New OpenAI Model 'Imminent' and AI Stakes Get Raised (plus Med Gemini, GPT 2 Chatbot and Scale AI)
Summary
TLDRThe video script discusses recent developments in the field of AI, focusing on the anticipation of new OpenAI models, possibly named GPT 4.5, which are expected to be released soon. The discussion includes insights from company insiders and government officials, as well as the significance of two newly released papers totaling 90 pages. The script also explores the performance of AI models on benchmarks, particularly in mathematical reasoning, and the potential of Google's Med Gemini model in the medical field. Med Gemini is highlighted for its ability to provide medical answers competitive with doctors and assist in areas like surgery, showcasing the potential for AI to reduce medical errors and improve patient outcomes.
Takeaways
- 🤖 **Imminent AI Model Release**: Insiders suggest an imminent release of new OpenAI models, possibly named GPT 4.5, optimized for reasoning and planning.
- 🔍 **AI Safety and Oversight**: There is a lack of UK government safety testing for the latest AI models, despite promises from major companies like Meta and OpenAI.
- 📈 **Iterative Deployment**: OpenAI is likely to release an iterative model before GPT 5, focusing on gradual rollout and societal influence on AI systems.
- 🧠 **Generalization in Large Models**: Larger models tend to generalize better, even if they have seen questions in their training data, indicating a potential for 'Elementary reasoning ability'.
- 🚀 **Med Gemini's Advancements**: Google's Med Gemini models are competitive with doctors in providing medical answers, showcasing significant innovation in AI for healthcare.
- 🔋 **Energy and Compute Constraints**: The race for AI model development may soon face energy and data center constraints, affecting the continuous investment in new models.
- 📚 **Data Set Importance**: The performance of AI models is heavily influenced by the quality of their training data, suggesting that with enough compute power and a good data set, top performance can be achieved.
- 🧐 **Benchmark Contamination**: Some models may perform well on benchmarks due to having seen similar questions in their training data, which can skew performance metrics.
- 📉 **Model Limitations**: Despite advancements, there are still limitations in how much AI models can generalize, as seen in their performance on basic high school math questions.
- 🌐 **Web Search Integration**: Med Gemini uses web search integration to resolve uncertainty in answers, demonstrating the potential of combining AI with external data sources.
- ⚖️ **Ethical Deployment of AI in Medicine**: The development of AI in medical diagnosis raises ethical questions about the point at which it becomes necessary to deploy AI to assist clinicians to reduce medical errors.
Q & A
What is the significance of the rumored release of new OpenAI models?
-The rumored release of new OpenAI models is significant because it suggests advancements in AI technology that could potentially impact various sectors, including how AI systems are rolled out and their interaction with society.
Why did the author test the GPT2 chatbot instead of claiming that AGI has arrived?
-The author chose to test the GPT2 chatbot to provide a more measured and evidence-based response rather than making sensational claims about the arrival of Artificial General Intelligence (AGI) without proper evaluation.
What is the issue with the AI safety summit held in Bletchley?
-The issue is that major AI companies like Meta and OpenAI promised the UK government that they could safety test their latest models before release, but this hasn't been done, which raises concerns about the transparency and safety of AI model deployments.
Why is the author skeptical about the name 'GPT 5' for the next OpenAI model?
-The author is skeptical about the name 'GPT 5' because of hints and insider information suggesting that OpenAI might release an iterative model such as GPT 4.5 before a major release like GPT 5.
What does the author imply about the importance of data in AI model performance?
-The author implies that the quality and size of the dataset used for training are crucial for AI model performance, potentially allowing for 'Brute Force' performance improvements with enough computational power and a quality dataset.
What is the controversy surrounding the benchmark tests for AI models?
-The controversy is that some AI models may have been exposed to benchmark questions during their training, leading to artificially high performance results. This issue is known as 'contamination' and affects the reliability of benchmark tests.
How does the author describe the potential impact of Med Gemini on the medical field?
-The author describes Med Gemini as a potentially groundbreaking tool in the medical field, as it can provide medical answers competitive with doctors and assist in areas like surgery, which could significantly reduce medical errors and improve patient outcomes.
What is the main concern regarding the deployment of AI in sensitive areas like medicine?
-The main concern is the ethical and safety implications of deploying AI in medicine. There is a need to ensure that AI models are accurate and reliable enough to assist or potentially replace human clinicians in diagnosing diseases and assisting in procedures.
Why did the author find the performance of models like Claude 3 and Opus on basic high school questions surprising?
-The author found it surprising because these models can perform well on complex expert reasoning tasks, yet they struggle with basic high school-level questions, indicating a potential limit in their generalization capabilities.
What is the significance of the long context abilities of the Gemini 1.5 series of models?
-The significance is that these models can process and analyze extremely long documents, such as a 700,000-word electronic health record, which would be a daunting task for a human doctor, enhancing the potential utility of AI in medical diagnostics.
How does the author view the competition between Google and Microsoft in the medical AI field?
-The author views the competition positively, as it drives innovation and improvements in AI capabilities within the medical field, potentially leading to better patient outcomes and more efficient healthcare systems.
What is the author's stance on the deployment of AI models like Med Gemini in clinical settings?
-The author believes that once AI models like Med Gemini demonstrate unambiguous superiority in diagnosing diseases over human clinicians, it becomes unethical not to deploy them in assisting clinicians, considering the potential to save lives by reducing medical errors.
