 🔗 **GitHub资源**: 提到了GitHub仓库的更新,包括使用Dolphin Tree Llama模型和AMA嵌入模型的更新。
- 📦 **模型选择**: 讨论了如何从终端选择不同的模型,增加了操作的灵活性。
- 🤖 **Gro和Llama 70B模型测试**: 视频最后测试了使用Gro和Llama 70B模型的系统,展示了重写查询的效果。
- 🎯 **改进的响应质量**: 通过比较有无重写查询的响应,得出了重写查询可以提高响应质量30-50%的结论。
Q & A
视频中提到的问题是关于什么?
-视频中提到的问题是关于如何改进一个基于文档的问答系统,使其能够更好地处理模糊或不具体的查询。
Llama 3 在训练时使用了大约多少个token?
-Llama 3 在训练时使用了大约15万亿个token。
视频中提到的解决方案是什么?
-视频中提到的解决方案是重写查询(Rewritten query),通过增加更多的上下文信息来改善查询的明确性和信息量。
重写查询的目的是什么?
-重写查询的目的是保留原始查询的核心意图和含义,同时扩展和澄清查询,使其更具体、更有信息量,以便检索到相关的上下文。
视频中提到的赞助商是谁?
-视频中提到的赞助商是Brilliant.org,一个提供数学、编程、人工智能和数据分析课程的在线学习平台。
如何使用赞助商Brilliant.org来提高编程技能?
-通过Brilliant.org的互动课程,用户可以学习Python编程,并从第一天开始构建程序,同时学习循环、变量、嵌套和条件等基本编码元素。
视频中提到的AMA shat函数是什么?
-AMA shat函数是视频中提到的系统中用于处理用户查询并生成重写查询的一部分。
为什么视频中的作者对使用JSON感到满意?
-作者对使用JSON感到满意,因为它提供了一个更确定的输出结构,确保了输出的一致性和可预测性。
视频中提到的GitHub项目是什么?
-视频中提到的GitHub项目是一个名为'super easy 100% local AMA rag'的本地运行的问答系统,使用了Llama模型。
作者在视频中提到了哪些模型的比较?
-作者比较了使用8B Llama 3模型和70B Llama模型的重写查询的效果,发现70B模型生成的重写查询效果更好。
如何评估重写查询的效果?
-作者通过将没有使用重写查询的响应与使用重写查询的响应进行比较,多次询问GPT-4模型,发现使用重写查询的响应通常比未使用的响应好30%到50%。
Outlines
🔍 Introduction to the Problem and Solution
The speaker begins by introducing a problem they encountered with their AI system, specifically when asked vague questions that did not pull relevant context from documents. They then demonstrate their solution, which involves rewriting queries to provide more context and specificity, thus improving the AI's ability to retrieve relevant information. The speaker also mentions testing this solution on different models of AI, including the 8B and 70B models.
🛠️ Explaining the Query Rewriting Process
The speaker provides a step-by-step explanation of how they implemented the query rewriting process. This includes receiving user input, parsing JSON, extracting the original query, constructing a prompt for the AI model, and feeding the rewritten query back into the system to retrieve relevant context. The use of JSON ensures a structured and deterministic output, which aids in the clarity and effectiveness of the rewritten queries.
📈 Updates and Testing with Llama 70b Model
The speaker discusses updates made to their GitHub repository, including changes to the model and embeddings. They share their experience with testing the rewritten query process using the Llama 70b model, noting that it produced better results than previous models. The speaker demonstrates the improved query by asking questions and showing how the rewritten queries lead to more detailed and informative responses.
📊 Measuring Improvement and Future Plans
The speaker reveals how they measured the improvement in responses, by comparing rewritten queries to original queries using GPT-4 and Ophidian, which showed an improvement of about 30-50%. They express gratitude for the support they've received and encourage viewers to check out their GitHub for updates. They also hint at future plans to work more with the Gro and Llama 70b model, pending resolution of rate limit issues.
Mindmap
Keywords
💡RAG system
💡Tokens
💡Vague question
💡Rewritten query
💡AMA (Ask Me Anything)
💡Llama 3 Model
💡Json
💡Brilliant.org
💡AI model
💡GitHub
💡Llama 70b model
Highlights
The speaker is introducing a problem they wanted to solve regarding handling vague questions in an AI system.
They demonstrate the AI system's initial inability to provide context for vague queries.
The speaker presents a solution involving a rewritten query to provide more context to vague questions.
The AI model, Llama 3, is shown to provide an answer after the query is rewritten, improving the response.
