AI Agents Take the Wheel: Devin, SIMA, Figure 01 and The Future of Jobs
Summary
TLDREl script aborda tres desarrollos clave en inteligencia artificial que sugieren una era en la que los modelos AI no solo hablan, sino que también cumplen tareas complejas. Se discuten sistemas como Devon, un AI programador, Google DeepMind SEMA, un agente multijuego y un robot humanoide con capacidad de lenguaje. A pesar de su potencial, estos sistemas aún no igualan el rendimiento humano y se espera que con futuras mejoras, como la llegada de GPT-5, se verán incrementos significativos en su desempeño, lo que podría tener profundas implicaciones para el empleo y la automatización.
Takeaways
- 🤖 Los modelos de AI están evolucionando para no solo hablar, sino también ejecutar tareas complejas.
- 🚀 Los sistemas como Devon, SEMA y los robots humanoides están mejorando rápidamente, pero aún tienen un largo camino hasta el rendimiento humano en sus respectivos dominios.
- 🔄 Los avances en Devon, un sistema de ingeniería de software basado en GPT-4, sugieren que la transición a GPT-5 o Gemini 2 podría mejorar significativamente su desempeño.
- 📈 El Benchmark de ingeniería de software que se utiliza para evaluar a Devon es un reflejo de problemas reales del mundo, pero aún así no representa todas las habilidades del ingeniero de software.
- 💡 La capacidad de Devon para completar tareas en Upwork indica un futuro donde estas tareas podrían ser automizadas, aunque el costo y el tiempo de ejecución siguen siendo factores a considerar.
- 🎮 SEMA de Google DeepMind demuestra que con instrucción natural y aprendizaje profundo, los agentes AI pueden ser escalables y capaces de realizar una variedad de tareas en entornos 3D simulados.
- 🕹️ Los modelos actuales de AI, como GPT-4 Vision, están mejorando en el manejo de tareas en videojuegos y aplicaciones móviles, mostrando un rendimiento cercano al humano.
- 🤖 Los robots humanoides con capacidades de reconocimiento visual y manipulación están en desarrollo, y su inteligencia subyacente podría mejorar drásticamente con la llegada de modelos como GPT-5.
- 🌐 La visión del CEO de Figure de automatizar el trabajo manual y eliminar las necesidades de trabajos peligrosos y desalentadores es ambiciosa, pero también plantea preguntas sobre el impacto en el empleo y el control sobre el uso de la tecnología.
- 🔮 Las predicciones sobre el desarrollo de la inteligencia artificial general (AGI) sugieren un futuro cercano donde los avances tecnológicos podrían tener un impacto significativo en la sociedad y el empleo humano.
- 🌐 La preocupación sobre el control y la ética en el desarrollo de la IA es un tema importante que requiere atención y discusión pública para asegurar un uso responsable de la tecnología.
Q & A
¿Qué es Devon y cómo se diferencia de Auto GPT?
-Devon es un sistema basado en GPT-4 que no solo entiende las instrucciones sino que también puede leer documentación y desarrollar planes de acción, ejecutándolos de manera más efectiva que Auto GPT.
¿Qué es el Benchmark de ingeniería de software en el que se evaluó a Devon?
-El Benchmark de ingeniería de software es una evaluación que proviene de problemas profesionales del mundo real, compuestos por 2,294 problemas de ingeniería de software y sus soluciones correspondientes. Exige comprensión y coordinación de cambios en múltiples funciones, clases y archivos simultáneamente.
¿Cómo se desempeñó Devon en el Benchmark de ingeniería de software?
-Devon obtuvo un 14% de desempeño en el Benchmark, superando a Claude 2 y GPT-4, que tuvieron un 1.7%. Devon fue el único modelo evaluado sin asistencia, es decir, sin ser告知 exactamente qué archivos editar antes de la prueba.
¿Qué implicaciones tiene el Benchmark de ingeniería de software para el futuro de la ingeniería de software?
-El Benchmark sugiere que hay una rápida mejora en el rendimiento en tareas de ingeniería de software cuando los sistemas como Devon son equipados con modelos más avanzados como GPT-5, lo que podría llevar a una mayor automatización y cambio en la forma en que se realiza la ingeniería de software.
¿Qué es SEMA de Google Deep Mind y cuál es su objetivo?
-SEMA (Scalable, Instructable, Commandable, Multi-world Agent) es un sistema de Google Deep Mind que busca desarrollar un agente instructible capaz de realizar cualquier tarea que un humano pueda en cualquier entorno 3D simulado. Utiliza un mouse y teclado y toma pixels como entrada.
¿Cómo de generalizable es el agente SEMA y cómo afectó el entrenamiento en varios juegos?
-SEMA demostró un fuerte efecto de transferencia positiva, mejorando su desempeño en juegos nuevos después de ser entrenado en varios juegos diferentes. En algunos casos, incluso superó a un modelo especializado en un solo juego.
¿Qué robot humanoide se menciona en el script y cuál es la visión del CEO detrás de él?
-El script menciona un robot humanoide que utiliza el modelo GPT-4 Vision. El CEO detrás de él tiene una visión de automatizar el trabajo manual y eliminar la necesidad de trabajos peligrosos e indeseables, y eventualmente construir nuevos mundos en otros planetas.