Outlines
🚀 Imminent Release of New OpenAI Models and AI Developments
The first paragraph discusses recent developments in AI, hinting at the imminent release of new models from OpenAI. It mentions an article from Politico about an AI safety summit where major AI companies like Meta and OpenAI promised the UK government early access to new models for safety testing. Insiders reveal that OpenAI is close to releasing a new model, possibly named GPT 4.5, optimized for reasoning and planning. The paragraph also references two papers that may be more significant than current rumors and discusses the testing of a mysterious GPT-2 chatbot that was showcased and then withdrawn.
🧐 Analysis of GPT-2 Chatbot Performance and Data's Role in AI
The second paragraph delves into the performance of the GPT-2 chatbot, which was tested by the author and compared to GPT 4 Turbo. It suggests that the data set used for training is crucial for AI performance, as highlighted by James Becker of OpenAI. The paragraph also discusses the importance of compute power and the potential for 'brute forcing' performance with sufficient resources. It touches on the supply constraints of GPUs and the recent release of a refined benchmark for testing mathematical reasoning capabilities of AI models, which revealed issues with data contamination and the generalization abilities of larger models.
🏥 Google's Med-Gemini: A Breakthrough in Medical AI Assistance
The third paragraph introduces Google's Med-Gemini, a significant advancement in medical AI that is competitive with doctors in providing medical answers. The paper outlines innovations such as inspecting model confidence, using search queries to resolve conflicts, and a fine-tuning loop. Med-Gemini has shown state-of-the-art performance in diagnosing diseases and has the potential to assist in surgery by analyzing video scenes in real-time. The paragraph also discusses the competitive nature of the field, with Google and Microsoft engaging in a positive rivalry to improve medical AI.
🤖 Ethical Considerations and Future Prospects of Medical AI
The fourth and final paragraph ponders the ethical implications of deploying AI in medicine, especially when it outperforms human clinicians in diagnostics. It raises the question of when it becomes unethical not to use AI in assisting clinicians, given its potential to reduce medical errors. The paragraph concludes by congratulating the team behind Med-Gemini and expressing optimism about the positive uses of AI, especially in contrast to other concerning autonomous AI deployments.
Mindmap
Keywords
💡AI safety
💡OpenAI models
💡GPT 4.5
💡Data set
💡Benchmarking
💡Medical AI
💡Contamination in benchmarks
💡Iterative deployment
💡Compute
💡Generalization in AI
💡Multimodal model
Highlights
Rumors suggest an imminent release of new OpenAI models, possibly named GPT 4.5, optimized for reasoning and planning.
Insiders reveal that only Google DeepMind has given the UK government early access to AI models, contrary to previous promises.
AI safety concerns are raised as the government has not yet safety-tested the latest models from major AI companies.
The performance of AI models on benchmarks may be influenced by the quality of their training data, as highlighted by a paper from Scale AI.
Large language models like GPT 4 and Claude demonstrate the ability to generalize and perform well on new, unseen questions.
Contamination of benchmark tests by models having seen the questions in their training data is a significant concern.
The paper suggests that larger models can learn elementary reasoning ability during training, even from contaminated data.
Google's Med Gemini model shows state-of-the-art performance in medical question answering, rivaling doctors' capabilities.
Innovations in Med Gemini include using search queries to resolve conflicts in model answers and fine-tuning models with correct answers.
Med Gemini's long context abilities allow it to process extensive medical records, which could greatly assist in diagnosis.
The model's performance on medical diagnosis is so advanced that it raises ethical questions about the deployment of AI in healthcare.
Google and Microsoft are in a competitive race to develop the most effective AI models for medical applications.
Med Gemini's multimodal capabilities enable it to analyze images and assist in surgeries, although it has not yet been deployed for ethical reasons.
The paper discusses the potential for improving Med Gemini by restricting its web searches to authoritative medical sources.
Despite its potential, Med Gemini is not open-sourced or widely available due to safety and commercial implications.
The development of AI models like Med Gemini represents a positive use of technology that could save lives by reducing medical errors.
The competition between tech giants to create better AI models for healthcare could lead to significant advancements in medical diagnostics and patient outcomes.