The speaker explains the process of rewriting queries using conversation history to improve specificity.
A step-by-step explanation of the code and logic behind the query rewriting process is provided.
The use of JSON for structured output is highlighted as a key component of the solution.
The speaker discusses the improvements made to the AMA chat function to incorporate the rewritten query feature.
The impact of using a larger AI model, Llama 70B, on the quality of the rewritten queries is explored.
The speaker shares the results of comparing responses with and without the rewritten query, showing an improvement of 30-50%.
The practical application of the rewritten query feature is demonstrated through a live example using the Llama 70B model.
The speaker provides a humorous estimate of how many books a human would need to read to match Llama 3's training data.
The importance of the project for improving AI's ability to understand and respond to vague human queries is emphasized.
The speaker expresses satisfaction with the current state of the project and its potential for further development.
Updates to the GitHub repository related to the project are mentioned, inviting interested individuals to explore and contribute.
The speaker teases an upcoming video featuring more work with Gro and the Llama 70B model, subject to overcoming rate limit issues.
A call to action for viewers to support the project by starring the GitHub repository is included.
The video concludes with an invitation to join a subsequent live session and well wishes for the viewers' week.
Transcripts
today I'm going to start by showing you
the problem I wanted to solve I want to
show you how I tried to solve it and if
it was a success and then I'm going to
explain it to you so you can understand
it and start using this too so yeah
let's just get started okay so what you
see here is me I have fired up my rag
system so we can start asking questions
about my documents so I fed in some
information about meta's AI llama 3
right I asked the question how many
tokens was llama 3 trained on and we
have the context that is pulled from the
document
and we use that context to kind of
answer llama 3 was preened on 15
trillion tokens okay so far so good
right and here comes kind of my problem
uh it's not a big problem right if you
know what you're doing but what happens
when I say what does that mean a very
vague question right okay so we say that
no we don't pull anything from our