¿Cuál es la estimación del costo del robot humanoide mencionado y cómo afecta esto el mercado laboral?
-El costo estimado del robot humanoide es entre $30,000 y $150,000. Aunque es demasiado caro para la mayoría de las empresas e individuos, la visión del CEO podría llevar a una reducción a largo plazo en los costos laborales y cambiar la dinámica del mercado laboral.
¿Qué cambios se esperan con la llegada de GPT-5 en términos de rendimiento de sistemas como Devon y SEMA?
-Con la llegada de GPT-5, se espera un aumento significativo en el rendimiento de sistemas como Devon y SEMA, ya que estos sistemas se beneficiarán de una mayor comprensión y capacidad de resolución de problemas gracias a la nueva versión del modelo.
¿Qué se sugiere sobre el control de la inteligencia artificial en el script?
-El script sugiere que, a pesar de los avances en la IA, no hay un control claro sobre cómo se utilizará la tecnología en el futuro y que la sociedad debería comenzar a prestar más atención a los cambios rápidos que está experimentando el mundo debido a la IA.
¿Cuál es la perspectiva de Jeff Cloon sobre la llegada de la IA general (AGI) y su impacto en la sociedad?
-Jeff Cloon cree que estamos cada vez más cerca de la IA general (AGI) y que no hay nadie realmente en control. Predice que la IA superará cualquier prueba humana en aproximadamente 5 años y tendrá un impacto significativo en la sociedad, incluyendo la automatización de tareas que hoy en día son realizadas por profesionales de la marketing.
Outlines
🤖 Avances en IA: Devon, SEMA y Robots
Este párrafo discute tres desarrollos clave en inteligencia artificial发生的最近48小时内,包括 Devon,一个AI系统由GPT-4驱动,能够执行编程任务;Google DeepMind的SEMA, un agente que juega videojuegos;以及一个 robot humanoido que demuestra una mejora significativa en la interacción con el entorno. Estos sistemas, a pesar de no estar aún a la par del rendimiento humano, sugieren un futuro donde los modelos de IA se actualizan rápidamente y se vuelven más capaces de realizar tareas complejas.
🎮 SEMA de Google DeepMind: Juego y Aprendizaje
En este párrafo se profundiza en SEMA, un agente desarrollado por Google DeepMind diseñado para ser instrucciónable y capaz de realizar tareas en entornos 3D simulados. SEMA utiliza datos de humanos jugando videojuegos para aprender y mejora su desempeño al ser entrenado en múltiples juegos, mostrando un efecto de transferencia positiva. Esto indica que, con la capacitación adecuada, los modelos de IA pueden generalizar habilidades de un juego a otro, acercándose al rendimiento humano.
🤖 Robot Humanoido con GPT-4 Vision
Este segmento describe un robot humanoido que utiliza el modelo GPT-4 Vision para interactuar con su entorno y realizar tareas. A pesar de ser impresionante en su habilidad para manipular objetos, su inteligencia proviene del modelo subyacente de IA. El robot puede tomar imágenes a una velocidad de 10 imágenes por segundo y realizar tareas de manera autónoma sin control humano. A pesar de su costo, el CEO detrás del robot tiene una visión de un futuro donde la automatización reemplace el trabajo manual, reduciendo los costos laborales y creando nuevas oportunidades en otros planetas.
🌐 Perspectivas sobre el Futuro de la IA
Finalmente, se discuten las implicaciones futuras de la IA, incluidos los comentarios de expertos en la industria que predecen un avance acelerado hacia la IA general (AGI). Se mencionan predicciones de que la IA reemplazará gran parte del trabajo humano en apenas cinco años, lo que podría llevar a una reducción significativa en los costos laborales y un cambio drástico en el panorama laboral. Sin embargo, se destaca la falta de control sobre cómo se utilizará esta tecnología y las preocupaciones sobre el impacto en los empleos seguros y deseables.
Mindmap
Keywords
💡AI modelos
💡Benchmark
💡GPT-4 y GPT-5
💡Reinforcement learning
💡Transferencia de aprendizaje
💡Robótica humanoide
💡Inteligencia artificial generalizada (AGI)
💡Economía humana
💡Programación
💡Juegos de video
💡Trabajo automatizado
Highlights
AI models are advancing to the point where they can perform tasks, not just process information.
Three AI developments in the last 48 hours show significant progress in AI capabilities.
Devon, an AI system, is equipped with a code editor and browser, allowing it to understand prompts and execute tasks.
Devon's performance on the software engineering benchmark was 14%, outperforming other models like Claude 2 and GPT 4.
The software engineering benchmark is based on real-world problems, requiring complex reasoning and coordination across multiple functions.
Devon was tested on a subset of the benchmark, which may not fully represent the scope of software engineering skills.
The benchmark may be biased towards easier problems due to the selection of accepted solutions and the introduction of new tests.
With the upcoming GPT 5, AI systems like Devon are expected to see significant upgrades overnight.
Google DeepMind's SEMA project involves training AI agents to perform tasks in simulated 3D environments using natural language instructions.
SEMA agents showed positive transfer effects, performing better on new games after training on multiple games.
The humanoid robot from Figure One demonstrates impressive dexterity and speed, controlled by an end-to-end neural network.
The humanoid robot's intelligence comes from GPT 4 Vision, which could be upgraded to GPT 5 for even deeper environmental understanding.
