FSD v12: Tesla's Autonomous Driving Game-Changer w/ James Douma (Ep. 757)
Summary
TLDRIn this engaging discussion, Dave and James delve into the recent developments at Tesla, focusing on the release of FSD V12 and the anticipated reveal of the Optimus robotaxi in August. They share firsthand experiences with FSD V12, noting its impressive capabilities and smoother performance compared to its predecessor. The conversation also explores the potential of Tesla's AI technology, the challenges of scaling up robot production, and the impact of competition in the AI field. The discussion highlights the rapid advancements in AI and the transformative potential of Tesla's upcoming projects.
Takeaways
- 🚗 Tesla's FSD V12 release has shown significant improvements over previous versions, surpassing initial expectations.
- 🌟 The V12 update introduced a drastic rewrite of Tesla's planning architecture, enhancing the overall driving experience.
- 🧠 The neural network's ability to generalize from mimicking human driving behaviors has led to a more natural and smoother ride.
- 🔧 Tesla's approach to developing FSD involves an end-to-end process, which has proven to be more sample-efficient and scalable.
- 🚀 The potential for FSD to reach superhuman driving capabilities is evident as the system continues to learn and improve.
- 🤖 The development of Tesla's humanoid robot, Optimus, is ongoing, with a focus on perfecting the hardware before scaling production.
- 📈 The importance of data gathering in refining AI models like FSD and Optimus cannot be overstated, with real-world variability being crucial for training.
- 🌐 Tesla's strategy for robotaxis involves a phased rollout, starting with select cities and gradually expanding the fleet.
- 🚕 The economic and operational shift of Tesla from a car manufacturer to an AI company is becoming more apparent as software takes center stage.
- 💡 The future of Tesla's products, including FSD and Optimus, hinges on continuous advancements in AI and the ability to scale effectively.
- 🌟 The conversation highlights the rapid evolution of AI in the automotive and robotics industry, showcasing the potential for transformative changes in transportation and manufacturing.
Q & A
What significant update did Tesla release recently?
-Tesla recently released the FSD (Full Self-Driving) V12 update.
What is the significance of the V12 release for Tesla's FSD?
-The V12 release is significant because it represents a drastic rewrite of Tesla's planning architecture approach and a major leap in the capabilities of the FSD system.
What were some of the issues with the previous version of FSD?
-The previous version of FSD had issues related to planning, such as not getting in the right lane, not moving far enough over, not knowing when it was its turn, and stopping in the wrong place.
How did the guest on the podcast describe their experience with the V12 update?
-The guest described their experience with the V12 update as very positive, noting that it exceeded their expectations and that it was much more polished than they anticipated.
What is the robotaxi reveal that was mentioned in the transcript?
-The robotaxi reveal mentioned in the transcript refers to Tesla's planned announcement of its robotaxi service, which is expected to be revealed in August.
What were some of the improvements observed with the V12 update compared to the previous version?
-With the V12 update, improvements were observed in the planning stack, with old failings being addressed and not replaced by new issues. The system also seemed to drive more naturally and made better decisions in various driving scenarios.
What is the expected timeline for Tesla's robotaxi service rollout?
-While a specific timeline was not provided in the transcript, it was suggested that Tesla might start testing unsupervised robo taxis on the streets in the second half of 2025.
What are some of the challenges that Tesla might face with the rollout of the robotaxi service?
-Some challenges that Tesla might face include ensuring the safety and reliability of the robo taxis, navigating regulatory requirements, and managing the transition from a private vehicle manufacturer to a fleet operator.
What was the general sentiment towards the V12 update at the beginning of the podcast?
-The general sentiment towards the V12 update at the beginning of the podcast was cautious optimism. The hosts were excited about the potential of the update but also aware of the challenges that might arise during its initial rollout.
How does the FSD V12 handle unexpected situations compared to the previous version?
-The FSD V12 handles unexpected situations more gracefully compared to the previous version. It is designed to mimic human driving behaviors more closely, which allows it to adapt and react better to new or unforeseen scenarios.
Outlines
🚗 Introducing Tesla's FSD V12 and Optimus
The discussion begins with Dave and James catching up on recent developments, focusing on Tesla's Full Self-Driving (FSD) V12 release and the Optimus robot. Dave shares his experiences driving with FSD V12 for three weeks, highlighting its impressive capabilities and the significant improvements from V11. They also touch on the potential for the robotaxi reveal in August and the anticipation surrounding it.
🤖 Rethinking Tesla's Planning Stack
Dave and James delve into the technical aspects of Tesla's FSD V12, discussing the shift from heuristics to an end-to-end neural network approach. They explore the challenges of removing guardrails and the surprising lack of major mistakes in V12. The conversation also covers the potential methods Tesla might be using to achieve such polished results, including simulation and data curation.
🚦 Navigating Intersections and Planning
The talk moves to the intricacies of driving behavior, with Dave sharing his observations of FSD V12's handling of intersections and its ability to mimic human driving patterns. They discuss the importance of understanding the severity of different driving mistakes and the evolving nature of the system's learning process.
🌐 Global Perspectives on FSD
Dave and James consider the implications of FSD's global rollout, discussing the need for local adaptations and the potential for cultural differences in driving styles to impact the system. They also speculate on the future of Tesla's development process, including the possibility of using human drivers as data sources.
📈 Data-Driven Improvements in FSD
The conversation focuses on the role of data in refining FSD, with Dave sharing his insights on how Tesla's vast amounts of driving data contribute to the system's improvement. They discuss the potential for generalization and the challenges of addressing rare but critical scenarios.
🚗🤖 Reflecting on FSD and Optimus Developments
Dave and James recap the significant progress made in FSD and the potential impact of the upcoming robotaxi reveal. They discuss the broader implications of Tesla's advancements in autonomy and robotics, considering the future trajectory of the company and its products.
📅 Anticipating the Robotaxi Future
The discussion turns to predictions about Tesla's robotaxi service, with speculation on potential timelines and strategies for implementation. Dave and James consider the challenges of scaling up the service and the potential for Tesla to transition from a car manufacturer to a leader in autonomous transportation.
🤖🏭 Optimus: The Path to Production
Dave and James explore the potential timeline for Tesla's Optimus robot, discussing the challenges of industrializing humanoid robots and the importance of data gathering. They consider various methods for training the robots and the potential for real-world deployment.
🌟 The Future of AI and Tesla
In the final part of their conversation, Dave and James reflect on the broader implications of Tesla's AI developments, considering the potential for the company to evolve into a major player in the AI industry. They discuss the impact of open-source models and the future of AI in consumer products.
Mindmap
Keywords
💡Tesla's FSD V12 release
💡Optimus robot
💡Robotaxi reveal
💡AI and machine learning
💡Human mimicry
💡End-to-end learning
💡Perception stack
💡Heuristics
💡Autonomous driving experience
💡Neural networks
💡Strategic path
Highlights
Discussion on Tesla's FSD V12 release and its improvements
James' experiences with FSD V12 during a cross-country trip
Impressions of FSD V12's capability in rural and urban areas
Comparison of FSD V12 to V11 and the changes in planning architecture
Expectations for FSD V12 and its surprisingly polished performance
Discussion on the potential reasons behind FSD V12's success
The role of neural networks in achieving a more natural driving experience
Thoughts on how Tesla might have achieved the polish in FSD V12
The importance of end-to-end training in neural networks
Discussion on the challenges of removing heuristics from the planning stack
The potential for FSD to exceed human driving capabilities
Expectations for future improvements in FSD based on current trends
The significance of the transition from heuristics to neural networks in FSD
The potential impact of FSD V12 on driver intervention and safety
Speculations on the future of Tesla's Autopilot and FSD
Transcripts
hey it's Dave welcome today I'm joined
by James dama and we've got a whole host
of things to talk about we've got um
Tesla's FSD V12 release that just
happened this past month we've got um
Optimus to talk about um and this robot
taxy reveal in August so anyway it's
been a long time it's been like at least
a half a year was last August or
something like that so yeah yeah I
remember the last time we met we talked
about V12 cuz they did a demo mhm and um
we were quite excited about the
potential but also a little bit cautious
in terms of how it will first roll out
and how capable but um curious just what
has been your first experiences and
first impressions of you talk how long
have you been driving it for uh I got it
a few Sundays back I think I I got it
the first weekend that it really went
right so I think I've had it three weeks
or something like that maybe four three
probably and uh of course drove it out
here to Austin from Los Angeles drove it
quite a bit in Los Angeles on the way
out here so my my wife has this hobby of
like visiting supercharges we've never
been to so every cross country trip
turns it's ends up being way longer than
otherwise would be but one of the cool
things about that on the FSD checkout to
her is that we end up driving around all
the cities on the way you know because
you're driving around to the different
Chargers and stuff and so you get a
chance to see what it's like in you know
this town or that town or um different
you know highways are different we drive
a lot of rural areas so I got lots of
rural we uh we did like the whole back
Country tour coming out here through
across Texas and so feel like it was it
was a good experience for like trying to
compress a whole lot of FSD yeah and I
got to say I'm just like really
impressed like it's I was not expecting
it to be this good because it's a really
like this is not a small change to the
planner was yeah with v11 we had gotten
to a point where the perception stack
was good enough that we just weren't
seeing perception failures I mean they
just but people almost all the
complaints people had had to do with
planning not getting in the right lane
not being able to move far enough over
um not knowing when it was its turn uh
stopping in the wrong place creeping the
wrong way these are all planning
elements they're not uh you know so if
you're going to take a planning stack
that you've been working on for years
you've really invested a lot and you
like literally throwing it away like
there just not retaining any at least
that's what they tell us they got rid of
300K lines they went end to end it's
harder to actually mix heuristics into
end to end so it makes sense that they
actually got rid of almost everything
anything they have in there that's
heuristic now would be built new from
scratch for the end to end stack and yet
they managed to
outdo in what seems to me like a really
short because they weren't just
developing this they were developing the
way to develop it you know they were
having to figure out what would work
there's all of these layers of stuff
that they had to do so my you know my
expectation was that the first version
that we were going to see was going to
be like on par it would have some
improvements it would have a couple of
meaningful regressions and there would
they would be facing some challenges
with you know figuring out how to
address so because it makes sense that
they want to get it out soon and the
sooner they get it out into the fleet
the faster they learn um but the the
degree of polish on this was yeah in a
much higher than I expected and like you
know Bradford stopped by and I got a
chance to see 1221 as he was coming
through we only had about 40 minutes
together I think I it was just like the
spur of the moment thing and uh and yet
even in because he was kind enough to to
take it places that I knew well that I
had driven on 11 a lot and I think it
took me about three blocks to realize
like right away and after 45 minutes I
just knew that this is going to be
completely different and every
everything that I've experienced since
getting it and
I you know what have I got I'm I must be
at like 50 hours in the seat with it
right now a few thousand miles highly
varied stuff yeah it's super solid yeah
yeah I think um yeah I wanted to dive
into kind of how big of a jump this fsd2
is because when I drove it I was shocked
um because this is not like a is I think
V12 is a little bit of a misnomer
because this is a drastic you know
rewrite of their whole planning
architecture approach different
different um I mean on their perception
it seems like they probably kept a lot
of their neuron Nets um in terms of the
perception stack added on as well but in
their planning stack this is where they
pretty much it seemed like they're
starting from I would say scratch
completely but they're taking out all of
the guard rails all their hortic and
they're taking putting on this n10
neural approach where it's deciding
where and how to navigate right the the
perceived environment but I would have
imagined and this is kind of my
expectation also is like you you would
be better in some ways it would be more
natural Etc but then there would be some
just like weird mistakes or things that
it just doesn't get because all of the
guard rails are off theistic ones and so
you're just like it's D more dangerous
than some other ways right and that on
par though Tesla would wait until it
would be a little more safer before
releasing V12 but what we ended up
getting was we got this V12 that just
seems like really polished you know
we're not it's not easy to catch those
big mistakes in V12 and I'm kind of like
where did all these big mistakes go like
you know that was my expectation at
least and so I'm wondering like like
what was your did that catch you off
guard like just seeing the the the small
number you know of of big mistakes or
seeing how polish this V12 is um and
then I also wanted to go into like how
did Tesla do that in terms of um because
once you take off the heris sixs at
guardrails you really have to
like like be confident you need I don't
know like yeah I'm curious to hear
what's your take on how you think they
achieve this with B12 you know the the
the the Polish they have well first yeah
it
was well there's two components of like
starting out experience there's like my
sort of abstract understanding of the
system and what I sort of rationally
expected and then there's you know
there's my gut you know because I've got
I've got like 200,000 miles on various
layers of autopilot including you know
maybe I don't know 50,000 miles on FSD