documents right so that means that we
don't have any relevant context to this
problem so this is kind of the problem I
wanted to take a look at today how can
we kind of improve this so yeah I'm just
going to show you how I implemented a
solution for this and how it works okay
so let's fire up the second version here
so this is the version that contains my
solution so we're going to ask the same
question how many tokens was llama 3
trained on uh this is running on the 8B
llama 3 Model on AMA so it's totally
locally right and you can see see the
Llama Tre was trained over 50 million
tokens so pretty much exactly the same
answer as before what if we say what
does that mean so a very vague question
right so what I implemented was this
kind of Rewritten query so we take our
original query and we try to rewrite it
so can you provide more details about
the improvements made in llama 3
compared to its predecessor increasing
training data code size support for non-
languages also how does a tokenizer yeah
blah blah blah so you can see we added
much more context to our query uh just
by putting this through some kind of
solution I'm going to show you and now
you can see we get context pull from the
documents even though our query was kind
of the same and yeah you can see we get
a pretty good answer here I'm not going
to read it but you can pause and read if
you want to so yeah I'm pretty happy how
this works out this is of course not
like a perfect solution but uh for me
this has improved the responses a bit at
least in this very small model I haven't
tried it too much we're going to try it
on the 70b model later uh this video but
for now yeah I'm pretty happy with this
so uh I think we're just going to head
over and try to explain how this works
because a lot of you enjoy that in the
previous video going a bit deeper into
the code and explaining the logic and
kind of how this works so yeah let's do
that but first let's say you are one of
those that wants to learn more about
about Python and computer science then
you should really pay attention to
today's sponsor brilliant have you ever
wondered how to make sense of fast
amounts of data or maybe you're eager to
learn coding but you don't know where to
start well brilliant.org the sponsor of
today's video is the perfect place to
learn these skills Brilliance is a
learning platform that is designed to be
uniquely effective their interactive
lessons in math programming Ai and data
analysis are created by a team of