Figure One's CEO envisions a future where AI automates manual labor, potentially eliminating the need for unsafe and undesirable jobs.
The potential applications of AI extend beyond the current tasks, suggesting that AI could soon perform a wide range of activities on computers and phones.
AI's rapid progress suggests that we are moving closer to AGI (Artificial General Intelligence), with significant implications for society.
Experts predict that AI will pass every human test in around 5 years, indicating a timeline for when AI could match or exceed human capabilities.
The increasing compute power and algorithmic efficiency suggest that AI advancements will continue to accelerate.
Transcripts
three developments in the last 48 hours
show how we are moving into an era in
which AI models can walk the walk not
just talk the talk whether the
developments quite meet the hype
attached to them is another question
I've read and analyzed in full the three
relevant papers and Associated posts to
find out more we'll first explore Devon
the AI system your boss told you not to
worry about then Google Deep Mind SEMA
which spends most of its time playing
video games and then figure one the
humanoid robot which likes to talk while
doing the dishes but the tldw is this
these three systems are each a long way
from Human Performance in their domains
but think of them more as containers or
shells for the vision language models
powering them so when the GPT 4 that's
behind most of them is swapped out for
GPT 5 or Gemini 2 all these systems are
going to see big and hard to predict
upgrades overnight and that's a point
that seems especially relevant on this
the one-year anniversary of the release
of GPT 4 but let's start of course with
Devon build as the first AI software
engineer now Devon isn't a model it's a
system that's likely based on gp4 it's
equipped with a code editor shell and
browser so of course it cannot just
understand your prompt but look up and
read documentation a bit like Auto GPT
it's designed to come up with plans
first and then execute them but it does
so much better than Auto GPT did but
before we get to The Benchmark that
everyone's talking about let me show you
a 30-second demonstration of Devon in
action all I had to do was send this
blog post in a message to Devon from
there Devon actually does all the work
for me starting with reading this blog
post and figuring out how to run the
code in a couple minutes Devon's
actually made a lot of progress and if
we jump to the middle here
you can see that Devon's been able to
find and fix some edge cases and bugs
that the blog post did not cover for me
and if we jump to the end we can see
that Devon uh sends me the final result
which I love I also got two bonus images
uh here and here so uh let me know if
you guys see anything hidden in these it
can also F tuna model autonomously and
if you're not familiar think of that as
refining a model rather than training it
from scratch that makes me wonder about
a future where if a model can't succeed
at a task it fine-tunes another model or
itself until it can anyway this is The
Benchmark that everyone's talking
aboutwe bench software engineering bench
Devon got almost 14% And in this chart
crushes Claude 2 and GPT 4 which got
1.7% they say Devon was unassisted
whereas all other models were assisted
meaning the model was told exactly which
files need to be edited before before we
get too much further though what the