Transcripts
it has been a somewhat surreal few days
in AI for so many reasons and the month
of May promises to be yet stranger and
according to this under the radar
article company insiders and government
officials tell of an imminent release of
new openai models and yes of course the
strangeness at the end of April was
Amplified by the gpt2 chatbot a mystery
model showcased and then withdrawn
within days but which I did get to test
I thought testing it would be a slightly
more appropriate response than doing an
all cap video claiming that AGI has
arrived I also want to bring in two
papers released in the last 24 hours 90
pages in total and read in full they
might be more significant than any rumor
you have heard first things first though
that article from Politico that I
mentioned and the context to this
article is this there was an AI safety
Summit in Bletchley last year near to
where I live actually in Southern
England some of the biggest players in
AI like meta and open AI promised the UK
government that the UK government could
safety test the frontier latest models
before they were released there's only
one slight problem they haven't done it
now you might say that's just part of
the course for big Tech but the article
also revealed some interesting Insider
gossip Politico spoke to a host many
company insiders Consultants lobbyists
and government officials they spoke
anonymously over several months and not
only did we learn that it's only Google
deep mine that have given the government
Early Access we also learned that open
AI didn't now somewhat obviously that
tells us that they have a new model and
that it's very near to release now I
very much doubt they're going to call it
GPT 5 and you can see more of my reasons
for that in the video you can see on
screen but I think it's more likely to
be something like GPT 4.5 optimized for
reason Ing and planning now some of you
might be thinking is that all the
evidence you've got that a GPT 4.5 will
be coming before GPT 5 well not quite
how about this MIT technology review
interview conducted with samman in the
last few days in a private discussion
samman was asked if he knew when the
next version of GPT is slated to be
released and he said calmly yes now
think about it if the model had months
and months more of Uncertain safety
testing you couldn't be that confident
about a release date think about what
happened to Google Gemini Ultra which
was delayed and delayed and delayed that
again points to a more imminent release
then another bit of secondhand evidence
this time from an AI Insider on patreon
we have a wonderful Discord and this
Insider at a Stanford event put a
question directly to samman very
recently this was a different Stanford
event to the one I'm about to also quote
from and in this response Sam wman
confirmed that he's personally using the
unreleased version of their new model
but enough of secondhand sources what
about another direct quote from Sam
Alman well here's some more evidence
released yesterday that rather than drop
a bombshell GPT 5 on us which I predict
to come somewhere between November and
January they're going to give us an
iterative GPT 4.5 first he doesn't want
to surprise us it does kind of suck to
ship a product that you're embarrassed
about but it's much better than the
alternative and in this case in
particular where I think we really owe
it to society to deploy tively
one thing we've learned is that Ai and
surprise don't go well together people
don't want to be surprised people want a
gradual roll out and the ability to
influence these systems that's how we're
going to do it now he might want to tell
that to open ai's recent former head of
developer relations he now works at
Google and said something I really
appreciate about Google's culture is how
transparent things are 30 days in I feel
like I have a great understanding of
where we are going from a model
perspective having line of sight on this
makes it so much easier to start
building compelling developer products
it almost sounds like the workers at