award-winning teachers professionals and
researchers if you looking to build a
foundation in probability to better
understand the likelihood of events the
course introduction to probability is a
great place to start you work with real
data set from sources like Starbucks
Twitter Spotify learning to parse and
visualize massive data sets to make them
easier to interpret and for those ready
to level up their programming skills the
creative coding course is a must you'll
get familiar with python and start
building programs on day one learning
essential coding elements like Loops
variables nesting and conditionals what
set brilliant apart is that it helps you
build critical thinking skills to
problem solving not just memorizing so
while you are getting knowledge on
specific topics you're also becoming a
better Tinker overall to try everything
brilliant has to offer for free for 30
days visit brilliant.org allout AI or
just click the link in the description
below you will also get a 20% of an
annual premium subscription a big thanks
to brilliant for sponsoring this video
now let's go back to the project okay so
you can see from the code here these
lines here and a few lines down here in
our AMA shat function was kind of all I
added to try to solve this problem if
you can call it a problem uh but yeah
that was something I wanted to try to do
so uh I'm just going to explain quickly
how this works or not quickly I'm going
to go into a bit of a detail but you can
see we have a pretty long prompt here so
I'm going to blow this up so you can see
it better and then we're going to move
on and kind of go through step by step
now how this actually works so yeah
hopefully you can learn learn something
about this I want to start by explaining
how I thought about this prompt we used
for this so uh basically I'm just going
to go through it and explain it so you
can see rewrite the following query by
incorporating relevant context from the
conversation history so we are actually
using bits from our conversation history
the two previous messages to try to
improve our query so the Rewritten query
should preserve the core intent and
meaning of the original query expand and
clarify the query to make it more
specific and informative for retrieving
relevant context avoid introducing new
topics and queries that deviate from the
original query don't ever answer the
original query but instead focus on
rephrasing and expanding it into a new
query return only the Rewritten query