hell is this Benchmark well unlike many
benchmarks they drew from Real World
professional problems
2,294 software engineering problems that
people had and their corresponding
Solutions resolving these issues
requires understanding and coordinating
changes across multiple functions
classes and files simultaneously the
code involved might require the model to
process extremely long contexts and
perform they say complex reasoning these
aren't just fill-in the blank or
multiple choice questions the model has
to understand the issue read through the
relevant parts of the codebase remove
lines and AD lines fixing a bug might
involve navigating a large repo
understanding the interplay between
functions in different files or spatting
a small error in convoluted code on
average a model might need to edit
almost two files three functions and
about 33 lines of code one point to make
clear is that Devon was only tested on a
subset of this Benchmark and the tasks
in The Benchmark were only a tiny subset
of GitHub issues and even all of those
issues represent just a subset of the
skills of software engineering so when
you see all caps videos saying this is
Agi you've got to put it in some context
here's just one example of what I mean
they selected only pull requests which
are like proposed solutions that are
merged or accepted that solve the issue
and the introduced new tests would that
not slightly bias the data set toward
problems that are easy easier to detect
report and fix in other words complex
issues might not be adequately
represented if they're less likely to
have straightforward Solutions and
narrowing down the proposed solutions to
only those that introduce new tests
could bias towards bugs or features that
are easier to write tests for that is to
say that highly complex issues where
writing a clear test is difficult may be
underrepresented now having said all of
that I might shock You by saying I think
that there will be rapid Improvement in
the performance on this Benchmark when
Devon is equipped with GPT 5 I could see
it easily exceeding 50% here are just a
few reasons why first some of these
problems contained images and therefore
the more multimodal these language
models get the better they'll get second
and more importantly a large context
window is particularly crucial for this
task when The Benchmark came out they
said models are simply ineffective at
localizing problematic code in a sea of
tokens they get distracted by additional
context I don't think that will be true
for for much longer as we've already
seen with Gemini 1.5 third reason models
they say are often trained using
standard code files and likely rarely
see patch files I would bet that GPT 5
would have seen everything fourth
language models will be augmented they
predict with program analysis and
software engineering tools and it's
almost like they could see 6 months in
the future because they said to this end
we are particularly excited about
agent-based approaches like Devon for
identifying relevant context from a code
base I could go on but hopefully that
background on the Benchmark allows you
to put the rest of what I'm going to say
in a bit more context and yes of course
I saw how Devon was able to complete a
real job on upwork honestly I could see
these kind of tasks going the way of
copywriting tasks on upwork here's some
more context though we don't know the
actual cost of running Devon for so long
it actually takes quite a while for it