open AI often don't have a great
understanding of where they're going
from a model perspective now In fairness
samman did say that the current GPT 4
will be significantly dumber than their
new model cha GPT is not phenomenal like
chpt is mildly embarrassing at best GPT
4 is the dumbest model any of you will
ever ever have to use again by a lot but
you know it's like important to ship
early and often and we believe in
iterative deployment so agency and
reasoning focused GPT 4.5 coming soon
but GPT 5 not until the end of the year
or early next those are my predictions
now some people were saying that the
mystery gpt2 chatbot could be GPT 4.5 it
was released on a site used to compare
the different outputs of language models
and look here is it creating a beautiful
unicorn which llama 3 couldn't do now I
Fran Bally got ready a tweet saying that
super intelligence had arrived but
quickly had to delete it and not just
because other people were reporting that
they couldn't get decent unicorns and
not just because that exact unicorn
could be found on the web but the main
reason was that I was one of the lucky
ones to get in and test gpt2 chat B on
the arena before it was withdrawn I
could only do eight questions but I gave
it my standard handcrafted so not on the
web set of test questions spanning logic
theory of mind mathematics coding and
more its performance was pretty much
identical to GPT 4 Turbo there was one
question that it would get right more
often than GPT 4 Turbo but that could
have been noise so if this was a sneak
preview of GPT 4.5 I don't think it's
going to shock and stun the entire
industry so tempting as it was to bang
out a video saying that AI has arrived
in all caps I resisted the urge since
then other testers have found broadly
the same thing on language translation
the mystery gpt2 chatbot massively
underperforms Claude Opus and still
underperforms gp4 turbo on an extended
test of logic it does about the same as
Opus and gp4 turbo of course that still
does leave the possibility that it is an
open AI model a tiny one and one that
they might even release open weights
meaning anyone can use it and in that
case the impressive thing would be how
well it's performing despite it size
well if gpt2 chatbot is a smaller model
how could it possibly be even vaguely
competitive the secret source is the
data as James Becker of open AI said
it's not so much about tweaking model
configurations and hyperparameters nor
is it really about architecture or
Optimizer choices behavior is determined
by your data set it is the data set that
you are approximating to an incredible
degree in a later post he referred to
the flaws of Dary 3 and gp4 and also
flaws in video probably referring to the
at the time unreleased Sora and said
they arise from a lack of data in a
specific domain and in a more recent
post he said that while computer
efficiency was still super important
anything can be stay of the-art with
enough scale compute and eval hacking
now we'll get to evaluation and
Benchmark hacking in just a moment but
it does seem to me that there are more
and more hints that you can Brute Force
performance with enough compute and and
as mentioned a quality data set at least
to me it seems increasingly clear that
you can pay your way to top performance
unless open AI reveal something
genuinely shocking the performance of
meta's llama 3 8 billion 70 billion and
soon 400 billion show that they have
less of a secret Source than many people
had thought and as Mark Zuckerberg
hinted recently it could just come down
to which company blinks first who among
Google meta and Microsoft which provides
the compute for open AI is willing to
continue to spend tens or hundreds of
billions of dollars on new models if the
secret is simply the data set that would
make less and less sense you over the
last few years I think there was this
issue of um GPU production yeah right so
even companies that had the money to pay
for the gpus couldn't necessarily get as
many as they wanted because there was
