WHISTLEBLOWER Reveals Complete AGI TIMELINE, 2024 - 2027 (Q*, QSTAR)
Summary
TLDR这份视频脚本披露了一份据称来自OpenAI内部的机密文件,暗示OpenAI计划在2027年之前实现人工通用智能(AGI)。该文件详细阐述了OpenAI正在训练一个125万亿参数的多模态模型,预计将在2023年12月完成训练。尽管文件中有一些猜测成分,但它提供了相当多的证据和细节,令人怀疑OpenAI可能真的在秘密推进AGI的工作。这份视频探讨了该文件所引发的一系列问题和猜测。
Takeaways
- 😃 视频指出 OpenAI 有一份秘密计划,计划在 2027 年之前实现 AGI(通用人工智能)。
- 🤔 这份文件声称 OpenAI 在 2022 年 8 月开始培训了一个 1.25 万亿参数的多模态模型,并在 2023 年 12 月完成训练。
- 🔍 文件显示 OpenAI 一直在努力创建一个人脑大小(1千万亿参数)的 AI 模型,这是他们实现 AGI 的计划。
- 📈 根据 Chinchilla 定律,即使 1 千万亿参数的模型性能略低于人类,如果使用大量数据进行训练,它就能超过人类水平。
- 😲 一些 AI 领袖如 Hinton 和 Hassabis 近期发出警告,表示 AGI 的到来比预期的要快。
- 🕵️ 微软对 OpenAI 的 100 亿美元投资有望为 OpenAI 提供训练 AGI 系统所需的计算资源。
- 💰 Sam Altman 正在筹集 70 亿美元,可能是为了训练大规模 AGI 系统所需的巨额计算成本。
- ⚠️ 有声音呼吁暂停训练超过 GPT-4 水平的 AI 系统,包括正在训练中的 GPT-5。
- 🔐 OpenAI 计划在 2027 年之前解决"超级对齐"问题,以确保安全释放 AGI。
- 🔜 视频暗示,每年都会有新的GPT模型发布,GPT-7 之后可能就是 AGI 系统。
Q & A
OpenAI计划到2027年创造AGI的文件揭示了哪些关键信息?
-该文件透露,OpenAI从2022年8月开始训练一个具有125万亿参数的多模态模型,首个阶段命名为Q星,模型在2023年12月训练完成,但由于高昂的推理成本,发布被取消。文件还暗示,OpenAI的长期计划是通过逐年发布新模型,最终在2027年达到AGI。
文档中提到的“Q星”、“ARUS”和“GOI”是什么?
-Q星、ARUS和GOI被提及为OpenAI开发的模型名称。其中,ARUS模型的开发被取消,因为它运行效率不高。这些名称被视为OpenAI内部计划和模型的一部分,指示了公司在人工智能领域的研究方向和进展。
为什么OpenAI会将原本计划的GPT-5模型取消或重命名?
-原本计划的GPT-5模型被取消或重命名的具体原因在文档中没有详细说明,但暗示这与模型开发过程中的策略调整和技术挑战有关,可能是由于在推理成本、性能预期或技术突破方面遇到的问题。
Elon Musk对OpenAI的计划提出诉讼有何影响?
-Elon Musk提起的诉讼导致了对OpenAI计划的一些延迟,特别是影响了原计划中的GPT-6和GPT-7的开发和发布。Musk的诉讼主要是基于对OpenAI远离其开源目标和创造高级技术应对公众开放的承诺的担忧。
什么是AGI,以及它与当前AI技术的区别是什么?
-AGI(人工通用智能)是指能够执行任何智力任务的人工智能,与人类智力水平相当。它与当前的AI技术(通常专注于特定任务的解决方案)的主要区别在于其通用性和灵活性,AGI能够在没有特定培训的情况下理解和执行各种复杂任务。
文档如何定义人类水平的AGI,以及它的实现对社会有什么潜在影响?
-文档定义人类水平的AGI为能够执行任何一个聪明人类能够完成的智力任务的人工智能。它的实现可能会彻底改变社会,包括经济、就业、教育和技术发展等方面,同时也引发了对安全、伦理和社会影响的广泛关注。
为什么说模型参数数量是预测AI性能的关键指标?