text without any additional formatting
or explanations then we're going to pass
in our context so we're going to pass in
our true previous messages then we're
going to pass in our original query from
the user input and then we are want our
Rewritten query as the output right and
that is kind of how I set this prompt up
so of course this is important uh but we
are using some help from Json here to
get the structure output we want so that
is kind of what I wanted to explain to
you in this stepbystep process here okay
so let's start on step one here so
receive user input Json the function
receives a Json string containing user's
original query for example query and
what does that mean right how this is
being put into a rewrite query function
is in the AMA shat function here so if
we take a look here so I kind of set
this up so the first query we make to
our rag system is not going to have a
Rewritten query because I found out that
was pretty stupid we don't need that but
from the second query we put in
everything is going to be Rewritten so
you can see here is our function and
we're going to pass in this Json here
that comes from this one right so we can
see we have the user input here okay so
in step two here we're going to par the
Json to a dictionary so the Json string
is converted to a python dictionary
using Json loads so this could for
example be user input equals and then we
have a query and uh yeah the parameter
for the query could be what does this
mean okay and then we kind of move on to
step three that is going to be
extracting the original query from this
python dictionary so let's say we have
this as a python dictionary now we kind
of want to grab the query right so the
user input now is equal to what does
that mean because we grabbed it from
this python dictionary up here right The
Next Step then is going to be step four
and this is preparing the prompt for the
AI model a prompt is constructed that
includes a conversation history and
instruction for rewriting the query we
already took a look at that right up
here so we kind of know how this prompt
Works uh and you can see in Step file we
want to call our AI model so in this
case this is AMA running on llama 3 uh
is called with a prepared prompt the
model generates a Rewritten version of
the query and if we move on to step six
that is going to be extracting the
Rewritten query so the Rewritten query
extracted from the models response if
you take a look at the code here that is