there were all these Supply constraints
now I think that's sort of getting less
so now I think you're seeing a bunch of
companies think about wow we should just
like really invest a lot of money in
building out these things and I think
that will go for um for some period of
time there is a capital question of like
okay at what point does it stop being
worth it to put the capital in but I
actually think before we hit that you're
going to run into energy constraints now
if you're curious about energy and data
center constraints check out my why does
open aai need a Stargate supercomputer
video released 4 weeks ago but before we
leave data centers and data set I must
draw your attention to this paper
released in the last 24 hours it's
actually a brilliant paper from scale AI
what they did was create a new and
refined version of a benchmark that's
used all the time to test the
mathematical reasoning capabilities of
AI models and there were at least four
fascinating findings relevant to all new
models coming out this year the first
the context and they worried that many
of the latest models had seen the
Benchmark questions in their training
data that's called contamination because
of course it contaminates the results on
the test the original test had 8,000
questions but what they did was create a
thousand new questions of similar
difficulty now if contamination wasn't a
problem then models should perform just
as well with the new questions as with
the old and obviously that didn't happen
for the mistol and fi family of models
performance notably lagged on the new
test compared to the old one whereas
fair's fair for GPT 4 and Claude
performance was the same or better on
the new fresh test but here's the thing
the authors figured out that that wasn't
just about Which models had seen the
questions in their training data they
say that mistol large which performed
exactly the same was just as likely to
have seen those questions as Mixr
instruct which way underperformed so
what could explain the difference well
the bigger models generalize even if
they have seen the questions they learn
more from them and can generalize to new
questions and here's another supporting
quote they lean toward the hypo
hypthesis that sufficiently strong large
language models learn Elementary
reasoning ability during training you
could almost say that benchmarks get
more reliable when you're talking about
the very biggest models next and this
seems to be a running theme in popular
ml benchmarks the GSM 8K designed for
high schoolers has a few errors they
didn't say how many but the answers were
supposed to be positive integers and
they weren't the new Benchmark however
passed through three layers of quality
checks third they provide extra theories
as to why models might overperform on
benchmarks compared to the real world
that's not just about data contamination
it could be that model Builders designed
data sets that are similar to test
questions after all if you were trying
to bake in reasoning to your model what
kind of data would you collect plenty of
exams and textbooks so the more similar
their data set is in nature not just
exact match to benchmarks the more your
benchmark performance will be elevated
compared to simple real world use think
about it it could be an inadvertent
thing where enhancing the overall
smartness of the model comes at the cost
of overperforming on benchmarks and
whatever you think about benchmarks that
does seem to work here Sebastian bck
lead author of The Five series of models
I've interviewed him for AI insiders and
he said this even on those 1,000 neverbe
seen questions 53 mini which is only 3.8
billion parameters performed within
about 8 or 9% of GP GT4 turbo now we
don't know the parameter count of GT4
turbo but it's almost certainly orders
of magnitude bigger so training on high
quality data as we have seen definitely
works even if it slightly skews
Benchmark performance but one final
observation from me about this paper I
read almost all the examples that the