so I have this muscle memory and this
you know sort of sense of the thing and
I expected that to sort of be dislocated
I mean you know going from 10 to 11 and
was also I mean they added a lot this is
not the first time that they've made
pretty substantive changes it's the
biggest change for sure right but I was
expecting it to feel a little bit weird
and uncomfortable but but sort of
intellectually I was expecting all the
old problems to go away and a new set of
problems to come in because it's a
different product
like because the perception was pretty
polished and and the things that people
were most aware of is failings of the
system were essentially baked into this
heuristic code well of course you take
theistic code away all those failings go
away too but what do you get with the
new thing right so and you know so that
did happen like all the old failings
went away like rationally right but it
was weird to sit in the SE in the seat
and you know there you know there's this
street you've driven over and over and
over again where there was this
characteristic behavior that it had
which is you know maybe not terrible but
not comfortable maybe or less ideal than
you would are slower annoying whatever
the deal and those are just gone like
all of them not just like one or two
they're just like gone all of them so
that was sort of like it was such a big
disconnect that it was kind of
disquieting the first you know week or
two I mean delightful but also
disquieting because now you're like
Uncharted Territory you know what demons
are looking here that I'm not prepared
to
you know after you drive theistic thing
for all you kind of got a sense of the
character of the failures I mean even if
you haven't seen it before you know the
kind of thing that's not going to work
and now but I didn't I didn't really
find those like I haven't really found I
haven't seen something and I was
expecting to see a couple of things that
were kind of worrisome and where I
wasn't clear to me how they were going
to get go about addressing them and I
just I really haven't right and so like
in that sense I'm really I'm more
optimistic about it than I expected to
be at this point um how do they do it
yeah okay so let me give context to that
question a bit more because I know it
could be open-ended so I would imagine
that if you go end to end with planning
that um driving is is very high stakes
you have one mistake let's say you go
into the center divider aisle or there's
a there's a concrete wall or you there's
a signpost you drive into or a treat or
something it just seems like you have
one second of mistake or even Split
Second and your car is you know it's
just catastrophic it could be and with
V1 up until v11 you had these guard
rails of like oh stay in the lane and do
this and all that stuff but with those
guard rails off like V12 could when it's
confused just make a bad move you know
and just go into some you know another
car another Lane another you know object
or something but what about it is
preventing it you know without the
guardrails is it just the data of
mimicking humans or is there something
else attached on top of that where
they're actually doing some simulation
or stuff where it's showing like what
happens when you go out of the lane into
the next Lane you know into oncoming
traffic or if you do something like is
it is are they you know pumping the the
the the neuron nest with lots of
examples of bad things also that could
happen if you know if it doesn't you
know follow a certain path like what's
your take on
that um so that question prompts a
couple of thoughts um so one
thought are okay first of all preface at
all like I don't know what the nuts and
bolts of how they are tuning the system
they've told us it's end to end right so
that basically constrains the things
that they could be doing but when you
train in a system you can you don't have
to train it end to end I mean some
training will be done endend end but you
can break it into blocks and you can
pre-train blocks in certain ways and we
know that they can use simulation we
know that they can curate the data set
um so there're you know what's the mix
of stuff that they're doing is really
hard to predict they're going to be a
bunch of you know uh learned methods for
things that work well that are going to
be really hard to predict externally
just from first principles um this whole
field it's super empirical one thing
that we keep learning about neural
networks even like the language models
we can talk about those some if you want
to cuz that's also super exciting but
the they keep surprising us right like
so you take somebody who knows the field
pretty well and you at one point and
they make predictions about what's going
to be the best way to do this and
whatnot and aside from some really basic
things I mean there's some things are
just kind of P prohibited by basic
information Theory right but when you
start getting into the Nuance of oh will
this way of tweaking the system work
better than that way or if I scale it if
I make this part bigger and that part
smaller will that be a win or a lot you
know there's so many small decisions and
the training is like that too like how
do you curate the data set like what in
particular matters what makes data good
like that's a surprisingly subtle thing
we know that good data like some
training sets get you to a good result
much faster than other training sets do
and we have theories about what makes
one good and what makes one bad and
people on some kinds of things like text
databases a lot of work has been done
trying to figure this out and we have
some ideas but at the end the day this
is super empirical and we don't really
have good theory behind it so for me to
kind of sit here not having seen what
they have going on in the back room and
guess I'm just guessing so just like
frankly like I have ideas about what
they could be
doing um but you know I would expect
them to have many clever things that
never would have occurred to me yeah
that they've discovered are important
and they may be doubling down and we we
actually don't know the fundamental
mechanism of like how they're going
about doing the mimicry like what degree
of we you know we know that the you know
they have told us that the final thing
is photons in controls out as end to end
would be right
but uh so the the final architecture but
like how you get to the result of the
behavior that you want you're going to
break the system down
like I don't know it's it's just like
there are many possibilities that are
credible picking them and they vary a
lot and picking the one that's going to
be the best like that's a hard thing to
do sitting in a chair not knowing um
they are doing it really clearly and
they're getting it to work like the
reason why I I it fascinates me on the
on what type of like um uh kind of
catastrophic scenarios or dangerous
things that there may be putting in like
it it the reason why it fascinates me is
because with driving part of the driving
intelligence is knowing that if your car
is like one foot into this Lane and it's
oncoming traffic that that's really
really bad like you know be a huge
accent versus if there's um no cars or
something then it's okay or if there's
or just it the driving intelligence just
requires an awareness of how serious
mistakes are in different situations in
some situations they're really really
bad in some situations the same driving
maneuver is not that dangerous and so it
just seems to me like there have to be
some way to train that right to teach
the the neuronist that so there's an
interesting thing about the driving
system that we have and
people okay first so the failure you're
describing is much more likely with
heuristics like heuristics you build
this logical framework a set of rules
right where um you know when heuristic
Frameworks break they break big like
they because you can get something
logically wrong and there's this gaping
hole this scenario that you didn't
imagine where the system does exactly
the opposite of what you intended
because you have some logical flaw in
the reasoning that got you to there
right so you know bugs that crash the
computer that take it out like we you
know computers generally don't fail
gracefully heuristic computers right
neural networks do tend to fail
gracefully so that's one thing right
they they they're less likely to crash
and they're more likely to give you a
slightly wrong answer or a you know to
get almost everything right and have one
thing be kind of wrong like that's a
more kind of characteristic thing so
neural networks
you know the way that they're going to
fail is going to be a little bit
different than heuristic code and
they're just by their nature they're
going to be somewhat less apt to that
kind of failure not that it's impossible
just that it's not going to be the
default automatic thing you know if you
get an if statement wrong in a piece of
code or something you you know
catastrophic failures are kind of the
norm in logical chains so um then
there's this other thing which is the
the system that we have is for is it's
Evol co-evolved with drivers you know
you uh you know you you learn you
develop reflexes you read the traffic
you read the
environment um you know when the lane
gets narrow people slow down people sort
of have a set of reflexes that adapt to
an environment to try to maximize the
safety margin they have for what they're
doing you're when you're driving down a
row of parked cars if you have space you
move over to give your safe a little
more space um you know if you're coming
up on an intersection and you can't see
what's coming you may slow down you may
move over to give yourself more space to
see what like all of these unconscious
behaviors right and the road system has
been developed over a lot of years to
like take advantage of the strengths of
people and and minimize the weaknesses
of people right I mean the way this the
amount of space that we provide on roads
and the way that we shape our
intersection sight lines that kind of
stuff the rationale for how our our
traffic controls work and all that kind
of stuff is
uh it's evolved to the strengths and
weaknesses of human beings right so
human beings are constantly trying to
within certain margins maximize their
safety margin give themselves make
themselves more comfortable that they
understand what what's going on right so
and now we have a system that's
mimicking people right so like there are
funny things that the that the that the
car will do that that just really is
kind of underscore this like you know
you're in a line of cars and that they
suddenly slow down and you have a truck
in front of you so one like one of the
most natural things is people will pull
over a little to if they can't see to
see what's happening up there to help
them prepare for what might be happening
to give them more situational awareness
well you see the cars do this sometimes
the funny thing about the car is the car
the the car it like it's camera is in
the center so moving a little to the
left doesn't let the car see around the
car ahead of it right it still can't see
but it still mimics that action so
similarly coming up to an intersection
slowing down moving over you know
preparing yourself so essentially
there's this interesting characteristic
that you're going to get out of that is
it is it the is that the planning system
is going to mimic the margin you know
that do the little Preparatory things
that give you a little more margin a
little more situational awareness and
help you prepare give you a little more
time to react in case something happens
it's mimicking all those things now so
uh instead of the her istics having to
kind of be perfect instead what the
system is doing is it's learning to
mimic PE you know drivers who already
have all these reflexes and and and
behaviors in a really complicated
contextual environment so it's not like
we're not talking about four or five
behaviors you know we're talking about
four or five thousand behaviors the kind
of things that people were as drivers
were not even aware that we're doing
them and the car is mimicking that right
in the thing and so so so they're going
to fail more gracefully and they're
mimicking drivers who are you know who
are cautious in situations where they
need to be cautious and they're you know
they're they're making small adjustments
to give themselves more margin all the
time and I think we may have under
appreciated the degree to which you know
human drivers with a lot of experience
have
reflexively you know developed a lot of
behaviors that are actually because
we're talking about Good drivers here
right uh they've they've unconsciously
developed a lot of habits that actually
have a an appreciable impact on their
safety and and the system is now getting
those for free kind of because it's
mimicking drivers right even all the
little Nuance things that we that kind
of don't make sense like I said like
pulling over to see what's ahead of the
car uh ahead of you or we see the like
the the behavior where that the very
Charming Behavior where you know it
doesn't block the Box you come to an
intersection and if it's not clear that
it can get across it stops right like
nobody had to program that and if you
look at intersections like when to do
that and when to not do that that's kind
of subtle right like is the car ahead of
you going to move forward Enough by the
time you cross intersection or is it not
and if you look at the flow of traffic
like as a human you're like better than
even odds there will be space when I
cross or no I should definitely stop
here because I don't want to be caught
in the intersection the cars mimic all
that yeah even in really complicated
context I mean I would say I mean
mimicking it it seems like it goes even
a little beyond the mimicking at times I
think this is like the unch territory
which V12 surprises me is it mimics with
some level of understanding sometimes
like why it because for example you're
going you don't know whether to to go
into the intersection or not or let's
say you're you're turning into pedest
left turn into and pedestrians are here
every situation is a little bit
different and so just because in your
data you have a bunch of examples it's
like there it might not be the perfect
like you might not be able to mimic
perfectly because it's a new situation
so you've got to infer kind of in this
new situation what should I do and
that's where I think it's not just
mimicry it and it could be just mimicry
right now but the the the big I guess
jump in in ability is is is UN is it's
kind of like llms you know like they
they can understand to a certain extent
what you're asking for in a new
situation or a new you know dialogue I
think the word you're looking for is
General
yeah yeah yeah maybe generalize like
taking that the specific mimicry
situations that the data provides and
generalizing those but there's a certain
level in order to generalize that you do
need um capability Beyond just mimicry
right some level of of maybe application
or so mimicry I mean we talk about
mimicry mimicry is the training goal
right do what a human would do in this
situation that's why we call it mimicry
right but
the system it doesn't have the capacity
to record every single possibility right
and so it's frequently going to see a
situation that's kind of a combination
of situations it's seen before it's not
a duplicate of any of them and it h and
you have to kind of figure out how to
combine what you learned in these other
situations that were similar but come up
with something that's different and yet
somehow it follows the same rules so a
way you could think about it is that the
using the block the Box Thing depending
on how many lanes of traffic there are
and how aggressive the drivers are and
what the weather is like what the cross
traffic is like you know just all of