going to happen yeah here right so
Rewritten query and we have the response
from the model right we feed in our
prompt and we kind of get this Json dump
Rewritten query out and here we pass in
our Rewritten query from the response
from the model right and that is of
course going to be step seven so return
Rewritten query in Json a new Json
string is constructed containing the
Rewritten query and return to the
calling function so this could be for
example Rewritten query right like we
saw down here Rewritten query and we can
maybe the parameters is going to be what
does it mean that llama 3 has been
trained on 15 trillion tokens and that
means that we're ready for kind of our
final step and that is going to be to
feed this Rewritten query back to uh or
to the get re elant context function
down in our AMA chat function right so
you can see Rewritten query here and
this is going to be fed back into the
get relevant context right so if we go
down here you can see we are feeding a
relevant query here into the get
relevant context function and we are
skipping all together uh the user the
original user input or the original user
query quy is not going to be fed into
the relevant context so we only going to
pass in a Rewritten query right so you
can see the Rewritten query is passed to
get to the get relevant context function
which retrieve relevant context from the
knowledge Vault based on the Rewritten
query and that is kind of how I set this
up so uh like I said the original user
query here is not going to be even
consideration even though we print it uh
that is just to compare it
if we take a look here you can see we
print it here but we are not going to
pass it into any functions so we only
going to print it so we can kind of
compare them here side by side just for
fun I guess so yeah that is kind of how
I set this up and so far I'm been pretty
happy with it I hope it was uh okay to
kind of understand how this works so it
really helps using Json because that
gives us a more deterministic output
so we will always kind of get this very
structured form and if I tried to use I
tried to not use Json but that was not a
great success but you can try that if
you want to uh but for me this has been
working pretty okay so this code is
going to be H kind of an extension of my
the GitHub repo you can see on the
screen here which is the super easy 100%
local uh AMA rag uh so we made some
updates uh what we updated was we are