paper gave from this new Benchmark and
as the paper mentions they involve basic
addition subtraction multiplication and
division after all the original test was
designed for youngsters you can pause
and try the questions yourself but
despite them being lots of words they
aren't actually hard at all so my
question is this why are models like
Claude 3 Opus still getting any of these
questions wrong remember they're scoring
around 60% in graduate level expert
reasoning the GP QA if Claude 3 Opus for
example can get questions right that
phds struggle to get right with Google
and 30 minutes why on Earth with five
short examples can they not get these
basic high school questions right either
there are still flaws in the test or
these models do have a limit in terms of
how much they can generalize now if you
like this kind of analysis feel free to
sign up to my completely free newsletter
it's called signal to noise and the link
is in the description and if you want to
chat in person about it the regional
networking on the AI insiders Discord
server is popping off there are meetings
being arranged not only in London but
Germany the Midwest Ireland San
Francisco Madrid Brazil and it goes on
and on honestly I've been surprised and
honored by the number of spontaneous
meetings being arranged across the world
but it's time arguably for the most
exciting development of the week Med
Gemini from Google it's a 58 page paper
but the tldr is this the latest series
of Gemini models from Google are more
than competitive with doctors at
providing medical answers and even in
areas where they can't quite perform
like in surgery they can be amazing
assistant in a world in which millions
of people die due to Medical errors this
could be a tremendous breakthrough Med
Gemini contains a number of Innovations
it wasn't just rerunning the same test
on a new model for example you can
inspect how confident a model is in its
answer by trolling through the raw
outputs of a model called the logic you
could see how high probability they find
their answers if they gave confident
answers you would submit that as the
answer they use this technique by the
way for the original Gemini launch where
they claimed to be GPT 4 but that's
another story anyway if the model is not
confident you can get the model to
generate search queries to resolve those
conflicts train it in other words to use
Google seems appropriate then you can
feed that additional context provided by
the web back into the model to see if
it's confident now but that was just one
Innovation what about this fine-tuning
Loop to oversimplify they get the model
to Output answers again using the help
of search and then the outputs that had
correct answers were used to fine-tune
the models now that's not perfect of
course because sometimes you can get the
right answer with the wrong logic but it
worked up to a certain point at least
just last week by the way on patreon I
described how this reinforced in context
learning can be applied to multiple
domains other Innovations come from the
incredible long context abilities of the
Gemini 1.5 series of models with that
family of models you can troll through a
700,000 word electronic health record
now imagine a human doctor trying to do
the same thing I remember on the night
of Gemini 1.5s release calling it the
biggest news of the day even more
significant than Sora and I still stand
by that so what were the results well of
course a state of-the-art performance on
Med QA that assesses your ability to
diagnose diseases the doctor pass rate
by the way is around 60% and how about
this for a mini theme of the video when
they carefully analyze the questions in
the Benchmark they found that 7.4% of
the questions have quality issues things
like lacking key information incorrect
ansers or multiple plausible
interpretations so just in this video
alone we've seen multiple Benchmark
issues and I collected a thread of other
Benchmark issues on Twitter the positive
news though is just how good these
models are getting at things like