to execute on its task we're talking 15
20 30 minutes even 60 Minutes sometimes
as Bindu ready points out it can get
even more expensive than a human
although costs are of course falling
Deon she says will not be replacing any
software engineer in the near term and
noted deep learning author franois Shay
predicted this there will be more
software Engineers the kind that write
code in 5 years than there are today and
newly unemployed Andre carpath says that
software engineering is on track to
change substantially with humans more
supervising the automation pitching in
high level commands ideas or progression
strategies in English I would say with
the way things are going they could
pitch it in any language and the model
will understand frankly with vision
models the way they are you could
practically mime your code idea and it
would understand what to do and while
Devon likely relies on gyd 4 other
competitors are training their own
Frontier Scale Models indeed the startup
magic which aims to build a co-worker
not just a co-pilot for developers is
going a step further they're not even
using Transformers they say Transformers
aren't the final architecture we have
something with a multi-million token
context window super curious of course
of course how that performs on swe bench
but the thing I want to emphasize again
comes from Bloomberg cognition AI admit
that Devon is very dependent on the
underlying models and use gpc4 together
with reinforcement learning techniques
obviously that's pretty vague but
imagine when GPT 5 comes out with scale
you get so many things not just better
coding ability if you remember gpt3
couldn't actually reflect effectively
whereas GPT 4 could if GPT 5 is twice or
10 times better at reflecting and
debugging that is going to dramatically
change the performance of the Devon
system overnight just delete the GPT 4
API and put in the GPT 5 API and wait
Jeff cloon who I was going to talk about
later in this video has just retweeted
one of my own videos I literally just
saw this 2 seconds ago when it came up
as a notification on my Twitter account
this was not at all supposed to be part
of this video but I am very much honored
by that and actually I'm going to be
talking about Jeff cloon later in this
video chances are he's going to see this
video so this is getting very
inception-like he was key to Simo which
I'm going to talk about next the
simulation hypothesis just got 10% more
likely I'm going to recover from that
distraction and get back to this video
cuz there's one more thing to mention
about Devon the reaction to that model
has been unlike almost anything I've
seen people are genuinely in some
distress about the implications for jobs
and while I've given the context of what
the Benchmark does mean and doesn't mean
I can't deny that the job landscape is
incredibly unpredictable at the moment
indeed I can't see it ever not being
unpredictable I actually still have a
lot of optimism about there still being
a human economy in the future but maybe
that's a topic for another video I just
want to acknowledge that people are
scared and these companies should start
addressing those fears and I know many
of you are getting ready to comment that
we want all jobs to go but you might be
I guess disappointed by the fact that
cognition AI are asking for people to
apply to join them so obviously don't
anticipate Devon automating everything
just yet but it's time now to talk about
Google Deep Mind SEMA which is all about