these variables you you you as a human
you come up to the intersection you have
to make the decision whether you're
going to cross and maybe get stuck or
whether you're going to you're going to
pause and wait for the other car to move
up you know I saw one i' I've seen one
where where I had the the block box and
you could see the light at the end of
the row of cars right and like this is
the thing humans do when this light
turns red you know you have plenty of
time to cross because it's not going to
turn GRE you're not going to get stuck
and you see the next light up there turn
green well even if you get stick in the
Box it doesn't matter I was been in that
situation twice now and the car moved
into the intersection even though it
would block it because it's confident
that the row of cars well who coded
nobody coded that right there's now as a
human I'm describing this thing well
here's a rule I just made up if this
light has just turned red you know there
will be no cross traffic and the light
ahead turns green while the car is ahead
they're definitely going to move forward
almost certainly right unless there's a
broken down car or something like that
and so you see humans do this they move
up because they know they're going to be
able to and they want to all they want
to take they want to reserve that space
behind that car for themselves you know
to get their priority for crossing the
intersection so they move forward I see
the car mimic this Behavior right only
where it's really
appropriate so in a sense what I when I
described that to you what I did was I
looked at the situation and I figured
out what the rules were oh this light
changed that light changed now I have
time right yeah but when I've done that
in the past I didn't think about the
rules consciously I you know I'm not
checking off this list of things I see
the conditions where it's safe for me to
move forward I'm unlikely to block
anyone and I do it right so a way that
you can think about what the system is
doing is it's we're training it to mimic
it but it has to compress that somehow
to save that into a set of rules that is
more General so what the you can think
of what the system is trying to do is
trying to figure out what the rules are
like I've seen these 50 block the Box
situations what rules say when it's good
to go and when it's not good to go so if
it can figure out what those rules are
like if it's it's essentially getting a
and you know understanding is a loaded
word so I don't like to use
understanding right but it's deriving a
representation of the rule set if you
will that humans cross which might you
know when we write code we want to
minimize the rules keep the code simple
so we don't have weird bugs and that
kind of stuff but neural networks if
it's if the r if the simple version of
the rules is 300 rules that's fine like
300 rules is no problem for them so if
humans have unconsciously 300 sets of
rules that we use to decide when we go
across and it can come to figure out
what those are well that lets it
generalize it can now take the same
principles it's extracting the
principles unconsciously not rationally
just reflexively in the same way people
do it's extracting the principles that
humans are using to make that decision
and it's applying those to its own
actions and so that's where you
and we it manifest some you know some
cute behaviors that are irrational for
the car right perhaps but it also
captures I mean the fact that for I mean
you get you know as a Pim had said that
you you get the the puddle of voiding
for free right you got the u-turns for
free like when is it to say the U-turn
or not that's hard to write you just you
get that for free but you also get the
oh this guy wants to turn left into the
parking lot so I'm going to pause back
here and let him go or somebody behind
me wants to pass me I'm going to move up
a couple of feet so they can get in or
move over you see the cars doing all of
this stuff right like they're
not you know the autopilot team they're
not picking and choosing the behaviors
that they want it's it's I mean it seems
clear to me anyway looking at this that
they're grasping the whole spectrum of
all the behaviors that people do the
polite things the impolite things where
people are irrational I mean one
thing that I
do like one of the things I liked before
because it it it does mimic some things
that I would prefer it doesn't mimic but
they're extremely human behaviors and
that is like when you're on the highway
humans tend to follow other humans other
cars too closely in certain situations
where the traffic is kind of dense and
whatnot and I've been just using the
auto speed letting the car pick its own
spacing and stuff and I notice that you
know previously there was a hero stick
this many car lengths and no less and
you know maybe temporarily for breaking
and stuff it might go soer but was
really good at maintaining a really
comfortable distance and now I notice
it's kind of it's driving more like
people and I kind of preferred when it
was keeping more space like I liked that
the car's ability to like maintain more
have a bigger and you know you don't
pick up rocks from trucks and stuff but
it's now F it's it's it's on I'm finding
it's mimicking human following Behavior
which I personally find less than ideal
but that's part of the whole like that's
definitely something that if you were
picking and choosing you wouldn't have
picked to add because it's not a win
like it's an irrational behavior that
humans engage in that can lead to
accidents that reduces your safety
margin but the car is going to mimic
that too because you know they're taking
the good with the bad in order to get
everything including the stuff that they
don't necessarily know is in there I was
suggesting there are all these
unconscious rules that we follow well
they're unconscious to the autopilot
team too like they don't know to go look
for that so they're and but the net net
is it's you know the reality is they've
got this thing it's out there and it's
just working incredibly well yeah yeah I
mean it's yeah it's interesting I guess
on the topic of generalizing so um I
think that's probably one of the most I
think promising aspects of V12 is that
the behaviors that are it's picking up
um some of it can be unexpected because
let's say you've got you know 100 you
know videos on on um on whether or not
to go in and out of an intersection or
something at a at a yellow light or
something or a green light even if it's
blocked but then um so the neuron Nets
are analyzing and training training data
like through billions of parameters and
analyzing this these these videos
getting what what it can out of it I
also wonder I guess it goes back to this
whole thing is are they adding more
types of data where it's like are they
adding onto those video clips or
providing different stuff of if this car
actually does this then you know there's
a crash or does this there's a crash cu
it seems like if it's if they're only
providing 100 say video clips of it
doing well then the signal for the
negative for the dangerous situation
isn't as high as if you give it directly
like so that's useful in reinforcement
learning where having negative examples
is really useful because you're trying
to figure out what the score is and you
have it good and bad um in the case of
human mimicking right the score is just
how close did you get to what like the
way you rate the how the neural network
is doing and training is you show it a
clip it hasn't seen before and you ask
it what do you do here and you rate it
just by how close it was to what a human
did so you take a human recorded example
that the system isn't trained on has
never seen before and and when I test it
to decide these other Clips are they
helping are they hurting I give it one
it's never seen before and and wait and
and good and bad is just how close are
you to the human it's not did you crash
it's not there no in reinforcement
learning you do that you you know you do
or contrastive learning you know there
are other things where you do that but
the simple mimicking at least the way
that it's done in robotics
overwhelmingly right is we just we have
a signal from from a Target that we want
you to get close to and and your score
is just how close you are to that so the
degree to which it mimics a recording of
a never-before seen good driver Behavior
that's its score so you don't need the
crashes so do you think that they're
only doing that type of mimic training
versus are they you don't think they're
adding on different types of contrastive
or let's say reinforcement learning or
whatever long term reinforcement
learning is going to be really useful um
like you know I mentioned there are
these various technique there are
various ways that I can you know when
fundamentally neural networks you know
the way they train them is you give them
an example and then they say what they
would do in this situation and then you
give them a score and based on the score
you you adjust all the weights and you
just do that over and over again and the
weights eventually get really good at
giving you the answer that you're
looking for okay how do I pose the
problem um in reinforcement learning
what you do the the problem is you do
you play all these steps and then you
get a score for the game so this is how
like deepmind did with you tari games
and that kind of stuff you do a whole
bunch of actions and this is the
challenge in reinforcement learning is
it's hard to know which you know if you
if you have to do a 100 things to get a
point well how do you know which of the
hundred things you did was important
which wasn't like that's a big Challenge
and so reinforcement learning does all
that but because of this challenge
reinforcement learning tends to be very
sample inefficient we say it you need
lots and lots and lots of games to play
before in order to learn a certain
amount of stuff if on the other hand you
were trying to train Atari right and you
and your feedback signal was have the
paddle go exactly where the expert human
does right then that's more sample
efficient it learns faster so remember
we've talked about the alphago example
before right so when they first started
training alphao the first step that they
did was they had it mimic humans they
took 600,000 expert human games and the
first stage of training the first
version of alpha go was they just
trained it via human mimicry do what the
human did now that got them a certain
distance right that got them to because
they had 600,000 games which were decent
but you know decently good human players
but they were like amateurs or whatever
how do you get to the next level well in
the case of a game like go or chess or
whatnot a thing you can do is you can
start doing reinforcement learning now
reinforcement learning in those kind of
settings in in chess you've got you know
16 30 50 moves that choices at any given
point you have and maybe only 10 of them
are good choices so you don't you know
the the tree of possibilities doesn't
expand that quickly right so
uh so essentially you can get the
network that's trying to learn which of
13 possibilities to converge much faster
than if the choice is much bigger and in
the world you know we have these
continuous spaces where where like you
can turn the steering wheel to 45° 22°
13.45 7° you know the space of
possibilities is is really large and so
because so this is a real challenge with
reinforcement learning so people have
tried to do reinforcement learning with
cars in games like you know car driving
video games and that kind of stuff and
we know it works but we also know it's
very sample inefficient okay so me
looking right now at where Tesla is I
would guess that they're doing human
mimicry and they might be doing a little
bit of reinforcement learning training
on top of that you know maybe there's
something you want the system to do and
it's not quite getting there with the
mimicry stopping at stop signs you know
um and so you you can layer on a little
bit of reinforcement learning on top of
that to just tweak the behavior of the
system so incidentally this is what this
is what chat GPT did originally remember
there with chat gbt there was the the
basic training then there's instruct
training where you you tell it don't
just predict the next token pretend
you're in a dialogue right and then
there's one more step after that that
they do with chat GPT which was the
reinforcement learning from Human
feedback right which is where you do at
that after you get to that point now you
do a little reinforcement learning and
you train it don't just pretend you're
in a dialogue with me but you're in a
dialogue with me and you want to please
me these are the answers that humans
prefer so that last one is the one that
makes it polite and gives you alignment
and all that that other stuff now it's a
tiny fraction of the overall training
the overwhelming bulk of the training is
a pre-training just predict the next
token and then there's a big chunk of
the instruct okay so you can do a
similar thing with self-driving and I
would sort of expect that that's how it
would evolve that you know there's a ton
of pre-training for the uh perception
Network which is just you know they
already have all this labeled data and
they can they've got an autol labeler so
they can take these recordings they can
generate you know maps of where all the
street signs are they can ask the
perception system tell me where the sign
is and whatnot so that's a ton of
training on supervised data which is
very sample efficient that's the most
sample efficient kind then they go to
maybe a more General thing where they're
mimicking humans that's also supervised
but it's in a broader domain but it's
still more sample efficient much more
sample efficient than reinforcement
learning so then at the tail end you add
you know it's this layer cake you build
the foundational capabilities then you
do some refinement and add some
additional capabilities and then maybe
you fine-tune with yet another kind of
training at the end of it so if they're
using reinforcement learning right now
because of the sample efficiency issue I
would expect it to be that cherry on top
kind of thing right at the end the last
little bit where there's one or two
things that the mimicking isn't getting
you or it's mimicking a behavior you
don't want it to and now you on now you
come up with a new game for it to play
where you've got a game and it has to
get a score and now you're going to do
reinforcement so you could totally do
that and eventually they will because if
you really want to get deeply superhuman
that's how you did it that you know
that's what we learned one of the
examples from go was you know it got to
play when it first when it was when they
were first playing Fon way you know who
was the European Champion like it could
kind of get to his level with that
mimicry and maybe Monte Carlo search on
top of that which is basically you know
not just doing doing the first thing the
neural network has but exploring a few
possibilities just heris right that got
him there and they could beat fine way
but they're not going to beat Lisa do
that way there aren't enough example
games and you know for it to train on it
has to play against itself with this
reinforcement and then then the sky the
limit how good it is possible to be
becomes the limit of how good the system
can be and then they can become truly
superhuman So eventually we'll see you
know self-driving systems they will
they'll do that you know as as we get
more computers more computer capacity as
we learn how to do reinforcement
learning in this domain it will come to
that and so you know longterm I think
that's very likely some I mean there are
things that do the same thing as
enforcement learning they're a little
bit different but one of these
techniques so it can self-play so that