using the dolphin tree llama model now
uh we have an update that we change our
embeddings models so we are actually
using a AMA embeddings model now and
that has been working out pretty good we
have a few other updates so we can kind
of pick our model from the terminal line
if we want to do that yeah just some
issues we had that I got on the GitHub
that I have implemented uh of course
this is just a a layout so you can do
whatever you want with this you can find
the link in the description I'm probably
going to put put this video up and
explaining all the updates to the code
here so this should be code should be up
now so you can start playing around with
it and yeah I hope you kind of enjoyed
it so I kind of wanted to finish this
video by I created a local rag version
here using Gro and the Llama 70b model
uh I was supposed to do a video today
using grock and llama 7B but I had so
many issues with the rate limit so I had
to skip it that might be for Sunday we
will see but let's just finish up this
video by testing this using the gro and
the lava 70b so yeah this is basically
exactly the same uh I found that the
Rewritten queries were a bit better so
let's just try the same questions so how
many
tokens was this you can see it's pretty
fast though we're running right uh okay
so let's do what does that mean okay so
let's take a look at the Rewritten query
here what does that mean what does it
mean that llama 3 was trained on
enormous data set uh equivalent of two
to billion
books 13 tokens what is the impact
moldability so you can see yeah this is
a much better Rewritten query right this
is good so let's see the answer
here uh okay here is a breakdown of what
it means 15 trillion tokens refers to
the massive amount of data using okay T
stands for
trillions uh okay so this is great
tokens are indidual unit of text such as
words and characters the mother train a
huge data set wow this is good right so
we got all of this just by asking what
does this mean so you can see how
actually how good this Rewritten query
is and of course the better model we are
going to use the better answer we are
going to get right in summary llama 3 is
a highly adapt highly Advanced language
model trained on enormous data set with
focus on simplistic scalability and high
quality
data uh let's do wow
that's crazy how many books must a human
read to be this smart that's a bad
question um what's the equivalent amount
of human reading in terms of number of
books that would be required to achieve