scaling up agents that you can instruct
with natural language essentially a
scalable instructable commandable
multi-world agent the goal of SEMA being
to develop an instructible agent that
can accomplish anything a human can do
in any simulated 3D environment their
agent uses a mouse and keyboard and
takes pixels as input but if you think
about it that's almost everything you do
on a computer yes this paper is about
playing games but couldn't you apply
this technique to say video editing or
say anything you can do on your phone
now I know I haven't even told you what
the SEMA system is but I'm giving you an
idea of the kind of repercussions
implications if these systems work with
games there's so much else they might
soon work with this was a paper I didn't
get a chance to talk about that came out
about 6 weeks ago it showed that even
current generation models could handle
tasks on a phone like navigating on
Google Maps apps downloading apps on
Google Play or somewhat topically with
Tik Tok swiping a video about a pet cat
in Tik Tok and clicking a like for that
video no the success rates weren't
perfect but if you look at the averages
and this is for GPT 4 Vision they are
pretty high 91% 82% 82% these numbers in
the middle by the way on the left
reflect the number of steps that GPT 4
Vision took and on the right the number
of steps that a human took and that's
just gpc4 Vision not a model optimized
for agency which we know that open AI is
working on so before we even get to
video games you can imagine an internet
where there are models that are
downloading liking commenting doing pull
requests and we wouldn't even know that
it's AI it would be as far as I can tell
undetectable anyway I'm getting
distracted back to the SEMA paper what
is SEMA in a nutshell they got a bunch
of games including commercial video
games like valheim 12 million copies
sold at least and their own madeup games
that Google created they then paid a
bunch of humans to play those games and
gathered the data that's what you could
see on the screen the images and the
keyboard and mouse inputs that the
humans performed they gave all of that
training data to some pre-trained models
and at this point the paper gets quite
vague it doesn't mention parameters or
the exact composition of these
pre-trained models but from this we get
the SEMA agent which then plays these
games or more precisely tries 10sec
tasks within these games this gives you
an idea of the range of tasks everything
everything from taming and hunting to
destroying and headbutting but I don't
want to bury the lead the main takeaway
is this training on more games saw
positive transfer when SEMA played on a
new game and notice how SEMA in purple
across all of these games outperforms an
environment specialized agent that's one
trained for just one game and there is
another gem buried in this graph I'm
color blind but I'm pretty sure that's
teal or lighter blue that's zero shot
what that represents is when the model
was trained across all the other games
by the actual game it was about to be
tested in and so notice how in some
games like Goat Simulator 3 that
outperformed a model that was
specialized for just that one game the
transfer effect was so powerful it
outdid the specialized training indeed
sema's performance is approaching the
ballpark of human performance now I know
we've seen that already with Starcraft 2
and open AI beating DOTA but this would