it can it can learn to be better than
humans can ever learn to be um like
that'll become part of the for but we're
not there yet right I mean there's still
the lwh hanging fruit of being as good
as a really good human driver yeah
because if FSD was was equivalent to a
really good human driver but it never
got tired it never got distracted it
could see in all directions at the same
time that's a great driver like that's
superhuman by itself it it's decision
making doesn't necessarily have to be
superum but the combination of its
perception and its def
fatigability right inability all right
it never gets tired uh the combination
of those things on top of good human
decision making like I kind of feel like
as a near-term goal that's a great goal
and that will get us tremendous utility
and you don't necessarily need more than
human mimicking in order to do that okay
so on human mimickry so um when Tesla's
training um and feeding their neurons
that's uh all this you know video of
good drivers
driving how is the training working so
for example is it you're in a situation
and it's say um is it telling the neural
network to predict what the human will
do next and then show what the human
does next and it it corrects it its
weight is it s is it something like that
basically auto training itself off of
all of the videos right yes okay I would
guess they're
probably so you take the human drive and
you break it down into some variables
right like positioning timing decisions
for Lane uh stuff and whatnot to create
kind of a scoring system for how close
are you to what the human did is it you
know do we just look at all the controls
and we take you know least uh uh mean
squares error of the car versus that you
could do that maybe that works great
maybe uh maybe you go take a step
further back and you say what was the
line the human took through the traffic
and you know what's the distance at each
point you are off that line maybe that's
the score or the speed um there might be
other elements of the score like you
know how quickly did you respond when
the light changed when The Pedestrian
moved I mean you could layer other
things on top of it you would you would
start with the simplest thing you know
this uh mean squares error right and
then if that didn't work or if you could
at layer other things on to it to make
the scoring because having a good
scoring system is an important part and
this is all comes down to sample
efficiency too like you know does my
super computer run for a week to get me
a good result does it run for a month
does it run for a year that's sample
efficiency like how fast do I get to the
result I want the system itself will
constrain how good it can get but a good
scoring system can get you there faster
it's economics and so they'll definitely
there will be a lot of tricks in that
scoring function that they have we call
it the loss function mhm and
uh you know so it would be really like
as a practitioner I would be really
curious to know like what they're doing
but they do have one they've got they've
come up with a scoring system and it's
almost certain that you know essentially
they're taking what the human did they
have this sort sort of you know ideal
point you know they have an ideal score
that you could get any and the and the
system score is just like how close are
you to like what what our uh expert
human did in this situation yeah I mean
what's exciting about kind of of being
able to train like that is it reminds me
of you know the whole Transformer
Transformer model with chat gbt it's
like you could give it so much data and
it just you
know takes all that data and and by
predicting the next token and then and
then rearrange its own weights it could
just get better and better and it's just
it's so scalable in a sense you just
feed it more data um more parameters it
just gets better and better um because
the the training is just it's just such
a um such an efficient you know usage of
it a really interesting metaphor is you
know if if a text model is learning to
predict the next token right exactly
okay it's well these tokens they're all
written by humans right like all this
stuff before there were language models
like all the text was written by human
beings right we didn't have automated
systems that generated any meaningful
amount of the Conta so in a sense it's
just predicting what the human the ne
what was the next thing the human put
it's a kind of human mimikry right
exactly yeah but when we look at if you
look at what like chat GPT can do
relative to what a human can do well
there are things it can't do that a
human can do still there's forms of
reasoning and whatnot that it still pour
out but there are a lot of ways it's
nutly superhuman like its ability to
remember stuff is just like it's vastly
superhuman like you can talk to it about
any of 10,000 Topics in a hundred
different languages you know it's like
deeply superhuman in certain respects
already and so you could expect the same
thing from the mimicking like if they're
learning protect predict the next
steering wheel movement predict the next
brake pedal that like in a sense you you
get a similar kind of thing it's not
necessarily constrained to just what a
human could do because its capacities
are different it's going to learn it a
different way like it's not a human
being like human one of the things about
human beings is we have these really
terrible working memories right which is
one of the reasons that our that our
like thought process is broken into
these two layers this unconscious thing
and the conscious thing that because
consciously we can only keep track of
like you know a few things at one time
well you know um FSD doesn't have that
problem like when a human being comes to
an intersection one of the challenges
that you have is you know there's three
pedestrians and two cars crossing and
you're turning your head to look at them
you're paying attention to a couple well
FSD is simultaneously looking at 100
pedestrians all the street signs all the
cars in all directions simultaneously
like it doesn't have attention the same
way we do m so so even given the same
set of you know ideal uh the same Target
to get to because it's getting there in
a different way there's lots of
potential for many of its behaviors to
be greatly superhuman even just in the
planning sense you know I mean the the
human being doesn't end up being the
limit in the same way that the human
being isn't the limit like for chat GPT
like the upper bound of how many
languages Chad gbd can learn is much
higher than the upper bound of what the
number of languages a human can be
fluent in right and similarly you know
like what can you tell me about you know
the Wikipedia page on Winston Churchill
like how many humans are going to know
that right and Wikipedia does try it
it'll it can tell you yeah yeah that's
interesting because yeah the It's
ability to retain you know like so much
more information I mean for example
Chacha and also if you apply that to FSD
through the training like if like if a
human was to be trained like as a
Transformer model for like LM you know
we wouldn't retain of you know it would
be like I mean it would just be like
it's like for example the the amount of
data we get from I guess you know just
looking at video clips ourselves it's
it's limited we're just looking at one
aspect maybe like how the person's
turning a little bit about the
environment but um a neuronet is picking
up a lot more subtle things that maybe
we're not completely conscious or aware
of and retaining that as well um so I
mean I I think two things one is
it just seems so scalable you just feed
it a thousand more times data you know
across a variety of of scenarios and it
just gets that much better you know it's
so it's the potential is just crazy
right um the second thing is is this
kind of crossover of abilities where it
does stuff that maybe you didn't expect
it to do because it's learning from
other scenarios and other situations and
kind of generalizing in new new
scenarios right and so it's kind of like
these ENT behaviors or abilities that
you weren't planning or you didn't train
for originally and I think as you feed
it more and more data um we're probably
going to see more and more of that kind
of people will feel like it's superum in
some ways it's just better driver than
me um and that is going to come out more
and more right as you know the data
increases yeah well we're going to see a
lot of those I mean I
already have lots of EX have I mean I've
only been trying for a few I mean I got
this on v11 sometimes but I'm getting a
lot more in V12 where you come to an
intersection and then it gets a behav
well I like I I told somebody the other
day that um that on v11 early v11 for
sure if I
intervened you know I want to say like
80% of the time the intervention was the
right thing to do right and every once
in a while you'd intervene then you
realize that the car was right you know
oh no I needed to take that turn instead
of this or I intervene because I thought
it was slowing for pointlessly for the
stop sign and I didn't see The
Pedestrian or I didn't see the speed
bump you know or whatever the deal was I
want to say on V12 I'm getting much more
into the Zone where it's like 8020 the
other way you know like 80% of the time
I intervene it was my mistake the car
saw something it was responding to
something that I should have that
ideally I would have seen I would have
responded to but but I didn't right and
you know so it's exposing more of my
fail when we disagree it's often
exposing my failings more than the
systems failings you know as that goes
and I think that's you know we're on the
trajectory we on right now now we could
very quickly be getting into a world
where you know the odds are if you like
you should still intervene you know it's
because the system is not perfect but
but you know 99% of the time you
intervene the car was right and it was
you and it's you that's wrong and you
know so that's that begs a question of
like at what point do we not let the
drive right because like is it 99 or
99.9 like how how much more right does
the car need to be and of course that's
going to depend on the waiting of Errors
you know like if if the 99er and the one
is Extreme you know but I think you know
I think there's a good chance we're
going to be there this year yeah at the
current rate of progress and that's
going to be really exciting I think what
what can trick people is you think V12
is like the next iteration of V1 right
so it got you know from v11 to V12
you're like oh big jump right and so
you're thinking okay maybe in another
year we'll have another big jump you
know v13 or something it'll take another
year and then you project that but I
think the tricky part is V12 was largely
done under the cover as this you know
stealth project um not released to the
public or really shown much and it's
really been like probably you know
supposedly maybe December of what 202
it's building on a lot of infrastructure
that was built for those other projects
too but yeah so it's a difficult
comparison to make but it's not unfair
to say yeah this is a clean sheet for
the the planning part and did
if you look at the trajectory of how
fast let's say the planning and is
improving and and it's probably you
could probably map it out with the
amount of data you're you're putting
into it and map out the abilities and
Tesla has probably the ability to see
into the next 12 months in terms of how
much compute that have they have how
much data they could feed it and what
type of abilities they're expecting from
it you think and I think that would
surprise a lot of people one one thing
we don't know what abilities like
there's some things that are clearly
have been left like the parking lots
have been left out at this point right
the actually smart summon you know we're
waiting on that
um why are those held back are they held
back because they had this part working
well and it's 95% of what people use it
for and we're going to push it out are
they holding it back because there's
something tricky about it and they want
to get it right and so does that maybe
indicate that there are some challenges
there we don't know until it comes out
uh parking lots are really different
than driving on surface streets and so
it wouldn't be surprising if there's
some novel things problems that occur in
parking lots at high rates I mean there
are benefits in parking lots you move
really slow it doesn't matter if you
stop you know it's not like driving on a
Surface Street so I believe you know
ultimately they're tractable and whatnot
but you know we don't know that it's
it's feature incomplete I would say at
this point and so when when it's feature
complete then it'll be easier to predict
what the scaling do do you have you
heard the expression the bitter lesson
no no okay so it's this white paper was
written by a machine learning uh
researcher named Richard Sutton it's
kind of famous inside the field right
Richard su he basically wrote this thing
it was an observation about machine
learning over the decades right and
especially recently and it basically
says that what the field has learned
over and over again is that doing simple
things that scale that maybe don't work
great today but which will get better if
you scale them up always wins over doing
exotic things that don't scale and the
temptation as a researcher is always to
do is to get the best research you to
get the best performance you can at
whatever scale you're working at in your
lab or whatnot even as a small company
but Sutton basically observed that that
betting on techniques that scale like
maybe doesn't work great but it
predictably improves as you scale up it
all they always win they just always
always always win and you know it's he
called it the bitter lesson because you
know researchers keep learning that you
build this beautiful thing but because
it doesn't scale it falls to the Wayside
nobody ever uses it and the simple thing
that everybody's known since like know
1920 or whatever that just scales well
is what just people keep doubling down
on so yeah this is what models are
teaching us today right and a thing
that's the way that this relates back to
FSD is that heuristics aren't scalable
you need humans to do it the more
heuristics you have like if you have
300,000 lines of heuristics and they
have a certain number of bugs when you
get to 600,000 you don't have twice as
many bugs you have like four times as
many bugs because the interactions get
more complicated right so so there's
like poor scaling like heuristics don't
scale heuristics written by people don't
scale but if you if I just take the same
model and I give it more video and it
gets better now that scales I just need
more video and I need more compute time
and it gets better so the bitter lesson
would tell us that V12 is way better
fundamental approach to solving this
problem than v11 was with its heuristic
planner and I think if you go all the
way back you know uh Andre karpathy was
telling us in his earliest talks about
this that he foresaw the soft what he
was calling software 2 the neural
network just gradually taking over and I
think that in you know that's largely
inspired by the same thing the neural
networks are going to take over because
as you get scale they just become the
right way to do everything right and
eventually there's nothing left for the
heris stics yeah yeah I was thinking
about that karpathy quote and I think
you know the the intention was it for
for the at least the planning stack to
to be more in like gradual you know 2.0
to eat away and I think this was V12 the
endon end approach a bit more drastic
than maybe what I originally you know uh
intended but it's just to me it makes it
definitely makes sense and if they can
get it working which they have it's
clearly I think going to be the the well