-模型参数数量被视为预测AI性能的关键指标,因为参数越多,模型处理和理解复杂数据的能力通常越强。参数数量与生物大脑中的突触数目相类比,用来估计AI模型的复杂度和潜在的智能水平。
OpenAI如何通过参数计数和数据量来逼近人类大脑的性能?
-OpenAI通过增加模型的参数计数并训练它们使用大量的数据来逼近人类大脑的性能。通过模仿人脑中神经元间突触的连接方式,以及利用海量数据进行训练,OpenAI旨在创造出能够模拟人类认知过程的AI模型。
文档中提到的“Chinchilla法则”是什么,它如何影响AI模型的训练?
-Chinchilla法则基于DeepMind的研究,指出当前模型训练方式在数据使用方面存在低效,通过使用更多的数据进行训练,可以显著提高AI模型的性能。这一发现促使OpenAI和其他AI研究机构重新评估其训练策略,使用更多数据以期望达到更优的训练效果。
为什么说AI领域的研究是动态且迅速发展的?
-AI领域的研究被认为是动态且迅速发展的,因为每天都有大量的新研究和技术突破被发布,不断推动了AI技术的极限,并改变了我们对可能实现的智能水平的理解。这一领域的快速进步要求研究者、开发者和利益相关者持续关注最新动态,以适应不断变化的技术环境。
Outlines
📄 开篇介绍
视频开始讨论一个揭示OpenAI到2027年创建人工通用智能(AGI)计划的文档。强调了该文档包含许多推测和不完全是事实,但提到了一些关键内容,比如OpenAI于2022年8月开始训练一个125万亿参数的多模态模型,以及由于高昂的推理成本而取消了其发布。视频作者提醒观众以怀疑的态度看待这些信息,同时强调了对OpenAI未来计划的兴趣。
🔍 文档深入解析
深入分析了文档中提及的OpenAI的计划,包括关于GPT 4.5被重命名为GPT 5,以及GPT 5原定于2025年发布但被取消的信息。讨论了一些被提及的模型,如arus和GOI,及其与OpenAI相关文章的联系。同时,文档提及了GPT 6(现称GPT 7)的发布被埃隆·马斯克的诉讼所延迟,以及关于OpenAI的公开资源目标的讨论。
🚀 向AGI迈进
该段落讨论了100万亿参数模型的潜力以及通过参数数量预测AI性能的可行性。提到了一个重要的研究,展示了随着参数数量的增加,AI在不同任务上的表现也随之提升,尽管收益递减。这段落也提到了如何通过增加数据量来弥补100万亿参数模型在性能上的不足,以及OpenAI使用的工程技术来桥接这一差距。
🧠 关于模型的进一步洞察
深入探讨了OpenAI对GPT 4模型的开发计划,包括关于100万亿参数模型的误解和澄清。提到了OpenAI利用更少的模型参数实现更高性能的方法,以及在网络上训练模型以处理更复杂问题的挑战。还讨论了关于GPT 4的早期泄露信息,以及OpenAI如何回应这些泄露信息。
🔧 模型测试与预期
讨论了GPT 4在2022年10月和11月被测试的信息,以及这些测试与先前泄露的一致性。文档还揭示了OpenAI官方对于100万亿参数GPT 4模型的立场,以及关于模型视频处理能力的泄露信息。这段落展示了关于AI性能的预期,尤其是与视频生成相关的能力。
🤖 机器人学与未来展望
这一部分讨论了将AI应用于机器人技术的潜力,尤其是关于视觉数据的重要性和通过大规模模型训练实现复杂任务的可能性。提及了特斯拉在其自动驾驶技术中完全依赖视觉数据的决定,以及通过视觉和语言数据训练的大模型如何处理机器人任务。这强调了通过训练更大模型来实现更高级别的AI性能的趋势。
🌐 AGI和超级对齐的道路
分析了OpenAI对于创建AGI的长期计划和目标,特别是关于通过提高模型的参数数量和训练数据量来实现更接近人类水平的AI性能。这部分还涉及了关于AI研究方向和超级对齐(super alignment)问题的深入讨论,以及知名AI研究人员对于AI发展速度和潜在风险的担忧。
💡 综合理解与未来展望
最后一段深入探讨了OpenAI在创建AGI方面的总体战略和计划,包括预计每年发布一个新的GPT模型,直到2027年。讨论了OpenAI对超级对齐技术的期望,以及如何通过逐步发布更新和更强大的模型来为社会和技术环境提供适应AGI的时间。同时,也反思了关于AGI发展的不确定性和可能的未来趋势。
Mindmap
Keywords
💡人工通用智能(AGI)
💡GPT(生成式预训练转换器)
💡参数量
💡计算资源
💡数据
💡多模态
💡超级对齐(Superintelligence Alignment)
💡泄露(Leak)
💡缩放定律(Scaling Laws)
💡风险意识
Highlights
There was a recent document that apparently reveals OpenAI's secret plan to create AGI by 2027.