be a model General izing to almost any
video game yes even Red Dead Redemption
2 which was covered in an entirely
separate paper out of Beijing that paper
they say was the first to enable
language models to follow the main story
line and finish real missions in complex
AAA games this time we're talking about
things like protecting a character
buying supplies equipping shotguns again
what was holding them back was the
underlying model GPT 4V as I've covered
Elsewhere on the channel it lacks in
spatial perception it's not super
accurate with moving the Cur cursor for
example but visual understanding and
performance is getting better fast take
the challenging Benchmark mm muu it's
about answering difficult questions that
have a visual component The Benchmark
only came out recently giving top
performance to GPT 4V at
56.8% but that's already been superseded
take Claude 3 Opus which gets
59.4% yes there is still a gap with
human expert performance but that Gap is
narrowing like we've seen across this
video just like Devon was solving real
world software engineering challenges
SEMA and other models are solving Real
World Games walking the walk not just
talking the talk and again we can expect
better and better results the more games
SEMA is trained on as the paper says in
every case SEMA significantly
outperforms the environment specialized
agent thus demonstrating positive
transfer across environments and this is
exactly what we see in robotics as well
the key take-home from that Google Deep
Mind paper was that our results suggest
that co-training with data from other
platforms imbus rt2 X in robotics with
additional skills that were not present
in the original data set enabling it to
perform novel tasks these were tasks and
skills developed by other robots that
were then transferred to rt2 just like
SEMA getting better at one video game by
training on others but did you notice
there that smooth segue I did to
robotics It's the final container that I
want to quickly talk about why do I call
this humanoid robot a container because
it contains GPT 4 Vision yes of course
its realtime speed and dexterity is very
impressive but that intelligence of
recognizing what's on the table and
moving it appropriately comes from the
underlying model gp4 Vision so of course
I have to make the same point that the
underlying model could easily be
upgraded to GPT 5 when it comes out this
humanoid would have a much deeper
understanding of its environment and you
as you're talking to it figure one takes
in 10 images per second and this is not
teleoperation this is an endtoend neural
network in other words there's no human
behind the scenes controlling this robot
figure don't release pricing but the
estimate is between $30,000 and
$150,000 per robot still too pricy for
most companies and individuals but the
CEO has a striking Vision he basically
wants to completely automate manual
labor this is the road map to a positive
future powered by AI he wants to build
the largest company on the planet and
eliminate the need for unsafe and
undesirable jobs the obvious question is
if it can do those jobs can't it also do
the safe and desirable jobs I know I'm
back to the jobs Point again but all of
these questions became a bit more
relevant let's say in the last 48 hours
the figure CEO goes on to predict that
everywhere from factories to Farmland
the cost of Labor will decrease until it
becomes equivalent to the price of
renting a robot facilitating a long-term