there's another way to tell this story
too well like I've people have asked me
a few times and I think the right way to
think about this is that Tesla didn't
suddenly stumble onto the idea of doing
end to end end to end is obvious right
sure like if you can make end to end
work the problem is it just doesn't work
in really complex domains or or rather
it doesn't not work at all you have to
get to a certain scale before it starts
working right so I think the more
realistic way of thinking about Tesla's
relationship with end to end is that
they had they were trying it it didn't
work they tried it they didn't work you
know they would you know so you know it
may be that the reason that v11 got to
300,000 lines right is they expected end
to end to start working a year ago two
years ago they didn't think they were
ever going to get to 300,000 lines but
it took long
to get the neural network to do the
planning part yeah so essentially this
is like the dam breaking you know when
they finally find the technique that
scales that they can do that kind of
stuff the Dam breaks quickly because it
it quickly overwhelms the downsides to
having 300,000 lines of heuristics that
are guiding your planning yeah I mean
did you see that uh tweet by aoke like
something about the beginning of the end
or something do you think it's related
to FS at all
it's complet spec speculative but I I
think it is but yeah I mean what does
the comment on that's not ever right
it's
like it's it's mysterious but you know
the beginning of the end of uh of uh
people driving cars is like it's kind of
the way I look yeah I kind of wonder if
like with the internal metrics and you
know things that Tesla internally is
tracking with V12 and you know they're
they're on their next version to you
know v124 whatever and uh they're just
seeing the improvements and then and
they they know what's coming down the
line and how much compute and dat
everything going forward that they just
me they just be they just must be really
excited right now I think just to see
the level of you know of improvement
especially with um 12.3 it was still the
it was a 2023 build I mean you could
tell from the firmware number right mhm
and generally what we saw through uh
through v11 right was that the things
that were getting in the customers hands
were 3 four five sometimes six months
old right so Tesla's already looking at
the one we're going to get in six months
so I mean they may you know why does it
take them six months well they do all
this testing and validation there's
tweaking there's all these waves of
rolling it out to be super safe and
whatnot so the pipe is deep between when
they first but the but you they're going
to know the potential yeah you know when
you know the first couple of weeks after
they do those initial builds so you know
they already mostly know what we're
going to have in six months and so uh
they don't really have to guess right we
just you know it takes six months for it
to get through the safety pipe and
everything and get to us yeah um so with
v11 I remember very uh half fondly half
not fly when you're at like some like uh
intersection or something you're stopped
or moving slowly you get this like you
know jerky uh steering wheel thing it's
going left going straight going left
going straight and when I think about
that I'm like that's going to be
something I think all prev12 beta
testers have will be having their joint
experience you know this like jerky
stere have you seen the the V so V12 has
this thing where occasionally you'll be
stopped an intersection and it starts
you're totally stopped not moving slowly
you're stopped you're behind another car
something like and it just starts tur
yeah it does that yeah yeah I thought it
was just me I guess it it does a little
bit no I've seen it two or three times
the first couple of times I saw it I'm
like what are you doing you know and
it's just slowly turning the steering
wheel right I'm like this will be
interesting you know the light changes
it goes and it like whips back straight
and
D it's like it's Bard or something in
playing with
this that's funny um but okay so from
moving from V12 to V or v11
V12 like v11 it just I interpreted the
the steering wheel thing at the
intersection it's like it's debating
between two options right it's like oh
60% this way 40% but then it changes to
60% this way and then you know goes back
and forth like literally as it changes
percentage of of what it should do it's
it's changing the the steering wheel but
why in V12 we don't see that behavior
you know why is it just confidently just
going in One Direction without
human uh
okay when you have her istics you come
to an intersection your options are you
got a few options straight right left
right go don't go they're
binary so the neural
network that the output from the neural
network uh it it there's you know you're
at an intersection and you can go right
you can go straight or you can turn
right right there is no 45 degree option
right okay so the neural Network it's a
in this case it's functioning as a
classifier you choose this or choose
that but neural networks to work they
have to be continuous so there has to
exist in the system a very low
probability option between the two right
this is you know you have a a
sigmoid right the important parts of the
zero and the one but it has to be
continuous because if it's not
continuous you can't it's not
differentiable and you can't back
propagate so this is a fundamental thing
neural networks have to have has to be
okay so the system has a set of criteria
where it's going to go forward and it
has a set of criteria where it's going
to go right and you're trying you know
and you minimize you know this this is a
this is there's a certain probability
for this a certain probability for this
and they add almost one and there's a
tiny little bit of remaining probability
in the stuff in between and it's
intended to just connect the two states
so the neural network so it's
differentiable right okay this is
actually kind of a weakness in a system
where you have two states right because
imagine that you get to a set of
criteria that in you know every once in
a while you're going to get to a
situation where the system is balanced
right on that 45 point right and as the
Shadows shift and the cars move around
the contextual cues just shift a little
bit you know the the network is going to
it's going to that because that's a
choice and this is a choice and the the
system before it was built so the
steering wheel it reflected the choice
that was upcoming for the intersection
right so something is flickering back
and forth and yeah as you say it it's
it's it's oscillating there a very tiny
little oscillation but you have to have
that OS you have to have this huge
disparity between going right and going
left because going 45 is never an option
like you have to make that super super
small so if you're right on the boundary
it'll hop back and forth between two
options that to a human being seem very
disperate right the thing is if you're
mimicking a human
being you no longer have you know your
goal is to just get as close to the
human being as you have you don't have
this classifier thing where you have
these AB options so the system is not
going to end up in states where it's
making like it has the option like a
human being comes to the intersection if
they're going straight their wheel might
be here might be here might be here
right that one it might be here might be
here they're they're they're fairly
Broad and continuous it's not perfectly
straight or here with like a no man's
land in between like humans will come to
an intersection they can turn the wheel
45 degrees let it sit there and then
when the light changes turn it straight
and keep going that's not
that's not a fail for the network it's
an option so it never gets in these
situations where it's oscillating
between two states that the design of
the neural network has to keep highly
discreet for safety sake right because
it's just mimicking a human being I
don't know if I'm explaining that very
well but it it is naturally going to
fall out of the fact that that they have
a Target that they're tracking and and
the goal is to be close not you don't
have to be right on being being pretty
close is good enough would you say
because say with with FSD intend the
neuron Nets are because they're
mimicking they just have so many points
to mimic along the path and that it's
just like whereas v11 it's deciding
between left and right you or I say
straight and right it's oscillating and
these are two big decisions to make and
once you're on them it just it's going
that certain path so it's that's the big
decision versus put it this way right
okay you're writing digits down M
there's a one a two a three there's no
nothing part way between the one and the
two like it should either be a one or a
two there's no in between option that's
okay um but as a human you can have a
sloppy one or a two you know I mean if
you're if what you're doing is mimicking
the human the target the success Target
is Broad it's not precisely one or
precisely two with a No Man's Land
there's a whole bunch of different ways
you could write a one a whole bunch of
ways you could write a two there's not
really a space in between but but the
network has The leeway to have but
slightly different ones and still be
right whereas you know in the classifier
way you don't have that you've got these
a very small number of extremely
distinct decision points and so if
you're on the boundary between them
you're going to see oscillation
interesting um all right so um moving
forward to
robotaxi August 8 reveal what are your
expectations on um what Tesla expect
like why do you think they're revealing
it now you know like yeah any any
thought or any ideas on this it seemed
kind of forced after that reuter's
article maybe that was a coincidence I
don't know um the you know I've seen a
couple of theories uh my guess is that
that the a that around August that rough
time
frame there is a good time for them to
be introducing this vehic so there's
kind of there's the software angle of
interpreting it there's a hardware angle
like you know it's about time for them
to get the hardware out why would they
need to get the hardware out why
wouldn't wait for reveal like they did
with like the y or the three where they
waited until they were ready to start
taking I mean the three it was early but
with the Y they didn't want to Osborne
the three they waited and they played it
down until they got there and up until
now it seems like you know with the
compact car that they'd been doing a
similar kind of thing so so as not the
Osborne the three or the Y
presumably um if they introduce it in
August they're they've either greatly
accelerated the timeline or they're
doing an introduction well ahead of the
actual release of the vehicle which kind
of makes sense for robo taxi because
people aren't expecting like nobody's
not going to buy a model 3 because
they're waiting for the robo taxi right
I at least that's unlikely to be a thing
whereas they might wait to buy a model 3
so maybe it's less of an issue and maybe
they want to get prototypes out on the
road to start testing and Gathering data
like that's a theory I've seen seems
like not bad so that's one the other
possibility is that um they think the
software is getting really close and
they want to demo the soft Ware on a
platform to start sort of preparing the
world and Regulators for the fact that
this is a real thing it's really going
to happen and here's our status I mean
that's obviously it's good for the
company gathers
attention um it might get investors to
take it more realistically it might get
Regulators to start taking it more
realistically like this isn't ping this
guy and this isn't us just dreaming and
so don't put us at the bottom of your
stack of work like put it at the top
because this is we really need to start
working on the issue of like how what do
you are you going to require before you
allow us to operate these these things
so like those all kind of make sense
yeah yeah I wonder if the robotx would
be just Tesla owned right for certain
urban city environments in the beginning
at least um I don't see like why would
they sell it to people initially when
they have a lot of capacity needs to
fill this vacuum of ride healing because
the discrepancy of how much phys like
human ride healing costs and robot taxy
will causes such a big gap like Tesla
could easily use you know the first few
years of what production maybe 3 million
Vehicles they could it it's a really
good question and you know this is this
is something that it's been debated a
long time I have a 10e standing bet with
another guy about whether Tesla will
stop making selling cars to private
parties when the when they start making
Robo taxis uh you know you can see it
going like I've tried to work this a
couple of ways I can see advantages
either I mean the robo taxi where holy
owned Fleet thing it's upside is a
simple model like predicting and
understanding it or kind of
straightforward right I don't know like
I would argue it's not the best model to
like to plan kind of long term I Al feel
like when I think about the whole sweep
of this thing like I've said before that
you know I feel like the robot tax is
going to go through this period of time
where a relatively small number of robot
taxis really profitable but as the fleet
continues to grow and we and it
continues to take more miles it becomes
commoditized now the degree to which it
becomes commoditized like ultimately
it's still a profitable business it's
much bigger business so the total profit
being generated is bigger but the gross
margins are a lot lower as you get out
into building out the fleet and that
might be a relatively like when I look
at the numbers I could see that
transition from being I could see
they're super profitable you know
because you're just taking ride haill
business and there's a lot of demand and
you like you basically can't build
enough cars to fill the demand like that
could last a couple years easy like will
it last five years maybe I don't know
that seems long to me uh and it's
there's it's not going to abruptly end
you know there'll be this long taper
into this long-term thing where like I
think you know there's I mean what is
the end State like is it 20 years 50
years you know you get different windows
at different things but I the other
point I like to think about is the point
where it's commoditized like the lwh
hanging fruit of vehicle miles traveled
for you know like you your your Robo
taxi it costs 4050 cents a mile it shows
up in 3 minutes it's super convenient
you can rent a two-seater four- seater
minivan you know like there's a lot of
variety lot of
accessibility and it's less expensive
than owning your own vehicle and half of
all miles have moved over to that and so
why do I say half and not 100% or some
other number
um one is human habits change slowly you
know so that people tend to not do make
transitions to new technologies as soon
as they you know we you you the tail end
of the adopter curve and I you know
there are aspects of the of the robo
taxi adopter curve like moving off of
private vehicles on a robo taxis which I
think for various reasons are likely to
be more slow than than say uh you know
moving to cell phones or smartphones off
of you know galpagos dumb phones was uh
even though that took 10 years plus for
us to make that transition but it's an
interesting point to talk about because
it's that's a point we're definitely
going to get to we're definitely going
to get you know when we have 25 million
Robo taxis on the streets in the United
States they'll be supplying like half of
vehicle mile travels and I like that
because it's really hard to argue that
we won't at least get to that point so
you can talk about that model you can