medical note summarization and clinical
referral letter generation but I don't
want to detract from the headline which
is just how good these models are
getting at diagnosis here you can see
Med Gemini with search way outperforming
expert clinicians with search by the way
when errors from the test were taken out
performance bumped up to around 93% and
the authors can't wait to augment their
models with additional data things like
data from consumer wearables genomic
information nutritional data and
environmental factors and as a quite
amusing aside it seems like Google and
Microsoft are in a tussle to throw shade
at each other's methods in a positive
spirit Google contrast their approach to
Med prompt from Microsoft saying that
their approach is principled and it can
be easily extended to more complex
scenarios Beyond Med QA now you might
say that's harsh but Microsoft earlier
had said that their Med prompt approach
shows GPC 4's ability to outperform
Google's model that was fine-tuned
specifically for medical applications it
outperforms on the same benchmarks by a
significant margin well Google have
obviously won up them by reach new
state-of-the-art performances on 10 of
14 benchmarks Microsoft had also said
that their approach has simple prompting
and doesn't need more sophisticated and
expensive methods Google shot back
saying they don't need complex
specialized prompting and their approach
is best honestly this is competition
that I would encourage may they long
compete for Glory in this medical arena
in case you're wondering because Gemini
is a multimodal model it can see images
too you can interact with patients and
ask them to to provide images the model
can also interact with primary care
physicians and ask for things like
X-rays and most surprisingly to me it
can also interact with surgeons to help
boost performance yes that's video
assistance during live surgery of course
they haven't yet deployed this for
ethical and safety reasons but Gemini is
already capable of assessing a video
scene and helping with surgery for
example by answering whether the
critical view of safety criteria are
being met Do you have a great view of
the gallbladder for example and Med
Gemini could potentially guide surgeons
in real time during these complex
procedures for not only improved
accuracy but patient outcomes notice the
Nuance of the response from Gemini oh
the lower third of the gallbladder is
not dissected off the cystic plate and
the authors list many improvements that
they could have made they just didn't
have time to make for example the models
were searching the Wild web and one
option would be to restrict the search
results to just more authoritative
medical sources the catch though is that
this model is not open-sourced and isn't
widely available due to they say the
safety implications of unmonitored use I
suspect the commercial implications of
open sourcing Gemini also had something
to do with it but here's the question I
would set for you we know that hundreds
of thousands or even millions of people
die due to Medical mistakes around the
world so if and when Med Gemini 2 3 or 4
becomes unambiguously better than all
clinicians at diagnosing diseases then
at what point is it unethical not to at
least deploy them in assisting
clinicians that's definitely something
at least to think about overall this is
exciting and excellent work so many
congratulations to the team and in a
world that is seeing some Stark misuses
of AI as well as increasingly autonomous
deployment of AI like this autonomous
tank the fact that we can get
breakthroughs like this is genuinely
uplifting thank you so much for watching
to the end and have a wonderful day
5.0 / 5 (0 votes)
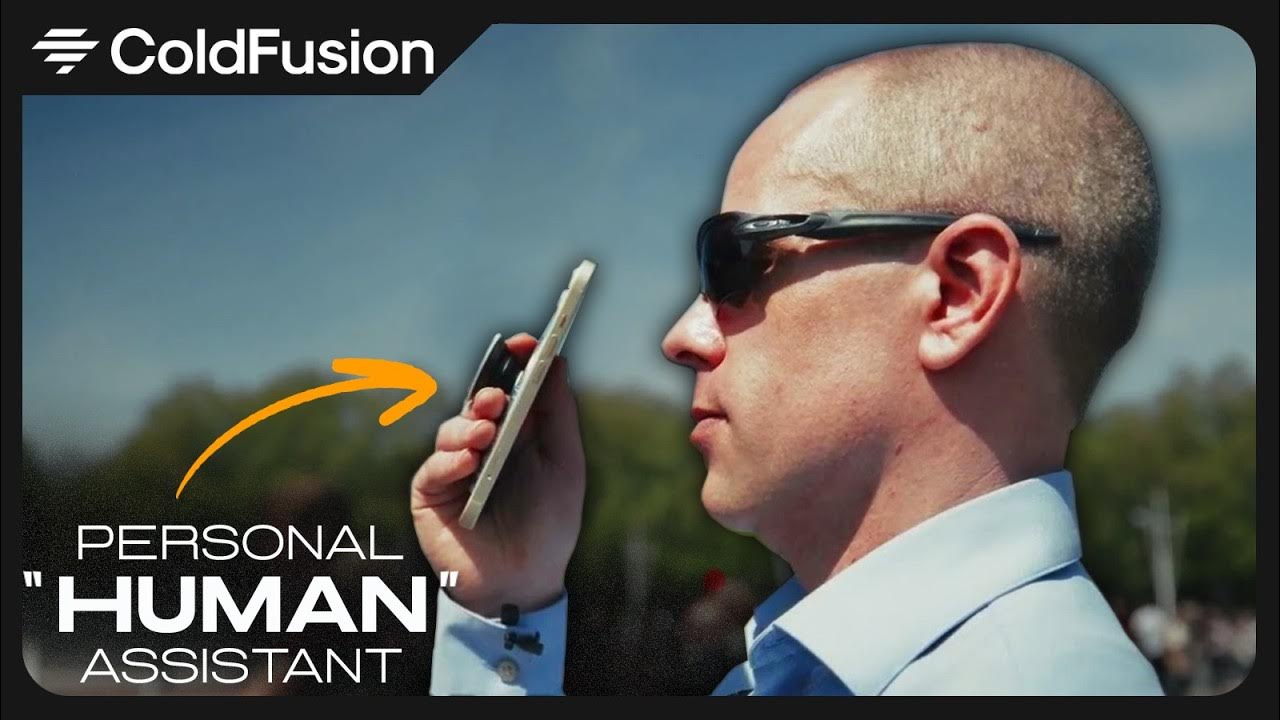
ChatGPT Can Now Talk Like a Human [Latest Updates]
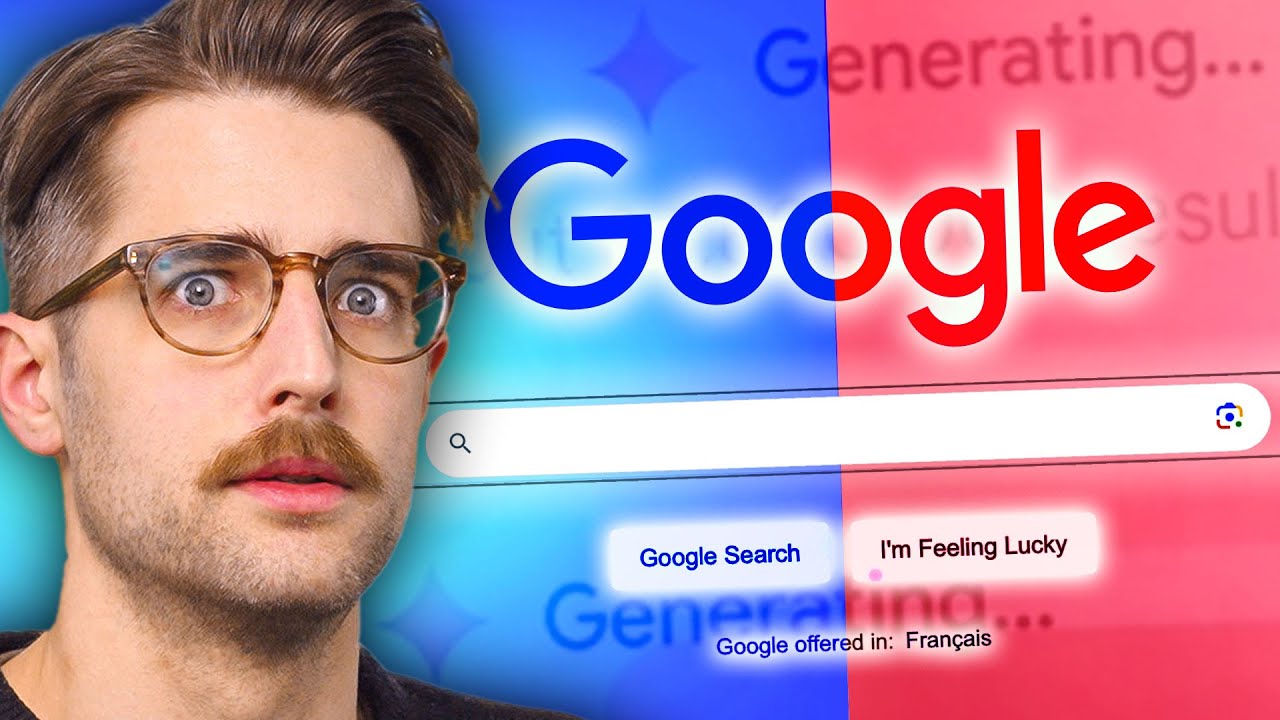
Search is About to Change Forever
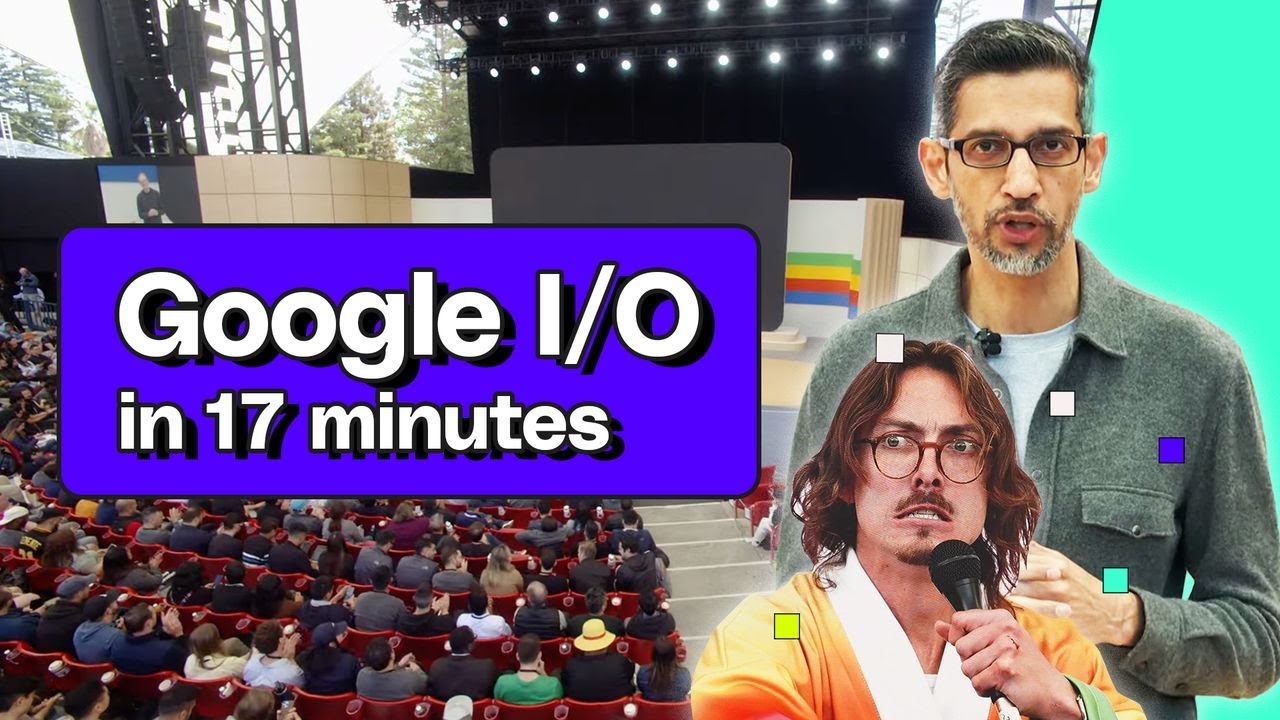
Google I/O 2024 keynote in 17 minutes
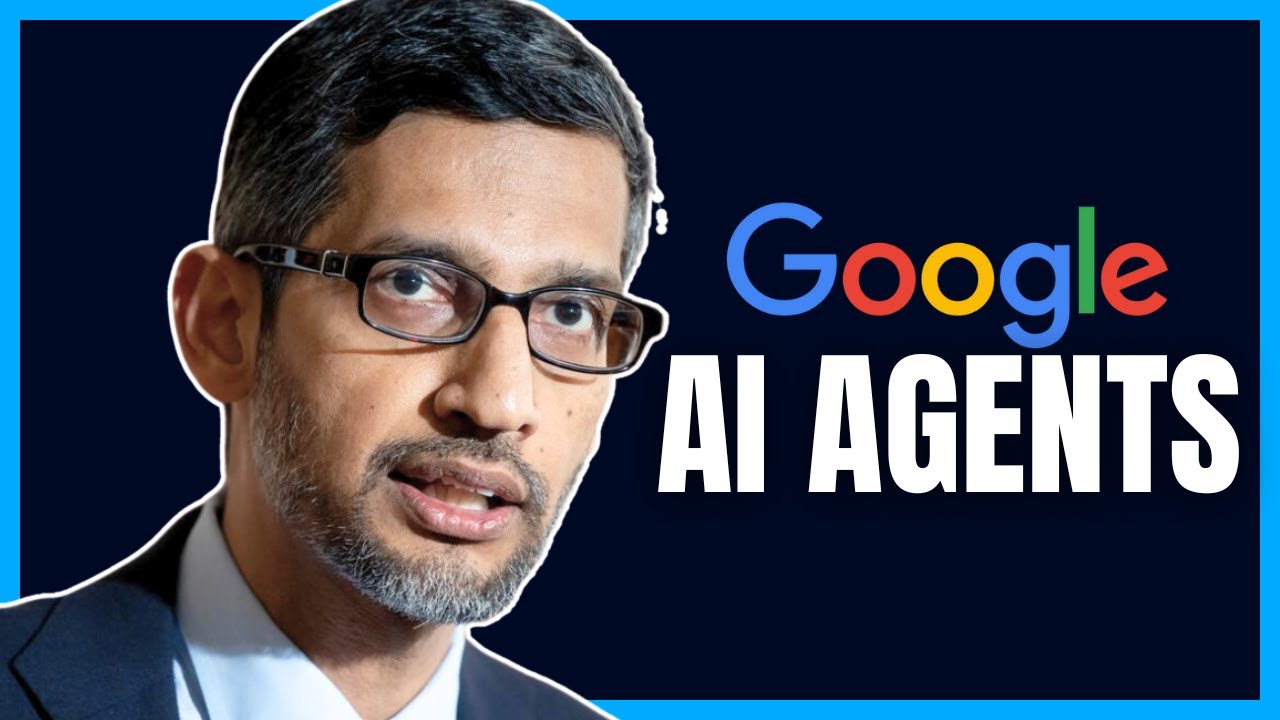
Google Releases AI AGENT BUILDER! 🤖 Worth The Wait?