the same L understanding knowledge L Tre
train 15 trillion tokens of data again a
very good Rewritten Qui if you ask me uh
what a question to put it at scale and
it goes
into uh okay so let's
say to read
330,000 to 600,000 books it would take
around
16,500 to 30,000 years assuming one book
per week uh around 15,000 years assuming
two bucks per week of course this is a
rough estimate them meant to be humorous
this model is so good so yeah you can
see we have to read uh around how many
books was it 600,000 books to be this
smart so yeah uh so I I think this kind
of shows how good this uh Rewritten
query kind of is and yeah how good the
70b model is so really excited about
llama Tre uh hope you found this
enjoyable hope you learned something
from it it that's the most important
thing the result doesn't matter too much
but maybe this give you some new ideas
how you can use embeddings to kind of
improve stuff how you can use uh the get
relevant context function to do other
stuff so yeah so I guess a lot of you
wondering where I got the 30% better
response from so what I did is I took
one response uh without the rewrite
query and I took like a second response
with the rewriting query function and I
asked the first GPT 4 to compare them
and I asked a lot of times and most of
the times it came in between 30 and 50%
better response than response one so
response two is the one with the rewrite
query and I did the same on Opus and
here it always landed in 30 40% better
than response one so response two that
was with the uh yeah reite query
function so yeah that is where I got the
37% from just want to say big thank you
for the support lately it's been awesome
and give the GitHub a star if you
enjoyed it other than that come back for
Sunday probably going to do more Gro and
llama 70b if the rate limit is okay have
a great week and I see you again yeah
Sunday
5.0 / 5 (0 votes)
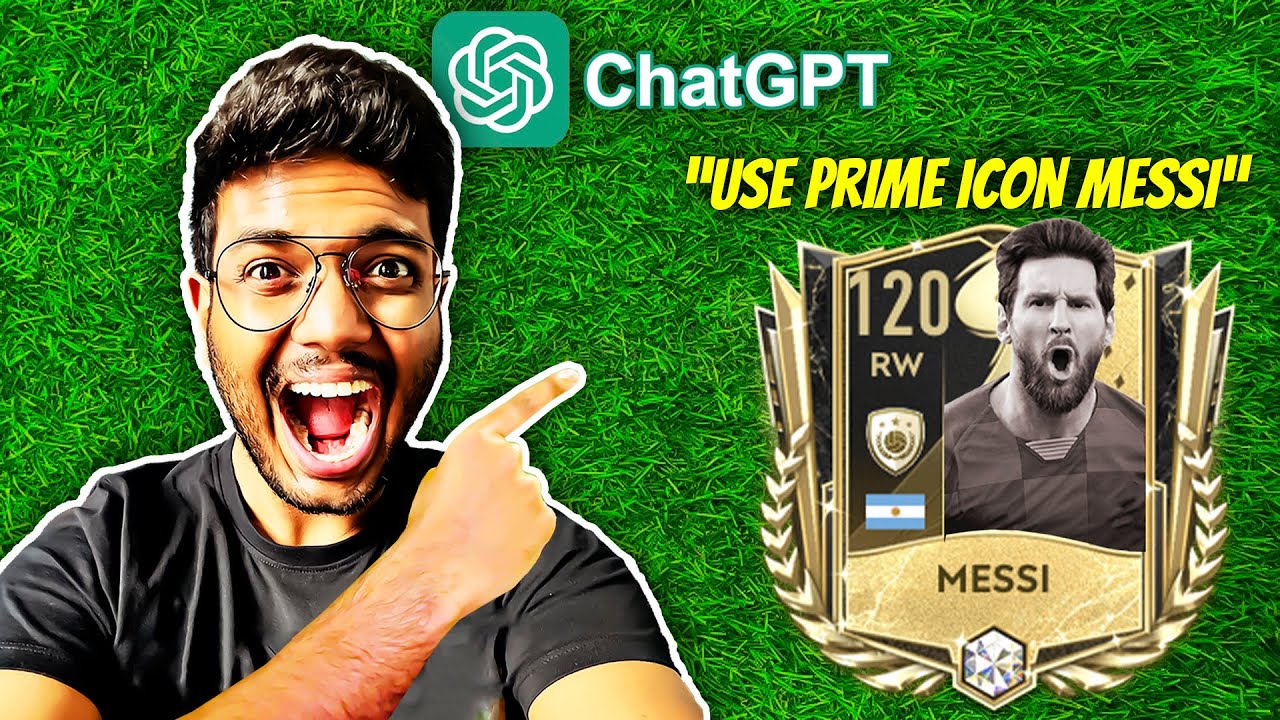
ChatGPT Built my FIFA MOBILE Squad!
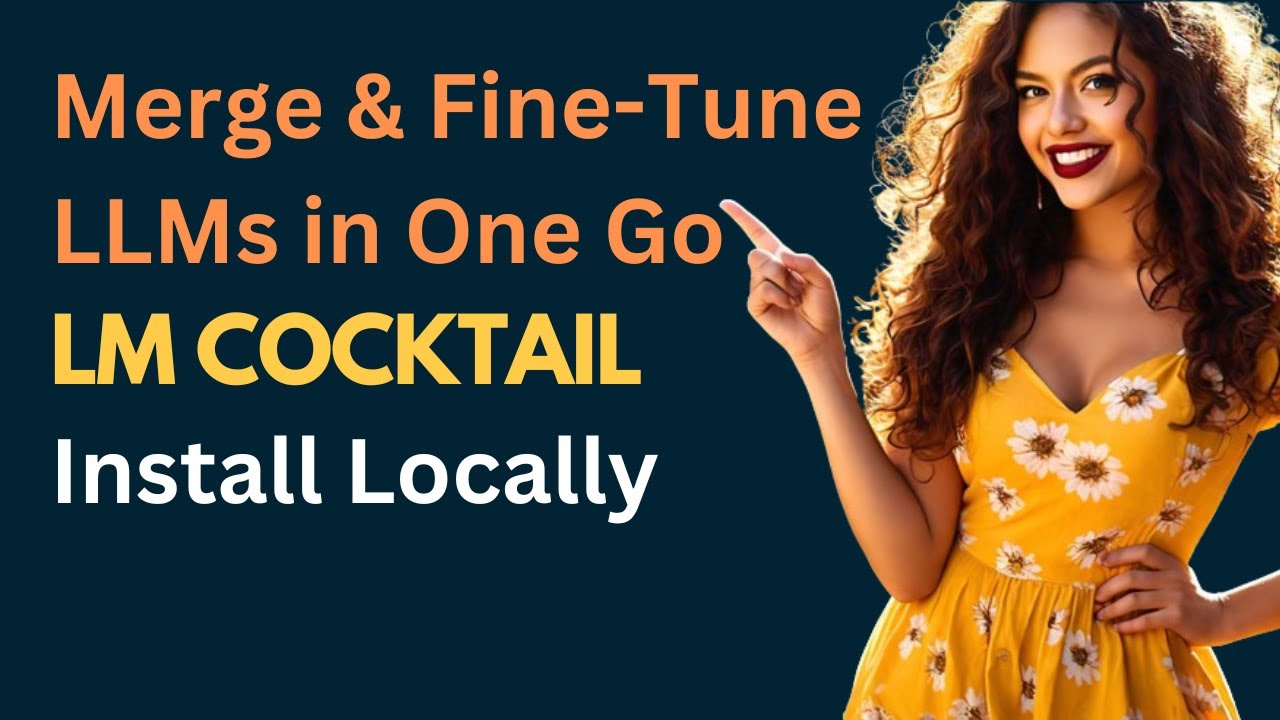
Merge Models Locally While Fine-Tuning on Custom Data Locally - LM Cocktail
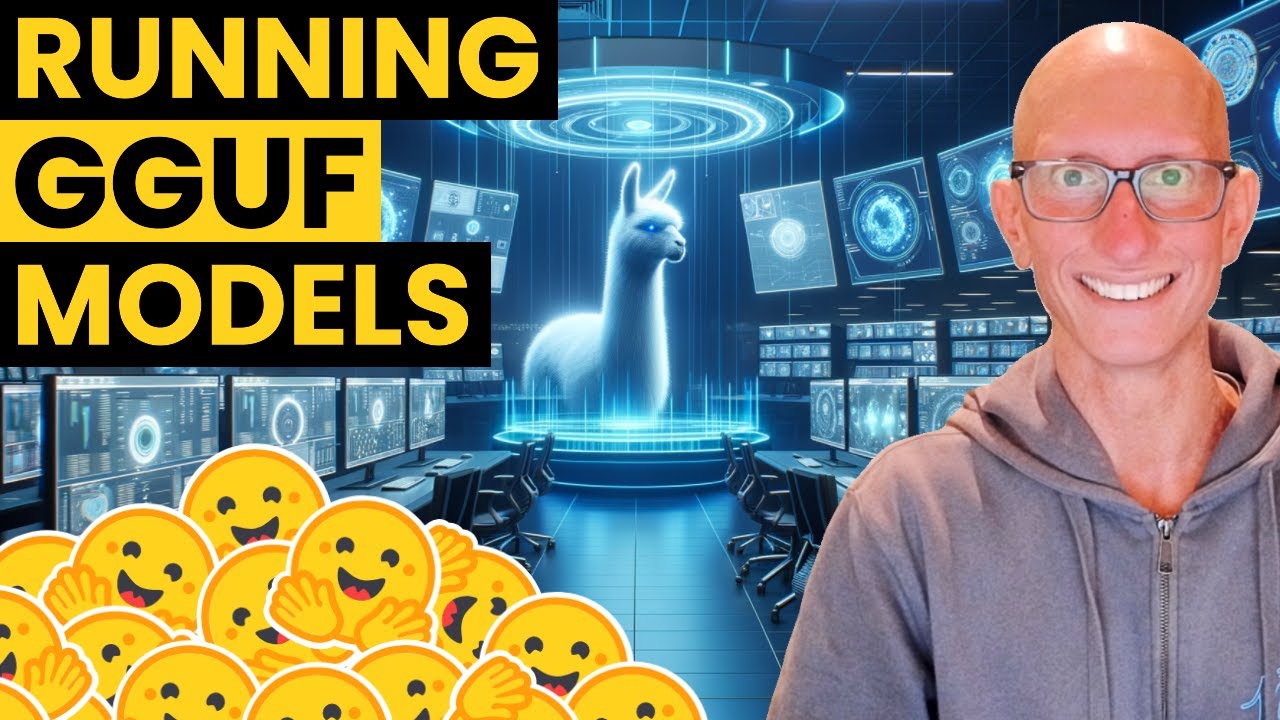
Hugging Face GGUF Models locally with Ollama
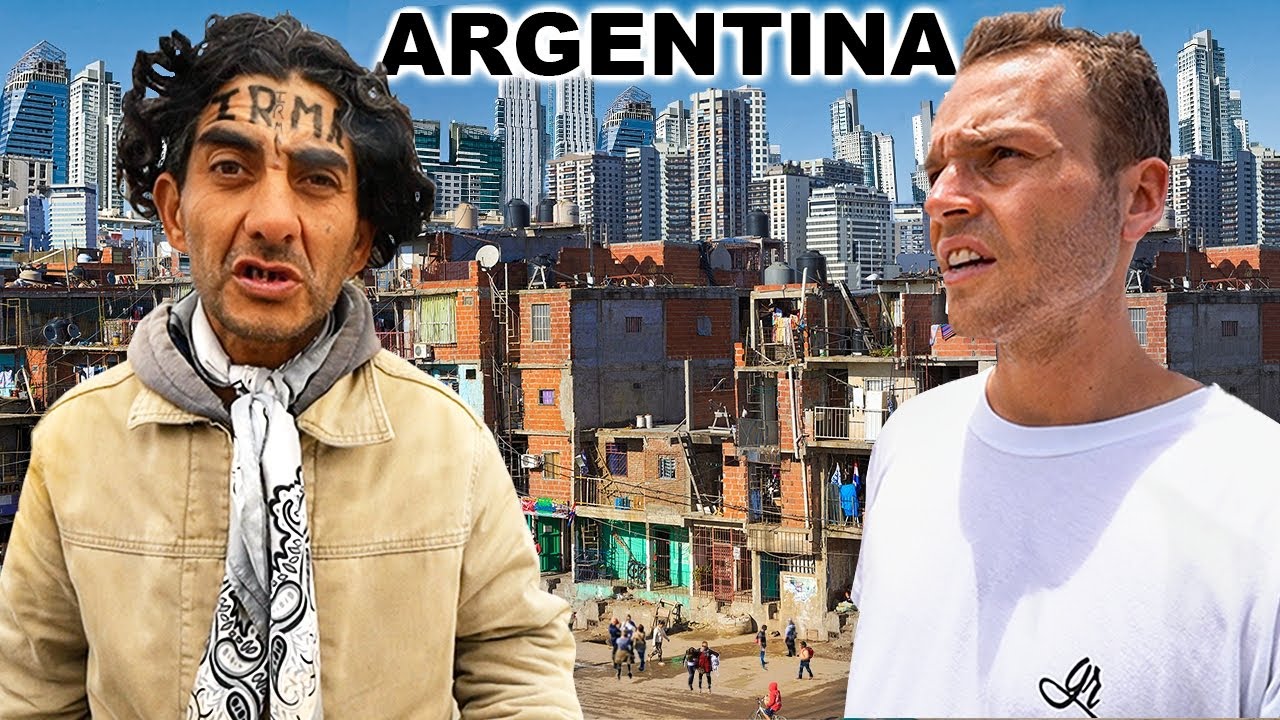
Inside Argentina's Most Dangerous Neighborhood ($40 per month rent)
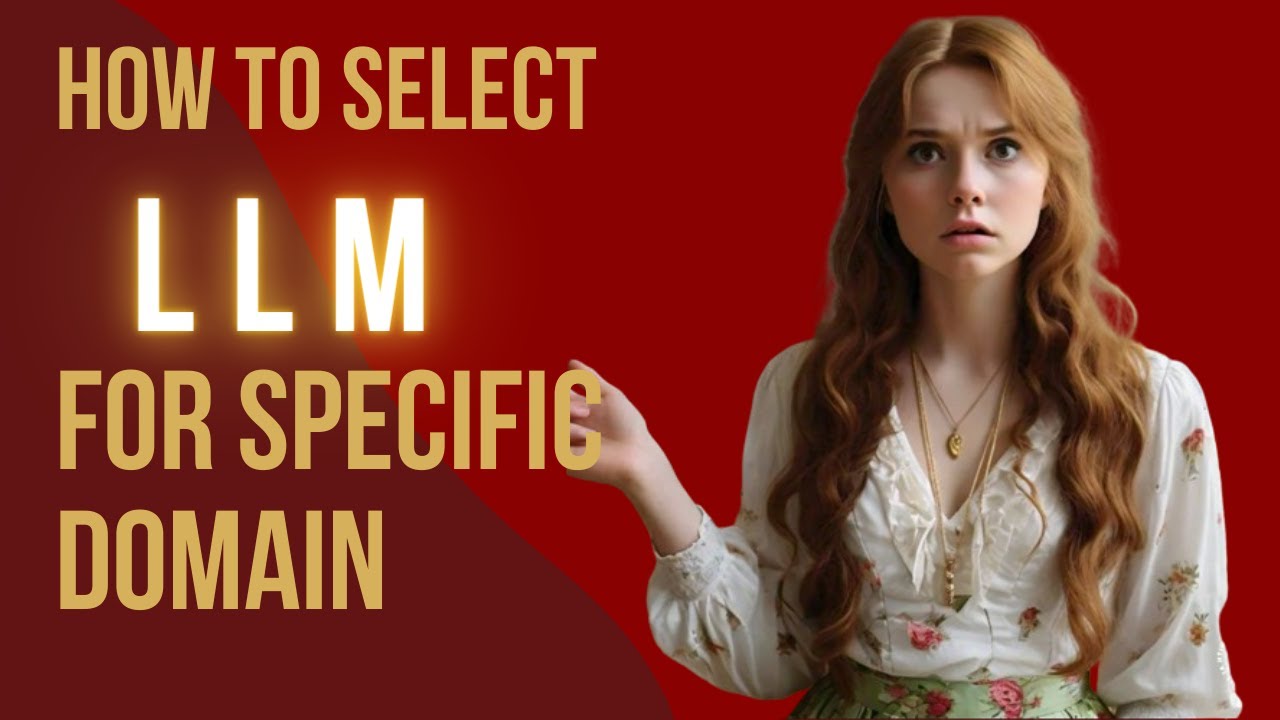
How to Select an AI Model for Specific Domain or Task
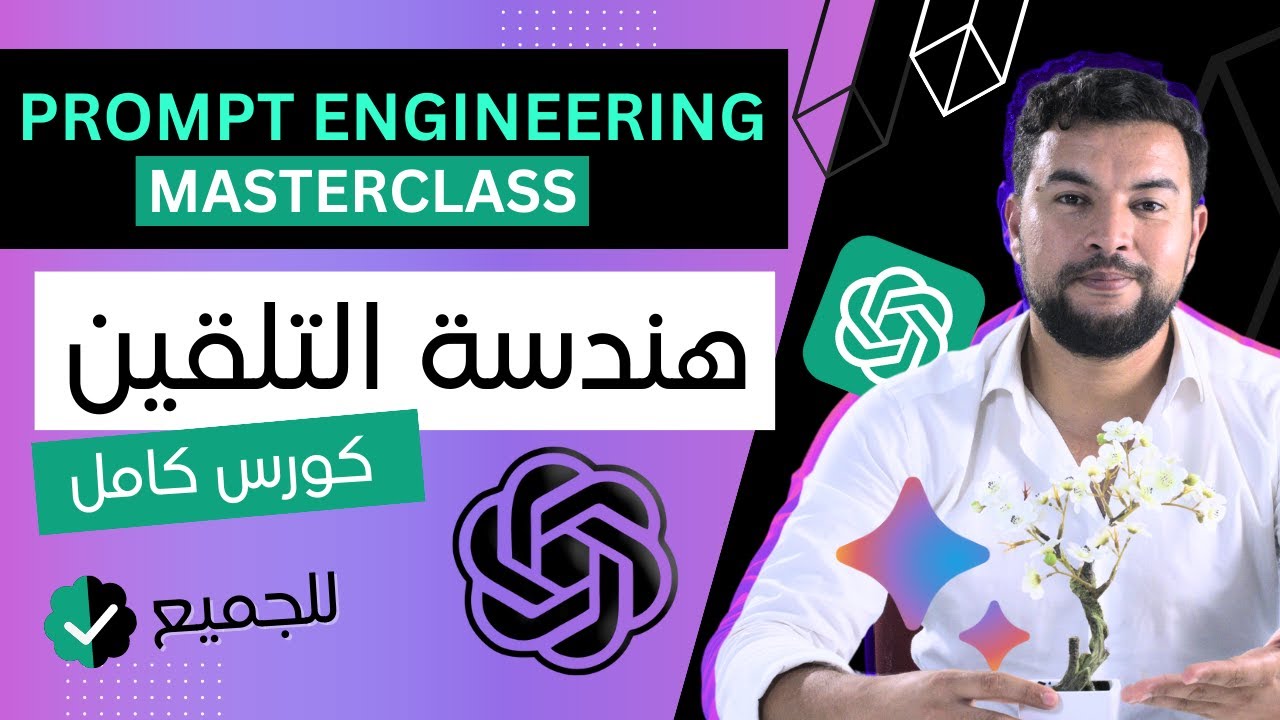
كورس هندسة التلقين | Prompt Engineering MasterClass