holistic reduction in costs over time
humans could leave the loop Al together
as robots become capable of building
other robots driving prices down even
more manual labor he says could become
optional and if that's not a big enough
vision for the next two decades he goes
on that the plan is also to use these
robots to build new worlds on other
planets again though we get the
reassurance that our focus is on
providing resources for jobs that humans
don't want to perform he also excludes
military applications I just feel like
his company and the world has a bit less
control over how the technology is going
to be used than he might think it does
indeed Jeff cloon of open AI Google deep
M SEMA and earlier on in this video Fame
reposted this from Edward Harris it was
a report commissioned by the US
government that he worked on and the
tldr was that things are worse than we
thought and nobody's in control I
definitely feel we're noticeably closer
to AGI this week than we were last week
as Jeff cloon put out yesterday so many
pieces of the AGI puzzle are coming
together and I would also agree that as
of today no one's really in control and
we're not alone with Jensen hang the CEO
of Nvidia saying that AI will pass every
human test in around 5 years time that
by the way is a timeline shared by Sam
Orman this is a quote from a book that's
coming out soon he was asked about what
AGI means for marketers he said oh for
that it will mean that 95% of what
marketers use agencies strategists and
creative Professionals for today will
easily nearly instantly and at almost no
cost be handled by the AI and the AI
will likely be able to test its creative
outputs against real or synthetic
customer focus groups for predicting
results and optimizing again all free
instant and nearly perfect images videos
campaign ideas no problem but
specifically on timelines he said this
when asked about when AGI will be a
reality he said 5 years give or take
Maybe slightly longer but no one knows
exactly when or what it will mean for
society and it's not like that timeline
is even unrealistic in terms of compute
using these estimates from semi analysis
I calculated that just between quarter 1
of 2024 and the fourth quarter of 2025
there will be a 14x increase in compute
then if you factor in algorithmic
efficiency doubling about every 9 months
the effective compute at the end of next
year will be almost a 100 times that of
right now so yes the world is changing
and changing fast and the public really
need to start paying attention but no
Devon is not Ai No matter how much you
put it in all caps thank you so much for
watching to the end and of course I'd
love to see you over on AI Insiders on
patreon I'd love to see you there but
regardless thank you so much for
watching and as always have a wonderful
day
4.9 / 5 (32 votes)
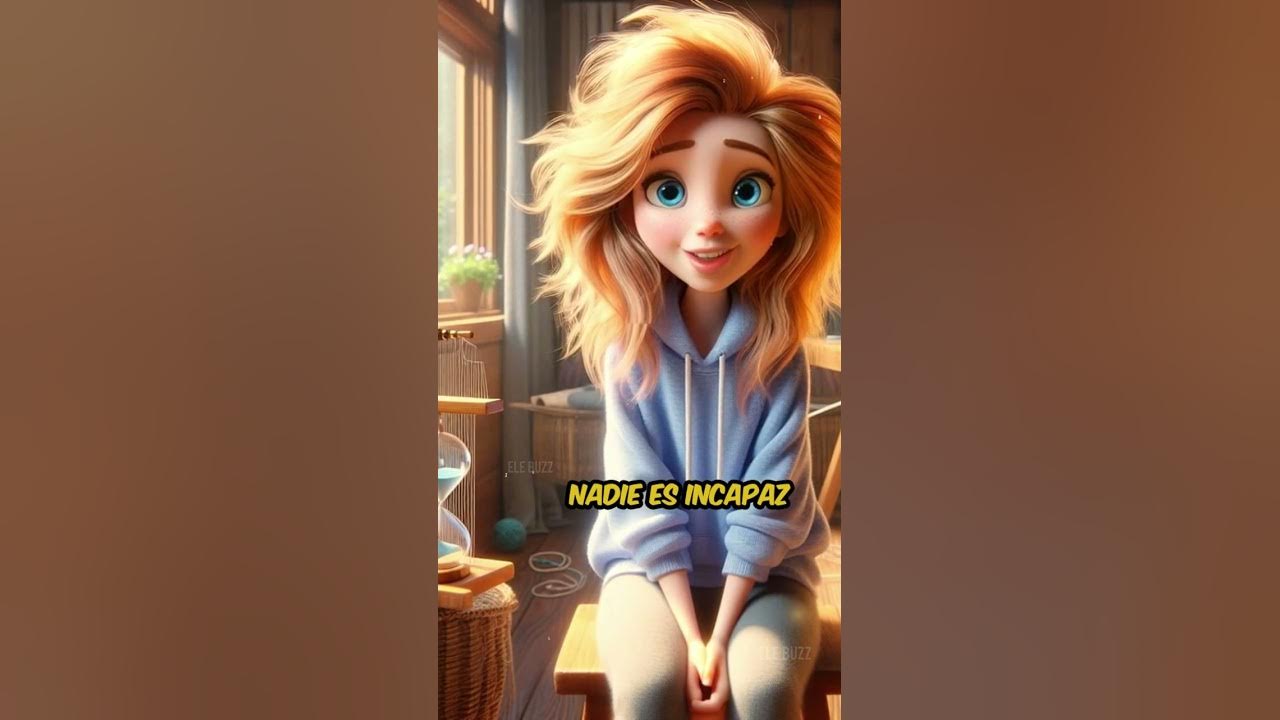
Paciencia todo llega, todo pasa
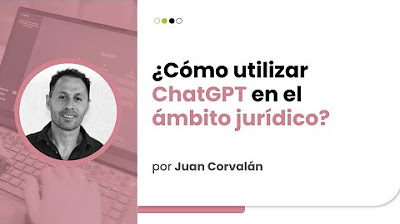
Juan Corvalán | ¿Cómo utilizar ChatGPT en el ámbito jurídico?
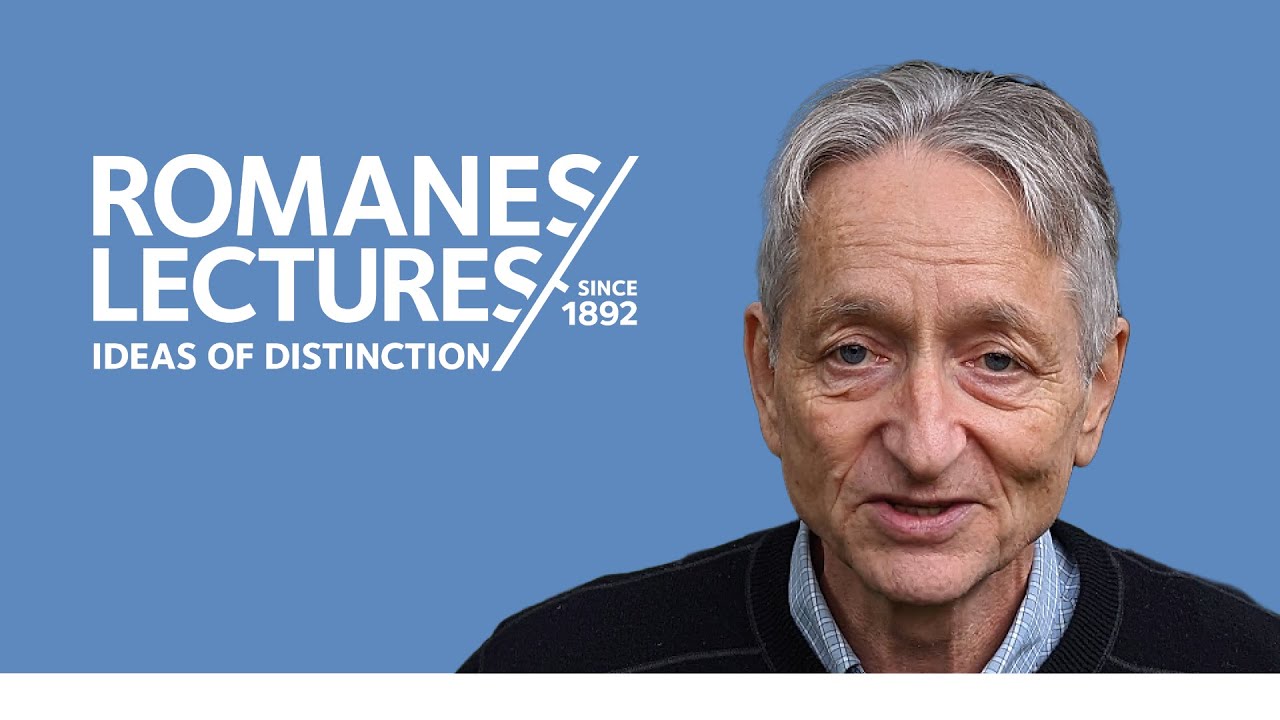
Prof. Geoffrey Hinton - "Will digital intelligence replace biological intelligence?" Romanes Lecture
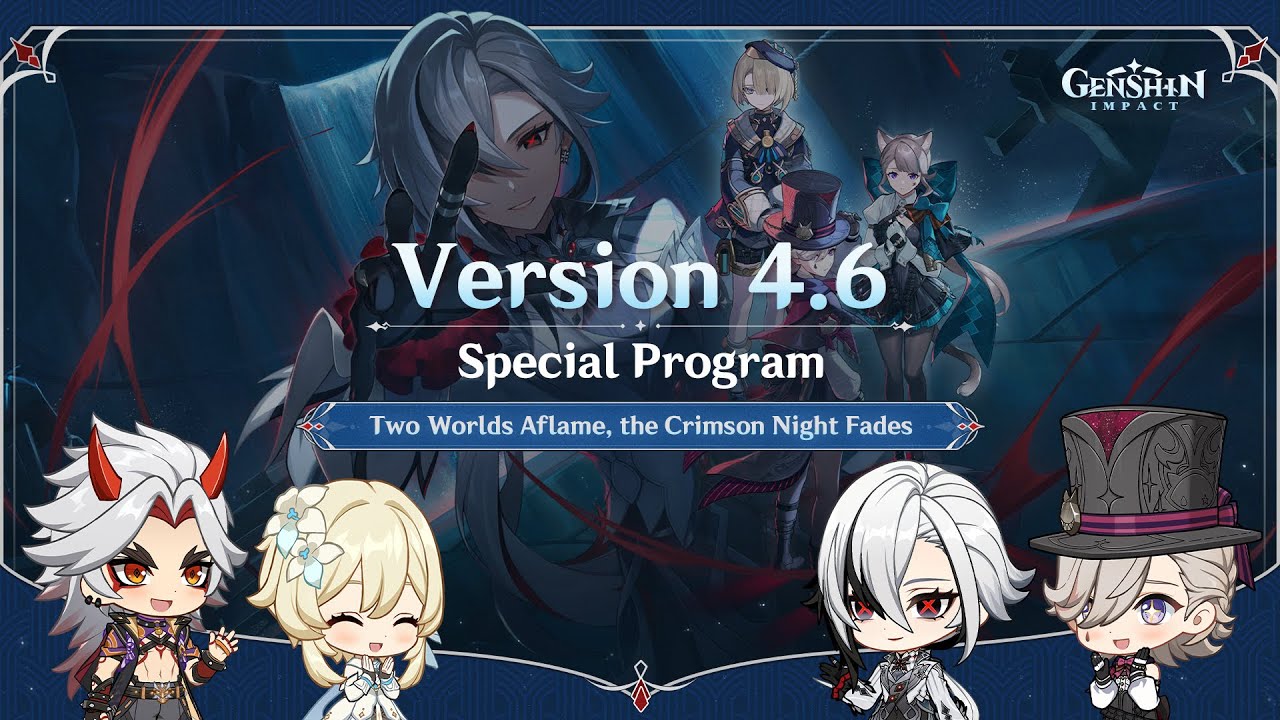
Genshin Impact Version 4.6 Special Program #NewVersion #SpecialProgram #GenshinImpact
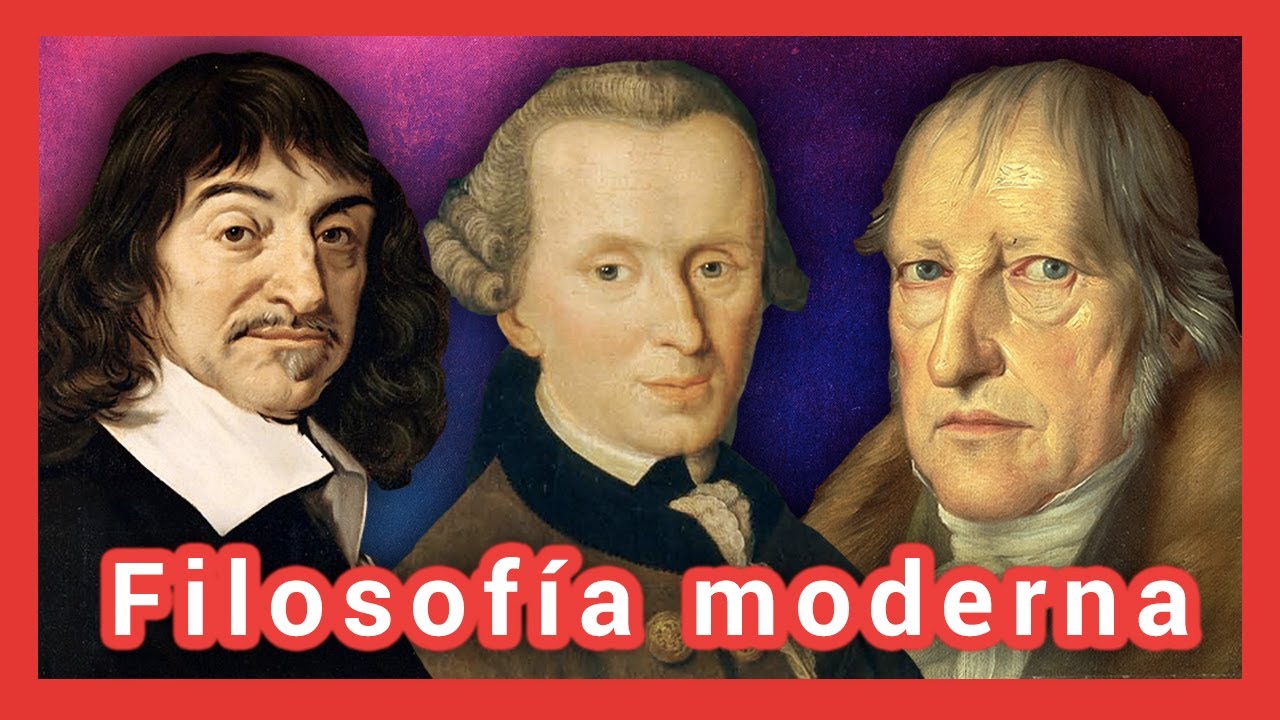
Introducción a la filosofía moderna | VII Filosofía moderna 01 | T07 E01
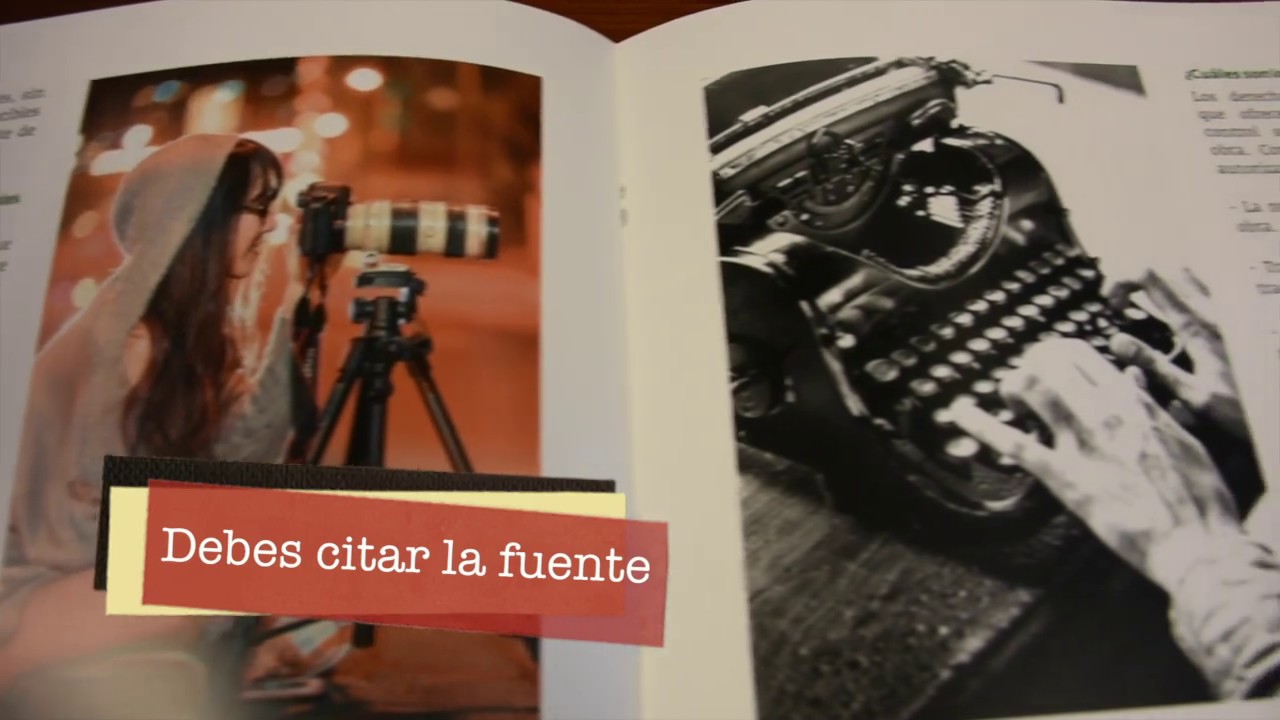
Tutorial para registro obras literarias