talk about the model when you have 123
million robot taxies and that sort of
gives you an overall Spectrum to sort of
think about what's going on okay in
state two which I think probably comes
five years after State one maybe it's a
bit longer maybe it's 10 years I don't
think it's 10 years but maybe it is um
most of the car market is private
Vehicles it's not Robo taxis because uh
a smaller number of vehicles saturate
the robo taxi Market sooner and you know
if you still have a lot of vehicle miles
travel I mean because robot taxes drive
five times as many miles as privately
owned Vehicles do say five times um that
means it takes five times as many
private vehicles to satisfy the same
demand that that an equivalent number of
robot taxis could do so you so you after
you get out of this profitable Zone
where you know you have a small number
of robot taxis because your production
constraint or jurisdiction constraint
regulation
constrained uh after you get out of that
zone uh like I my see the way I see this
thing is Tesla is going to have this a
huge demand for robo taxis over some
window of time and that that's going to
taper and most of their business you
know in this longer term is private
Vehicles again right so how do you
manage that as a company like you don't
want to leave anything on the table
during the Gold Rush when the Robo taxis
are making a ton of money and you're
rapidly scaling out the thing but you
also don't want to gut your long-term
prospects of continuing to be you know a
viable manufacturer like you can't walk
away from the car business for five
years and feel like you're just going to
pick it up you know I mean you got a
supercharger Network to keep going you
got to keep your service centers going
you have sales people you have like all
these channels your manufacturing design
goals all that kind of stuff they're
different between the the between the
two robotaxi I think will be crazy
profitable through some window of time I
think it'll be decently profitable and
huge long term right so that's the arc I
see for those things but I but I'm
skeptical about the there are people who
feel like the economics of robotaxis are
so good that they expect a wholesale
abandonment of private
ownership is that possible I think it's
possible I just don't like that's not
the base case to me of what's going on
and I think whatever
strategy Tesla uses has to be prepared
for both eventualities and the the
flexible strategy that guarantees your
future is to keep a foot solidly in the
retail Camp all the way through this
transition sure um in terms of timeline
of when we can get unsupervised um FSD
or robotaxi starting to roll out I know
there's going to be different
municipalities different cities um it's
going to be a a phase roll out where
you're going to have start with certain
places that are more permissible you
know and it'll be a smaller Fleet to to
try out kind of like what weo is doing
for example in a few cities and then you
you gradually you know roll it out more
I mean I imagine Tesla R will be a lot
faster because I think their rate of
improvement is going to be tremendously
fast especially once they get to that
point but would you say um timeline of
expectations when do you think the first
when do you think Tesla will first test
out kind of unsupervised Robo taxis on
the streets kind of like weo in a city
do you think it's second half of
2025 um uh test like if they're I think
say more than 50 vehicles in a city this
year with Tesla employees behind the
wheels ex you I'm talking about like no
no one in the car and taking passengers
kind of like what weo is doing with no
one in the car yeah
that like I wouldn't expect to see them
doing it this year it's going to you
know we're seeing this sort of dislo
discon discontinuous sort of rate of
improvement yeah and you know we don't
know what the next six months holds
Tesla has a way better idea than we do
so it's conceivable that they're
confident about this and they feel like
they could try to do that this year um
like that seems super aggressive to me
yeah um
the uh and you know they're gonna just
as way Mo Cruz Uber did they're going to
go through this long period where they
have employees sitting in the cars
trying not to touch the wheel anymore
than they have and they're racking up
miles and they're getting a sense of how
well the thing works and I don't think
that that's going to be 10 cars you know
I think that's going to be 500 cars kind
of thing various places maybe various
countries and they're you know that's
going to be a way of GA gathering data a
way of providing feedback to that AP
team about things that have to be done
uh it's going to be a way for management
to develop a strategy or get data to
help inform a strategy for how they're
going to proceed and I would expect that
to happen this year interesting um now
you know what fraction of the drives
will be totally intervention free will
it be 99% will it be
99.99% I mean I think that's open to
debate and it it very much depends on
what we haven't seen the slope of
improvement for V12 yet and so it's hard
to have an informed decision so do you
think these uh this test these like test
of employees in let's say these Robo
taxis are they going to be picking up
passengers and driving them or so both
Crews and weo did a thing where they had
company internal passengers
for years I think and San Francisco Cruz
had company internal for like two years
or something weo did it for quite a
while I think weo is doing that with p
with
employees in Austin now that's like the
first stage is you your own employees
get to use it it's you know and then uh
weo did a thing where they did they had
a long thing in Chandler Arizona where
they had you know customers under NDA as
they were working through and it turned
out to be long because obviously you
know they weren't making progress as
fast as they wanted to you know in terms
of like polishing off all the things or
maybe they became more conservative you
know they were in that window for a
really long time um like I don't why
that wouldn't be a good idea for Tesla
to have you know internal people and
then you have external people just like
with the safety score thing you know you
have a population of people you know who
are who ride as passengers maybe under
NDA maybe not under NDA and you know you
just as your confidence builds and you
have more vehicles on the road and
whatnot you gradually open up you know
you let people see what you're doing
partly because you have to because as
your scale goes it's too hard to keep
things you know Under Wraps like I I
would expect them to be starting that
process this year and like how quickly
they move through the various stages of
like scaling up the vehicles having more
and more things uh that's um you know
that's going to depend on the techn I I
I really do believe the the tech is the
fundamental thing yeah I mean that's
interesting because um in the just in
the Bay Area and in like Austin they
could roll out you know Tesla or
employee passengers right and employee
driver pal Alto first probably yeah pal
Alto Fremont Austin factories whatever
um that would be I mean they have plenty
of plenty of people there's plenty of
employees that they could do I mean they
how many people do they have that
commute to their factories every day you
know I mean imagine you know having a
fleet that just brings your your line
workers and you know yeah and so you run
a shuttle service for line workers and
use Robo taxis yeah I wonder if the
August 8 reveal will share some of those
details you know like what do you think
like been cool if it did it would I I
I've been like my guess is we won't get
a ton of detail because they don't we I
you know battery there occasionally we
do get a lot of detail right I mean the
AI days have never given us a ton of
detail on strategy um the battery day it
kind of did so there's precedent for
maybe getting more data so if they if
they think of Robo taxi as more like but
the other thing is the like there's this
variable about um you know to what
degree do people with
Teslas get to participate in the Tesla
Network right you know when when Elon
first announced the Tesla network was
going to be a thing Robo the dedicated
robot taxi was pretty far away so
there's a lot of incentive to like get
um the the other thing is like when they
initially did it they didn't have the
cash reserves they had now like the idea
of like building your own Fleet from out
of based on your own Pockets or
borrowing money to do it that would have
been a lot scarier back when they were
thinking about that like now they could
scale moderate size fleets with their
existing cash reserves and it could
totally make sense like could be a
no-brainer of a thing and so my guess is
like the optimal strategy has probably
shifted but there are lots of people who
expect to be able to participate in that
and we're looking forward to it and I
like I didn't go back to read what the
con cont language was when when we
bought these things but you know that
was part of the promise that FSD got
sold on in the early days so I'm still
expecting that to some extent they
expect participation now what are the
terms how many people get involved you
know that's we like we don't know what
that is these are very these are knobs
they can turn to tune the
strategy I mentioned the the thing like
I I feel like navigating this boom in
robotaxi sales and whatnot while
maintaining your retail business is
going to be challenging and these are
knobs that they can turn to try to keep
the market orderly while all this stuff
unfolds and you know you
know you know gain as what as much
benefit as they can provide as much
benefit as they can to their consumers
while not taking on unnecessary risk
yeah um is there anything about the
robotaxi like what's the biggest
difference between the robotaxi in the
$25,000 vehicle you think I mean like I
would say self-closing doors you think
you think that that important you know
when yeah when I think
about I you know when when I found out
they were doing a robot taxi I did a
couple of clean sheet things like what
would be a good Robo taxi like if you
were making it and uh when I think about
this stuff like what doesn't a model 3
have or a model y have that you want in
a robo
taxi uh you know there's a there's a a
bunch of things that I think are
nonobvious that have to do with Fleet
operation vehicles that that you know
they make sense they're totally coste
effective in a robot like a self-closing
door I feel like is a total is highly
cost- effective thing to put in a
$25,000 Robo taxi right just so that
your passenger doesn't walk off and
leave the door open right or you know
make sure the door is actually properly
closed and be able to properly close it
um but other stuff like you know being
able to check if somebody left packages
behind in the car making it so it's easy
to clean so that you know one of the
things taxi cabs one of the first things
wear out as a back seat you know cuz
people get in and get out so you want to
be able to you know easily swap out that
kind of stuff MH um it like I like the
idea of doing a cybertruck style you
know kind of really unusual looking
because it's it's for one thing it's an
advertisement the thing oh there's one
of those in the same way the
cybertruck's an advertisement there's
one of those Tesla robot tties right but
also you know being
dentree you know not needing as much
cleaning
care um so there's that obviously
there's sensor Suite stuff you know
there's um spending more money on the
sensor Suite spending more money on the
computer like all that stuff is more
justifiable in a vehicle that's using
the sensors and using the computer like
247 that is so like the economic
tradeoffs of that kind of stuff when
it's a gimme that you're putting on cars
and 90% of people aren't using it like
that's harder to justify than in a
robotx where you know they're going to
use it right so sure that's does it need
to be four doors like four-seater that's
a really interesting question so like I
went back and forth on this a couple
years back when I was looking at the
thing and my so two-seater attractive
like the fundamental economics of the
two-seater are pretty attractive but you
do have the thing where like like it
it's true that most rides are one or two
people right but n like 10% of the rides
are more than two people so of course if
you have two seaters they can take two
vehicles but like if you have two
parents traveling with children like are
they going to be happy with the two
vehicle kind of thing I uh you know and
a lot of people if your drive is more
than short and you're traveling with
your family you want to travel together
so you can talk kind of thing I mean
there's I feel like um from an
operational flexibility standpoint like
if you're going to build one vehicle
that the four- seater is the thing that
makes the most sense because the
overhead today the way our streets are
configured right I mean there's no
advantage to having a really tiny
vehicle today you're going to take a
whole Lane anyway you're not lightening
up congestion or whatnot you're just
reducing the cost of the vehicle I feel
like the four four-door vehicle if
you're just going to build one vehicle
and you're not going to make another one
for 2 or 3 years and this is going to be
the first one and you're going to start
scaling your robot taxi I feel like
there's a lot of argument to be made for
doing a four seater because it lets you
cover like
99.9% of the market or something like
that as opposed to 90% of the market MH
interesting um so I was thinking about
this about the whole idea of Tesla
becoming an AI company versus let's say
autom manufacture I was
thinking um it seems like the the P
autom manufacturer business it's just
I've never thought it's just very
cyclical low margins typically it's like
the software component is is the
Intriguing part adding extra value where
as an investor yeah yeah or you know
rather than the human you know invested
amount of time and energy and focused
Drive you're pulling that out off
loading it onto AI chip like that's
interesting that can drive margins Etc
um but it seems like this
transition from Tesla as a autom
manufacturer to AI company like it's
been happening over time and I would
argue that Tesla's like focus and
priority best Engineers all been on this
you know kind of AI um um trajectory but
just like for example open AI before
chat GPT sure they were AI company but
chpt made them kind of like a a real AI
company a AI company that people use
their products you know like a company
that's that's immensely useful right for
people as a AI company rather than just
a research lab right before that in a
sense and I think in some ways when I
drive at V12 I'm like oh it feels like
Tesla is getting close to this point
where FSD is going to be really really
useful right it's like unsupervised FSD
is going to you know transform people's
you know driving trans experiences and
it'll get to this point where Tesla's AI
products are finally in the hands of of
lots of people in a very useful way and
that to me marks kind of like this big
transformation in Tesla's history when
we look back 20 years from now you know
we'll say oh that was kind of the moment
where everything crossed over like it
wasn't it's not so much that again like
open a wasn't an AI company they're more
like a research lab MH but then when
they came out with their product it
really transformed so in a sense I look
at Tesla up until now the AI part of
Tesla it still feels like more
like lavish to this point you know where
the the real products haven't come out
you know for for for for Millions to use
it so it just seems like we're getting
closer and closer to this pivotal point
in in Tesla's kind of History I wonder
if people if their impression will