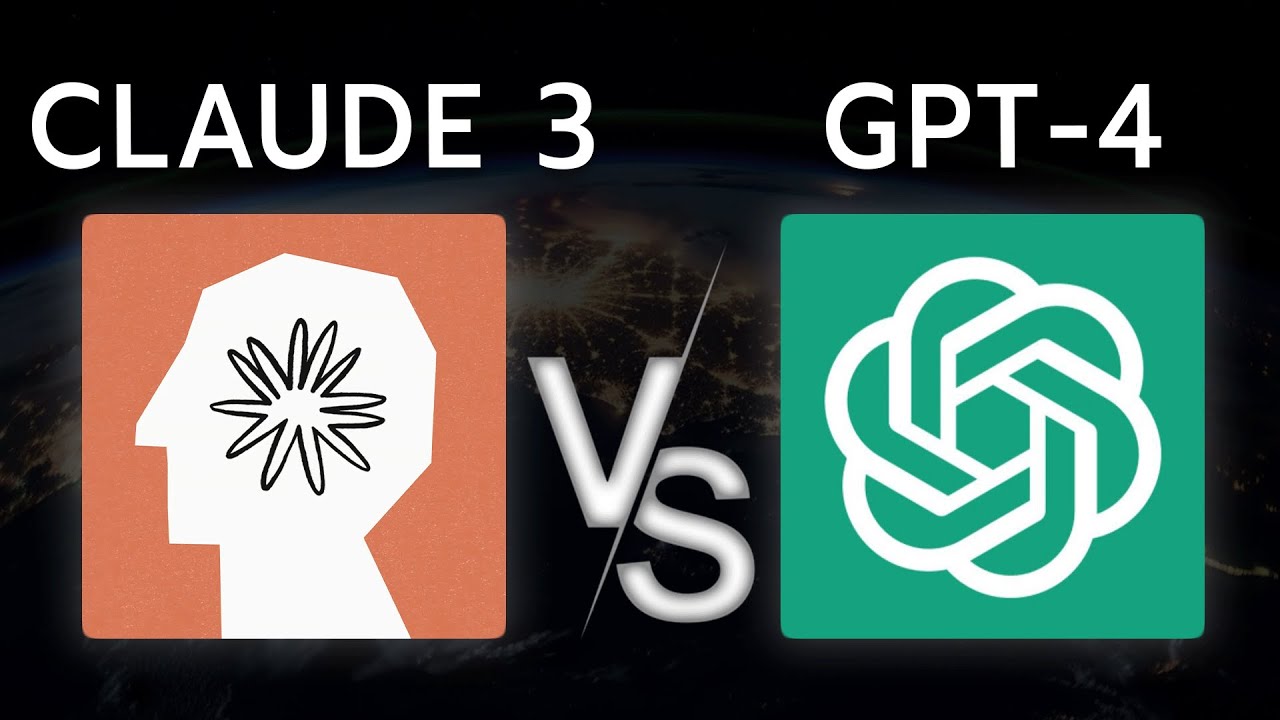
Why & When You Should Use Claude 3 Over ChatGPT
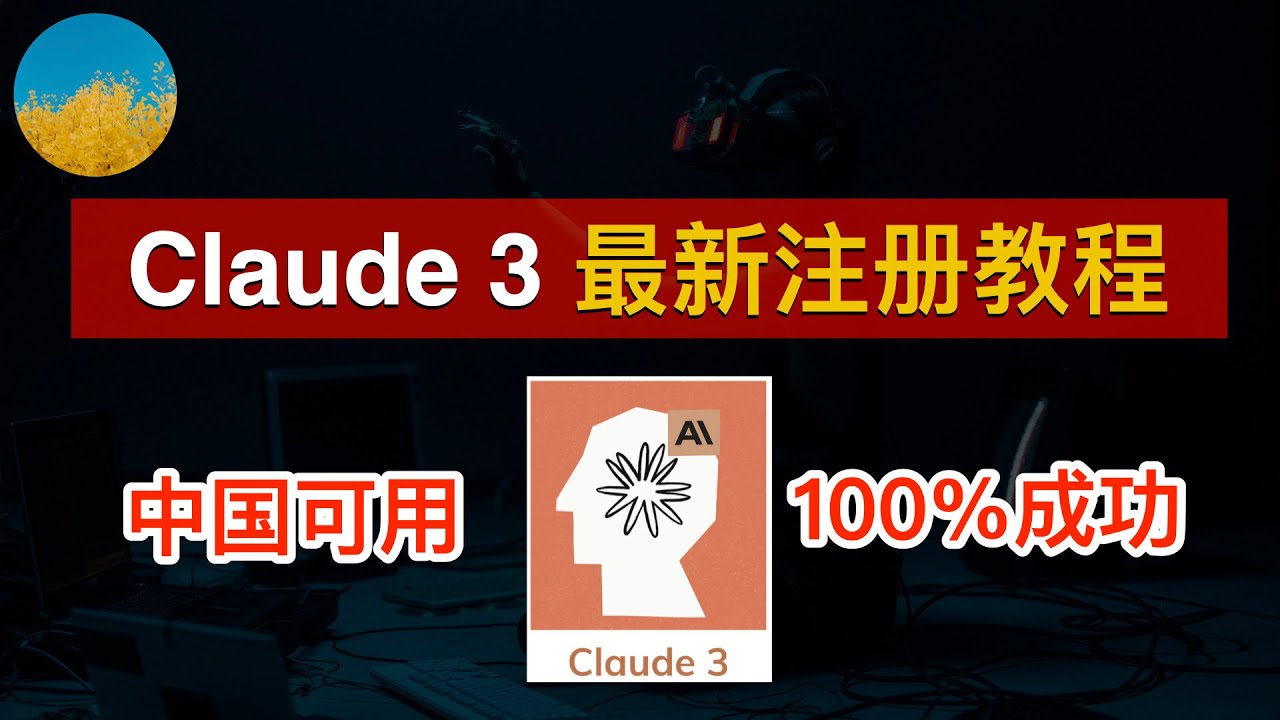
🎉【2024最新】Claude 3怎么注册?注册Claude 3一次成功、Claude AI最新注册教程!全面超越GPT-4、Gemini Ultra的Claude 3 AI怎么用 | 数字牧民LC