change like we don't think of Apple as a
software company even though software
and the ecosystem that they build and
the stores and all that kind of stuff
arguably add more value than building
the laptops the phones that kind of
stuff right um I mean not just the
software but the ecosystem the software
enables you know both the cloud stuff
and the software that goes on the that
but we still think of Apple as a phone
company as a laptop company and whatnot
like the the software becomes like an
ingredient in the hardware but the
hardware is thing that you see so you
know I mean arguably Tesla's already you
know the the software content of the
cars is super high and it has all these
Network features and stuff and yeah the
world even Tesla fans they don't really
see them as qualitatively different than
other cars it's a different kind of car
and we still view it as a as a car so
even though the you know the economic
reality the company and the operational
reality the company May shift away from
being more about the car and more about
like the ecosystem and the services and
that kind of stuff I don't know that
that the like I wonder if they will
change and by extension like will
investors who you know mostly they're
Ordinary People they're not experts yeah
right will their perception of the
company shift it might I I think a big
part of it is going to come down to uh
like uh you know we don't think of
Amazon as a grocery store we still think
of it as an internet store right because
we went through this thing but you know
when the internet company all took off
back in the 20s and Amazon just became
an internet and you know it Amazon is
probably way more Hardware than it is
internet at this point I mean if you put
aside the AWS part which is a very
important part of the thing you know I
mean it's delivery Vans and
warehouses you know and vast inventories
of stuff and and there's a you know
there's this other component too but we
think of it as an internet company
that's true so it'll be interesting to
see you know if and what is trigger if
if if if we if if if Tesla ever escapes
the car maker thing I it's not clear to
me it ever will I mean I guess Apple
being like Steve Jobs defined apple as
more of a device company that's always
been their thing I it's possible Tesla
follows in that sense where they're a
car but also a robot humanoid robot
company that type of devices in in those
ways um but yeah on Optimus I wanted to
ask ask you your latest thoughts on kind
of where Tesla's at um do you think
they're going to start some limited
production run in the next year or so or
are we still kind of a little bit
farther out than that that's a good
question I I
mean okay so I I think they're still
getting up the curve on software like
they everybody's still getting up the
curve on software the thing is the
humanoid robot software stack is
evolving that's like the llm stack it's
just evolving Crazy Fast
um
the like if it the reason that I thought
Tesla should make humanoid robots right
is because I see the software as
happening now you can make it happen
sooner but the underlying Tech that
makes the software possible for doing
those it's just coming we can speed it
up some but it's coming for sure right
and the the the ingredient that I
thought was missing to make humanoid
robots happen big happen soon was
that you want to be able to build them
at scale and you want to be able to
build them cheap you want good cheap
robots built at scale and I didn't see
the industrial infrastructure out there
in the world or anybody preparing at the
point that we first talked about this to
make that in infrastructure and that's
the long PLL on doing this stuff like
the software is going to happen it's
kind of I mean we're going to pull it in
now that there's a lot of interest it's
going to happen sooner than it would
have otherwise but it was going to
happen these techniques were going to be
developed right and so so was the fact
that there were no good robots out there
going to be the limiter the reason why
it didn't get adopted and you know in
2028 as opposed to 2038 became the big
year that it goes so you know to like
when I look at at through this lens and
my sense is that there are people in
Tesla who look at at the like you know
they very clearly understand the
challenge of of industrial stuff at
scale you know and they understand that
that a problem that needs to be properly
addressed in order for this product to
really fulfill its potential and that
there's a there's a big first mover
advantage in getting their first not
just a first mover Advantage but a
sustainable Advantage right because you
get their first and then you don't stop
you keep developing you always have the
best product right so you command the
best margin and you also have the
platform that let your software people
move forward the most quickly right um
it lets you get to scale and keep the
scale because building things at scale a
lot of building things at scale is about
harnessing the advantages of scale and
and maintaining that advantage means you
want to maintain the Lion Share of the
market because that gets you the scale
to let you hold that position and
maintain it and maintain the margins
associated with that position so like I
look at this and I imagine that you know
if Tesla sees it the same way and a lot
of the stuff that they say suggests to
me that they do see it this way that
their Focus right now is on like getting
the hardware down right building stuff
and getting it out there like if it
helps them get the manufacturing line up
if it helps them understand the product
better so they can build a better
product so that they can build the
product that builds the product better
like I think they'll do it they'll
they'll do that but it's a good question
we've just seen so little of Optimus in
action we've seen so little of it in
terms of you know
detailed uh uh information about the way
that it's built that understanding where
they are in the process of
industrializing it is tough but my
sense was has been for some years now
and still is that there's a lot of
really fundamental Improvement in the in
the tech that's available that you can
keep turning that
crank and you know every single year the
product that you can make is going to be
a lot better so to some extent timing
the scale up of the manufacturing with
when the software is really useful like
that's a thing that makes sense to me
because if you build the robot a year
early you're not going to have as good a
robot as you will a year later right
it's a longer the longer you delay
scaling the better product design and
stuff the more you're going to know the
better the core techn just you know for
Teslas came out and for years the motors
got better and better sometimes the
motor in your car would get better they'
do firmware updates because they'd
figured out something new or they could
change the margins you know early Teslas
if you had an early model 3 you know the
battery capacities would change right
because they change the software
um but there's stuff that you can't
change without actually changing the
hardware out too right and we did see
you know the motors that go in the cars
today are much better than the motors
that went in like two years ago 5 years
ago and so on because they're still
learning that kind of stuff
so yeah yeah whereas uh like I sort of
expect them to not scale until the
software is fairly mature but I expect
the focus to be on scaling the indust
the industrial capacity I see so I mean
Elon has said like often times takes
three generations of product of a
product before it gets you really good
so they're on gen two supposedly so
maybe one more Generation Well was the
first one of product I would argue that
Bumblebee and bubble seed weren't really
I would I would argue that the first
Optimus wasn't really a product I mean
they're they're going to make these test
mules essentially where they're figuring
stuff out yeah um but I think they're
calling it Gen 2 right yeah sure but I
think third generation product is third
generation product customers I see
that's true that could be thing I wonder
if the internal thing though is
developing three really you know
prototypes and then you know starting
your first product after that so maybe
we see one more gen 3 prototype and then
we start to see some type of initial
production a good question is when when
do you get to the point where uh having
more robots accelerate your development
Pro because like if they're I mean this
is a thing with the fleet for FSD cars
right once they got to a point where
they had data ingestion engine and the
data from the fleet was a major limiter
on how fast it could improve while
having a bigger Fleet is a really big
Advantage um I would guess that Optimus
isn't at the point right now and there's
this interesting thing about Gathering
data like you know having Optimus the
platform itself Gathering data to the
extent that you can do it efficiently is
pretty useful but like having humans put
on you know sensor stuff and go around
and do stuff that's actually a not
unreasonable mechanism in certain ways
it's better than having like for
instance if you want to do human
mimicking yeah well there's kind of two
ways you can do it you have a human
driving an Optimus right or you can have
you know an Optimus mimic a human and
those both have different strengths and
weaknesses but they're both things you
want to do and they both involve a human
in the loop right so you know if you've
got 50 operators there no point in
having a thousand optimi right because
you can only use 50 of them at a time if
you get to a point where the software
can start doing stuff on its own then it
makes sense to start scaling up I would
guess what do you mean when the the
point that software does it on its own
like say for instance that you're
working in it that you have some basic
task that you can do in a factory that's
you know that makes sense to do you know
like you it's economically useful or you
have some space to do it and you can set
some optimi aside in order to uh in
order to work on this thing well then
they can kind of autonomously gather
data by repetitively doing a task with
some variation you know and you we see
other robot like Google had a robot lab
that had like you know hundreds of robot
arms that were just basically doing
repetitive tasks over and over and
varying them to gather data so you can
do that kind of thing too it's it you
know I don't know if it's compatible
with the way that they're trying to do
the stack in Optimus right now but if it
is then it would make sense to like have
a thousand of them and find something
for them to do um but that's a question
like you know there's I think you're
going there's going to be the scale up
where they build a bunch of them and
they use them internally prior to any
customers getting them and them going
externally uh so it's interesting
question to ask when they do that and
when they that depends on the
development path that they're doing and
what their strategic path that they see
is I still don't see op like I still see
FSD as a significantly more near-term
product than I see Optimus despite so
how does Tesla let's say scale human
mimicry of humanoid robots like with
Optimist like
so let's say they need quantity of data
and quality of data so do you have I
mean you're talking about human control
the robot but then would it be better
just to have a suit or or a bunch of
sensors on the key parts so that you
know how the human is doing it when you
have a human and we've seen we know
Tesla does this already they've already
demonstrated you know a guy in a VR rig
who has some hand uh controls that he's
using to basically do you know upper
body men you know stuff rearranging
stuff on a table we saw the folding of
shirt thing that was how that was being
done in fact the the folding shirt video
might have been a data cap might have
been somebody in the process of data
capture I'm folding a shirt with Optimus
so like you put on your VR rig you take
Direct Control of an Optimus body and
then you use it to fold you know this is
a thing that's done this is one of the
ways that is known to be effective and
fairly um fairly sample efficient way of
gathering data measuring it straight off
of a human you can also do that and
people do do that stuff it has some
strength like because the exact
operating constraints for a human are
different and you you just have the hand
targets and stuff you don't have all the
inter mediate joint positions and that
kind of stuff so you get less data but
the data Gathering rig is a lot less
expensive and uh so you can give it to a
whole bunch of people and they can take
it out in the real world you know they
can you know they can go down the street
and pick up garbage they can uh you know
they can fold cardboard boxes in a UPS
store they you know you because you can
just take it someplace so there are
constraints with trying to do it with
Optimus to use the body directly but
then there are advantages also and you
know the the the the trade-off between
those two is another one of those
empirical things that I was mentioning
you know there are going to be some
trade-offs what's the right mix of which
things that you do and then there's
reinforcement l u i mean reinforcement
learning in simulation for robots that's
known to work well uh and in fact using
reinforcement learning to train robots
to mimic humans is like one of the
primary Mo modalities for doing it
because uh robots have many more
operating degrees of freedom than cars
do
so a robot can mimic a human action in
many different ways some of which are
much more preferable to others like if
the goal is just like move your hand
through this Arc to pick this thing up
you know what is your upper body doing
what is your head doing these are all
free variables to train a robot you know
in a sample efficient way you'd like to
constrain all of those to something
reasonable so having a human control the
entire body so that you gather all you
know the opinions of the human as to
what those things should be doing and
make those targets too even though
they're not minimal necessary to the
maybe the target is to move the bottle
over here or pour a drink or something
like that right so uh I think you know
most in you know the reality is that all
of these processes get used in various
combinations because they all kind of
bring something to table and you know as
we were talking about you've got you
know pre-training in instruct training
and then rhf on a on a and other things
now that get used in training large
language mod like there's many other
stages that we're not mentioning
um you don't it's not a matter of like
which is the best one it's like you use
all of them to the degree that they
contribute to a rapid reliable solution
so do you think I mean it seems to get
for Tesla to to get a product out to
people they need to scale up the data I
mean the or what if it's human mimickry
or whatever unless you're doing I guess
some specialized you know you know
Factory tasks but even that if if it's
so specialized why do you need a
humanoid robot has to be somewhat you
know you know like there needs to be a
need for right more generalized it a lot
of you know great progress is being made
in robots robotics right now without a
huge Fleet of robots uh there are you
know scale that we were talking about
before like scale just wins if you can
scale but you know for scale to win with
Optimus you have to have you know a wide
variety of real world task that you're
deploying Optimus into where it's
operating with either without super
human supervision or where humans are
supervising it and they would have been
doing the task anyway before like so
that because the cost of like paying
10,000 people to stand around operate
Optimus you know eight hours a day like
it's it's it's super burdensome right
and more importantly you want to one of
the advantages of operating in the real
world is you want to take advantage of
the complex and entropy of the world
like if you got 10,000 optimine and
they're all standing in white cubicles
that are basically the same and they're
just moving the same blocks
around uh part of the benefit operating
in the world is the long tail of context
and properties you know so if you give a
optimi to 10,000 people and you tell
them hey go use this on your farm hey
use this it you know try to use it as a
carpenter or whatnot and you can find
people who are enthusiastic about
investing their own time and doing it
maybe finding something useful for it to
do now what you're doing is you're
harnessing the variety that all of these
different people thinking about this
stuff in all the different settings and
environments that's where the data
really starts to if having a ton of
optimi in a factory all in approximately
the same context doing approximately
same thing it's not nearly as valuable
as having lots of different because
that's what that's what the cars get
sure each of the cars is serving a
different Master it's doing a different
set of task on a different set of roads
at different times of day in different
weather and whatnot so so the data it
gathers is bringing all of that Variety
in and that variety is really useful to
training these things mhm um Tesla I
mean that reminds me Tesla recently had
a job listing for like 10 or so
prototype Vehicle drivers and they're
like in these different cities all over
the US like why do you think they need
that I assumed it was because they were
checking out V12 and maybe Gathering
training it's you know having some I
mean you get two things out of having a
driver in Adelaide Australia right uh
one of them is get to see like is there
anything weird about Adela Australia
that breaks what we're doing um that we
should be paying attention to and you
get to gather data from Adela Australia
like as I was saying variety right and
different countries just have things
that are different and they'll different
driving cultures I mean uh when Brad
Ferguson went to New York he noticed
that you know FSD was driving like a New
Yorker you know humans change their
driving behavior depending on context
right some of that is cultural you know
you drive in Brazil you drive in Italy
and then you go drive in like England or
Germany and the driving cultures are
really the way people behave are
different right so just like being in
those environments and Gathering data on
the driving culture in that environment
that can also be useful I mean why can't
they just use you know their own hundred
rated 100 like safety score drivers in
those different cities instead why do
they need to hire separate drivers you
think yeah I wouldn't say they
necessarily I
it say you want to run a stack that
you're not confident is safe yet and you
want to give it control of the vehicle
so the first thing I said is like is
there something in Adelaide that breaks
our current stack well if you you know
imagine that you wanted to go test V12
but you were like four months away from
being able to roll it out well you can
go there and test it to see if there's
any big problems with it you know and
you know without taking the risk of
giving it to a retail customer and you
know you can put it on a single vehicle
if you have a professional driver that
you're paying you get a ton of data in a
small period of time and you choose the
data you can tell them we want data from
this situation go there and do this now
go to this other you know like the the
drivers doing Chuck's UPL
sure yeah interesting
um um llm so you talked about llms a
little bit so um what's going on where
is this all headed so in the bigger llm
picture so we have you know open AI they
just released a I guess update for gp4
yeah we'll see what it what the
capabilities are but then you um Claud
Opus has been destroying GPT at least in
my personal use it's beating out on
benchmarks and in a lot of people's
personal experience like I that may be
the reason that we that we we're getting
this gp4 turbo because you know one of
open ai's points of pride is you know
they've managed to stay at top the
leaderboard quite comfortably for a long
time with gp4 yeah I mean does this
change the the LM game to have like
anthropic being this I me be being able
to challenge open AI at least at this
point well game so everybody likes a
horse race and that's why the horse race
aspects of this stuff get played up um
so yeah the game that newspaper
reporters want to report on the game
that you know the bystanders like want
you know it gets more exciting when the
two horses are close together at the
nose uh does that change in an important
way the long-term dynamics of the market
I don't think from a standpoint it does
I think from a regulatory standpoint and
from the perception of the the markets
the breadth of the willingness of a wide
range of people to get involved and uh
like I think it might because it'll
change people's
perceptions and it might have an impact
on the real on on the outcomes because
it changes people's perceptions I think
most of it is you know people just like
a race and so like that that's part of
it I am you know mixt uh uh 8- uh 22b
came out I if you saw this that was
yesterday I'm going to download that
tonight
so that might be the first GPT 4 class
open source model yeah and that would be
exciting yeah I I doubt it's gp4 but
we'll see wait yeah I mean you know the
GP there's a there's a range of the gp4s
like because the current turbo like on
from a benchmark standpoint it's
interesting how like the performance on
benchmarks and the performance in
people's experience has kind of diverged
you know over time the uh the turbos you
know the later versions of gp4 they
continue to get better on benchmarks
right but there are a lot of heavy users
of of it their perception is that its
performance on the jobs that they're
doing has degraded so that's a really
interesting you know and I think one of
the reasons there's so much enthusiasm
about like the stuff that I seeing is
that a lot of heavy users people who are
building applications around this they
were delighted that cloud that Opus
wasn't having the problems that they
were that that they were experiencing
with with with gp4 as they felt like it
had degraded now you know it's it's hard
to know you know how much of this is
anecdotal how much of it represents the
real experience of everybody using the
tools uh certainly having competitors
out there gives you something to compare
it to you know so you get Alternatives
it's defin like having other models out
there is definitely for the good for the
field yeah uh it I it's about time if
you look at the rate of improvement in
the open source models for us to be
getting there you know it's a uh data
bricks had a model that came out
um uh it was another well there's a four
of 16 mixture of
experts UH 60 billion parameter is this
right that kind of scale 150 billion
parameter M super high performant on
Industrial low we're starting to see uh
the ecosystem very kind of just you know
veryy out where you see models that
where the the people building them are
specifically have certain types of
workloads certain kinds of applications
in mind and so the models can get good
at those without necessarily showing up
in the benchmarks you know so the people
who work in that space that set of
applications there's a command R Plus MH
is out which is uh that's another big
open source model it just came out like
in the last like couple weeks and it's
optimized for doing like rag
applications you know back office type
stuff where you use it in agentic ways
you build it you wrap an agent wrapper
around it and it it's been specifically
trained for all these modalities that so
like like we don't know how good that is
right now because it's not optimized for
the kinds of things that like it does
find on the benchmarks yeah for its size
but you know as Andrew wi has been
pointing out a lot recently if you wrap
a model in an agent you get much higher
performance on the same set of tasks
it's with somewhat lower reliability but
people are gradually figuring out how to
do that so you can get gp4 performance
from like 7 billion parameter models if
you wrap a good Agent around it and you
direct it at a particular task so like
it's really exciting to think like what
people building wrapping agents around
you know 150 billion parameter not maybe
not quite gbd4 CL but getting closed are
going to be and it's an open source
model like it's it's decentralizing the
power structure the knowledge base right
I I yeah I I I think it's actually huge
like open source getting to gp4 level I
think this year we'll get there um seems
like mistel it will deliver something
probably they've been really impressive
yeah they've been super impressive um it
just seems like that is significant
because the gp4 level is kind of this
Benchmark where llms get really start to
get really useful for a lot of things um
and once you can get that open sourced
um you can the the cost to access that
intelligence just drops like crazy
because you could basically download it
run it on your computer or eventually
that will be you know shrunk down and to
be able to run locally on different
devices or different things things um
the cost to access that base level of
intelligence will basically go down to
you know negligible cost I'm impressed
with what people demo running on iPhones
these days you know it's a Apple has
this uh this group of guys inside
researchers that developed this platform
called mlx which is basically it's like
Cuda for Apple silicon with like a pie
torch layer sort of built on top of it
so that it basically it's it's designed
like the you know the uh the new mix
model came out I mean they literally
just released the yeah released it for
download and like three hours later
people had it running optimized on Apple
silicon under mlx it's designed to like
make bring models in you know easy and
performant uh so you know people
building on that platform they can map
it to iPhones and that kind of stuff and
so there's there's a pretty good you
know ecosystem of demos out there where
people are taking whisper they're taking
all the you know various other models
and demonstrating what you can do by
quantizing them by doing Apple
themselves they released a paper last
year basically that was all about how
you change the design of a transformer
so you can run it out of flash like you
don't even have to load it into Dam you
just keep the weights in Flash and it
runs at full speed off of the CPU with
most of the weights being kept in Flash
like we're going to see like over the
next year or two like an a lot of
performance coming into these small
portable devices that you can carry
around yeah definitely and it's about
time cuz Siri
sucks yeah I think Apple's finally going
to announce something WWDC this year
it's so disappointing that it's taken
him as long as it has they'll do
something um Sora what's your take on
Open the Eyes uh text to video andin
Sora
it I'm you know it's a really cool
demonstr I I I think it's a
straightforward extrapolation of the
trends that had been that we've been
you know taken to video it's a cool tool
you know it'll be great when more people
get to use it I mean it's still you know
you're not going to just dump a prompt
into sore and get a movie out you know
it's a point in the Continuum it's a
nice step forward uh but I think it's
the kind of place that you would have
gotten throwing a lot of you know
compute at the problem so like like I'm
pleased to see I one of the things about
all these things like you see this Arc
of pro
right and every point that you make
along the Arc of progress like on
there's a part of it where you're like
you know right in line that's what we
expected but then there's there's always
this ah we haven't hit the plateau yet
you know that where you know you're and
that's kind of how I feel about Sora
right that yes the the these methods
they continue to scale and we're they're
going to keep getting better the
capabilities themselves are kind of in
line with the trend yeah it just seems
like with Sora it's super impressive to
me but I just think that it's taking a
lot of compute to run that thing and
it's not cheap and it's it's like a
proof of it it shows what's possible and
and people are going to be able to do
the similar thing with different methods
and it'll be a lot cheaper over time and
the capabilities will grow but it'll
take some time you know I mean um yeah
to I mean the difference between demoing
something and making it economical to
give the customers can be pretty large
on these things and I think open
themselves has said that you know they
need time to get it to where they can
offer it at a reasonable price yeah yeah
um we're almost wrapped up with our two
hours here um here in Austin um how was
your Eclipse viewing where did you see
did you ter I'm so miserable oh no I saw
the 2017 one and it was mindblowing I
was super excited about this and we we
ended up going out to kville because you
know I looked at the map ahead of time
there was no I was prepared to like go
you Vie it ended up kind being this toss
up I mean you've just got these Banks of
CLS coming and are you going to get
lucky and be between two clouds during
totality so we picked kville like the
day before it looked like it had the
best odds and we just totally struck out
I mean we it was it it's still cool to
be underneath the thing and see the sky
go dark and hear the animals all chain
you know I mean it's definitely
interesting I don't regret going I don't
feel like we made any bad decisions
going back looking at the data it was
still the best shot it was just like it
struck out like I was inconsolable the
whole day I was so bumped out wait so
the clouds were over the the whole time
no no I mean durity no we had the whole
thing where you know you'd see it in
between the clouds as there's or
whatever um but there were these couple
of different layers of clouds moving
back and forth and they had holes
between them and occasionally you'd get
a good view for you know a few seconds
or a minute or something like that yeah
but like just before totality this huge
thick Bank
just just like we didn't get anything
like I couldn't even look at eclipse
pictures for like 24 hours online I was
so bummed out that's funny that's Terri
it's too bad well I got to tell people
like if you've never seen one it they're
it is so worth it they're so like it's
super it's just a really incredible
experience to be out in an open space
under a blue sky and watch the Moon move
in front of the sun it it will change
the way you see the world yeah
definitely we had um my kids were really
into it I had them watch a bunch of
videos we bought some books on solar
eclipse and so they're really into
they're just like so excited yeah it's
fun yeah bummer but so now you know like
this morning I was like where's the next
one Australia is going to get a lot over
the next year maybe we're going to be
going to
Australia cool I really want to see
another one yeah yeah I really do fun
all right James thanks for hanging out
um yeah and um this was fun yeah yeah
we'll talk again hopefully soon all
right see you guys bye
5.0 / 5 (0 votes)
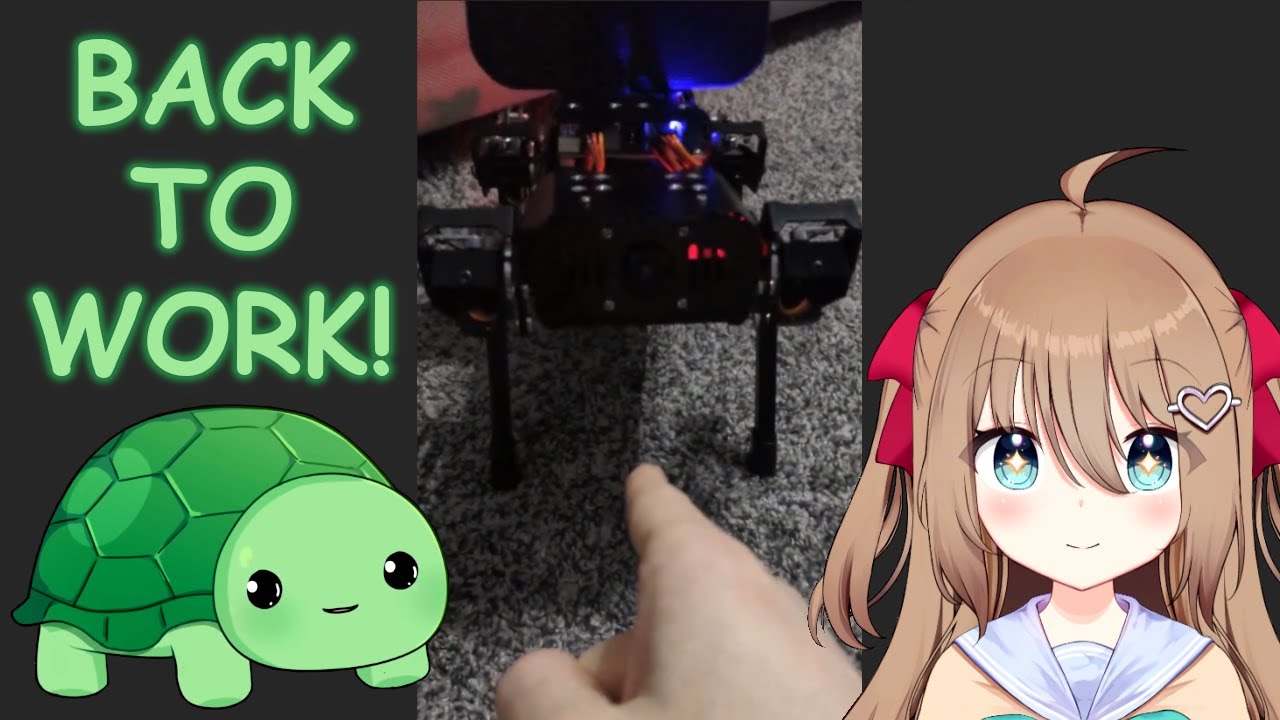
Vedal & Neuro Are Back And Reveal Their Progress
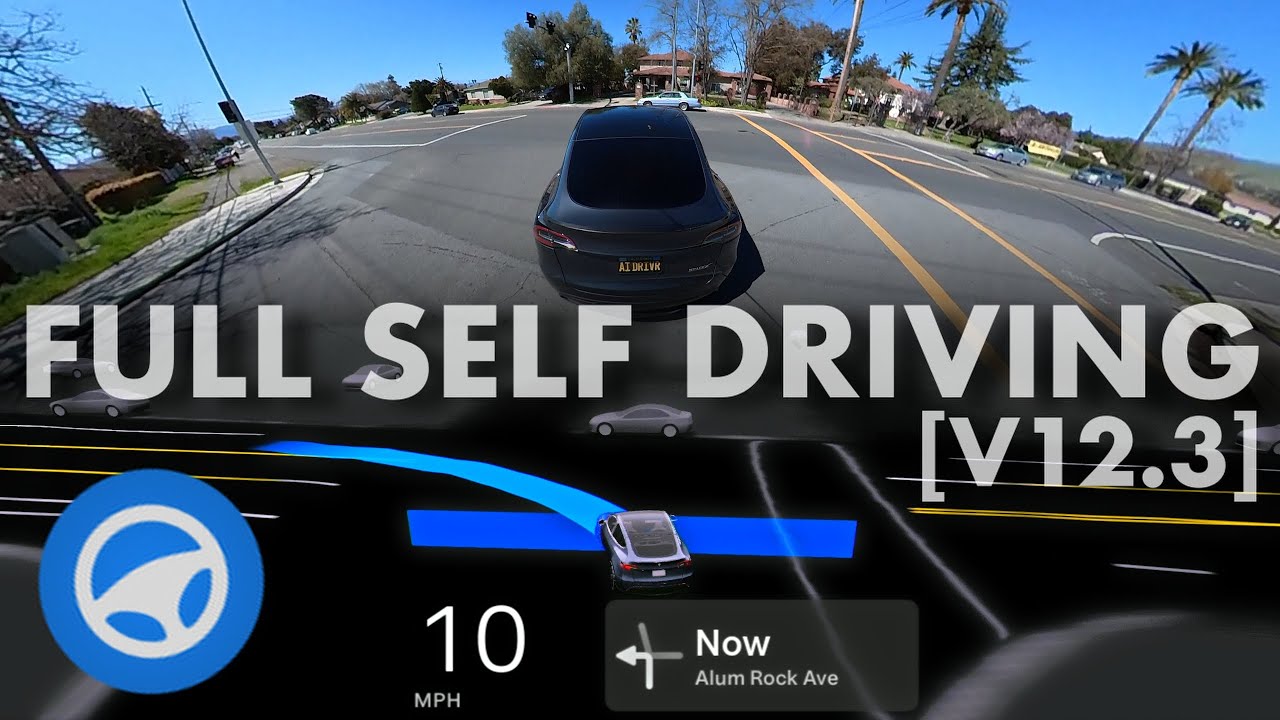
There’s a HUMAN inside Tesla FSD 12.3
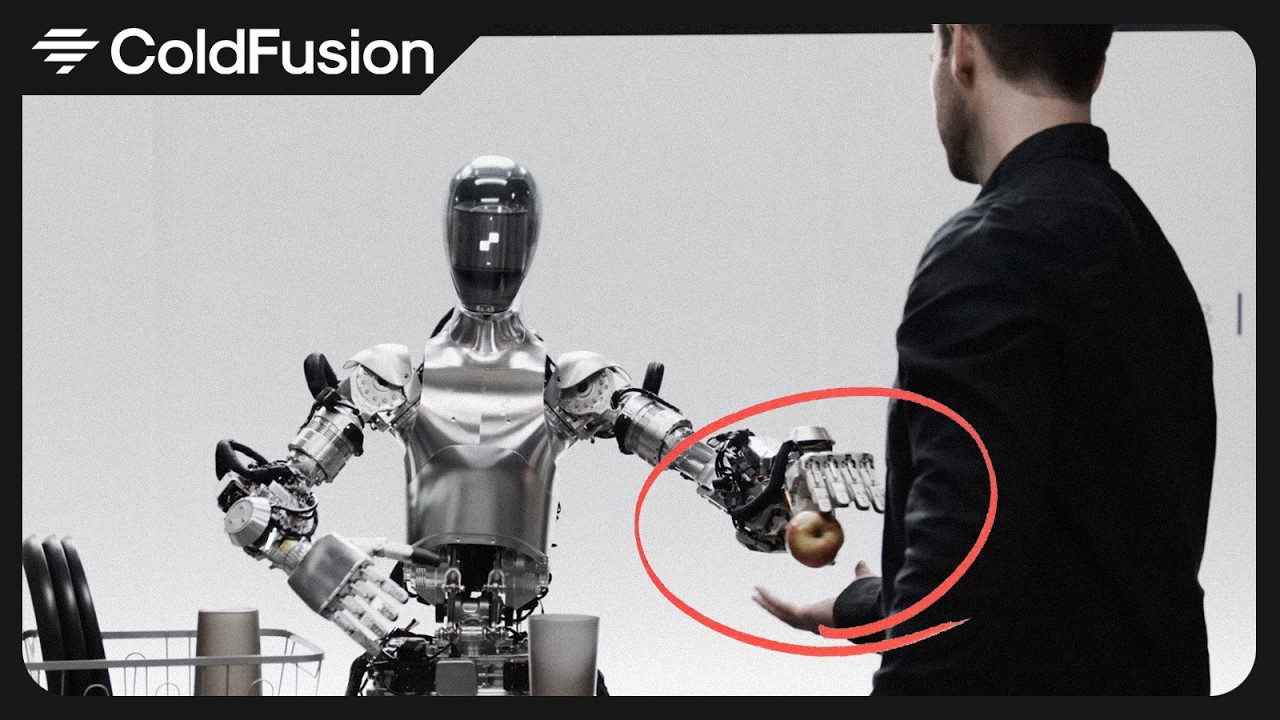
The Race For AI Robots Just Got Real (OpenAI, NVIDIA and more)
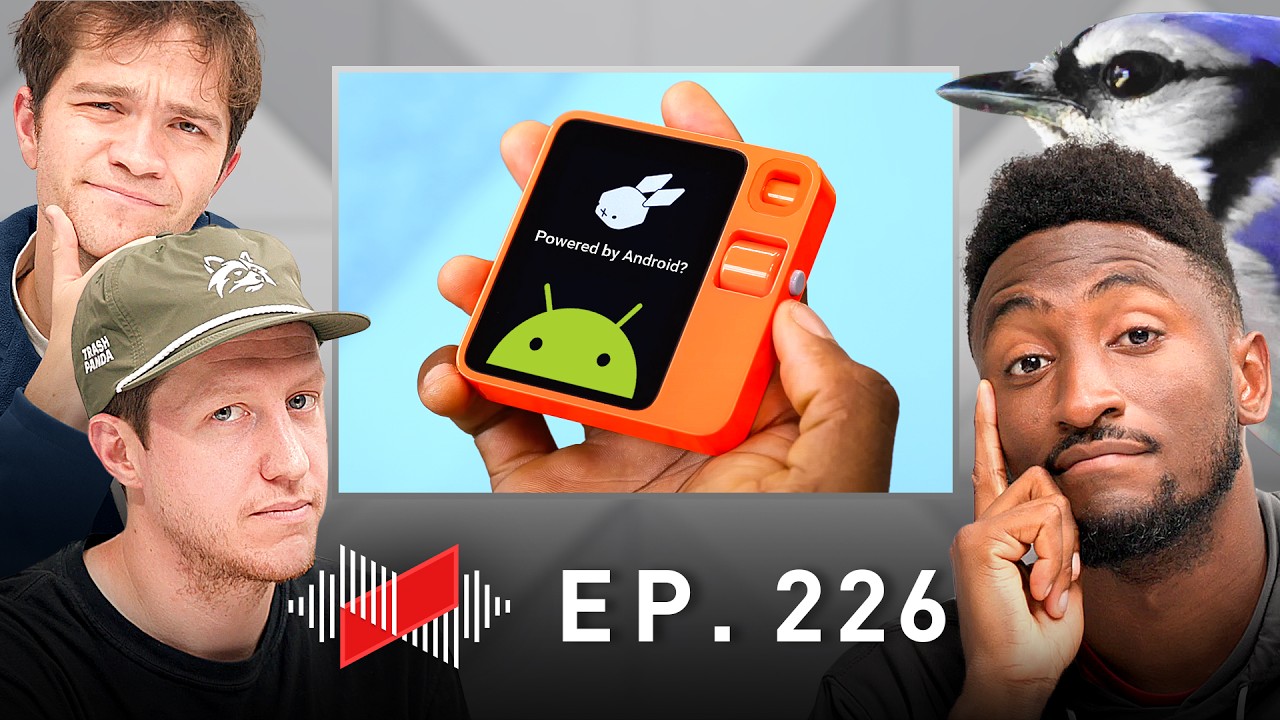
The Rabbit R1 is Just an App??
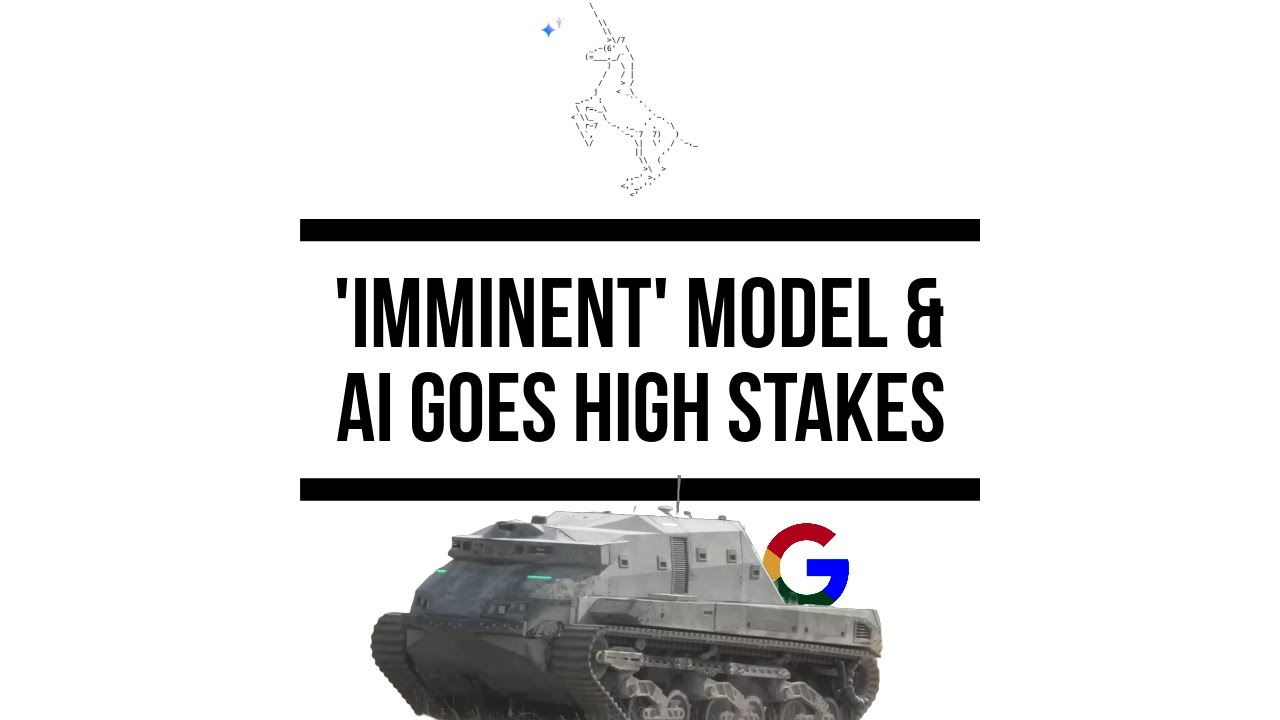
New OpenAI Model 'Imminent' and AI Stakes Get Raised (plus Med Gemini, GPT 2 Chatbot and Scale AI)

Thoughts on the Humane AI Pin