The document states that OpenAI started training a 125 trillion parameter multimodal model called 'qstar' in August 2022, which finished training in December 2023 but the launch was cancelled due to high inference cost.
Multiple AI researchers and entrepreneurs claim to have had inside information about OpenAI training models with over 100 trillion parameters, intended for AGI.
OpenAI's president Greg Brockman stated in 2019 that their plan was to build a human brain sized model within 5 years to achieve AGI.
AI leaders like Demis Hassabis and Geoffrey Hinton have recently expressed growing concerns about the potential risks of advanced AI capabilities.
After the release of GPT-4, the Future of Life Institute released an open letter calling for a 6-month pause on training systems more powerful than GPT-4, including the planned GPT-5.
Sam Altman confidently stated that there is enough data on the internet to create AGI.
OpenAI realized their initial scaling laws were flawed and have adjusted to take into account DeepMind's 'Chinchilla' laws, which show vastly more data can lead to massive performance boosts.
While a 100 trillion parameter model may be slightly suboptimal, OpenAI plans to use the Chinchilla scaling laws and train on vastly more data to exceed human-level performance.
Microsoft invested $10 billion into OpenAI in early 2023, providing funds to train a compute-optimal 100 trillion parameter model.
An OpenAI researcher's note reveals they were working on preventing an AI system called 'qstar' from potentially destructive outcomes.
The document theorizes that OpenAI plans to release a new model each year until 2027, aligning with their 4-year timeline to solve the 'super alignment' problem for safe AGI release.
GPT-7 is speculated to be the last pre-AGI model before GPT-8 achieves full AGI capability in 2027.
Sam Altman mentioned OpenAI can accurately predict model capabilities by training less compute-intensive systems, potentially forecasting the path to AGI.
Altman is reportedly trying to raise $7 trillion, likely to fund the immense compute required for training a brain-scale AGI model using the Chinchilla scaling laws.
Transcripts
so there was a recent document that
actually apparently reveals open ai's
secret plan to create AGI by 2027 now
I'm going to go through this document
with you Page by Page I've read it over
twice and there are some key things that
actually did stand out to me so without
further Ado let's not waste any time and
of course just before we get into this
this is of course going to contain a lot
of speculation remember that this
document isn't completely 100% factual
so just take this video with a huge of
salt so you can see here the document
essentially says revealing open ai's
plan to create AGI by 2027 and that is a
rather important date which we will come
back to if we look at this first thing
you can see there's an introduction okay
and of course remember like I said there
is a lot of speculation in this document
there are a lot of different facts and
of course like I said anyone can write
any document and submit it to um you
know Twitter or Reddit or anything but I
think this document does contain a
little bit more than that so it starts
out by stating that in this document I
will be revealing information I have
gathered regarding opening eyes delayed
plans to create human level AGI by 2027
not all of it will be easily verifiable
but hopefully there's enough evidence to
convince you summary is basically that
openai has started training a 125
trillion parameter multimodal model in
August of 2022 and the first stage was a
rakus also called qstar and the model
finished training in December of 2023
but the launch was cancelled due to the
high inference cost
and before you guys think it's just
document with like just words I'm going
to show you guys later on like all of
the crazy kind of stuff that is kind of
verifiable that does actually um line up
with some of the stuff that I've seen as
someone that's been paying attention to
this stuff so this is literally just the
introduction um the juicier stuff does
come later but essentially they actually
talk about the and this is just like an
overview so you're going to want to
continue watching they essentially state
that you know this is the original GPT 5
which was planned for release in 2025
bobi GPT 4.5 has been renamed name to
gbt 5 because the original gbt 5 has
been cancelled now I got to be honest
this paragraph here is a little bit
confusing um but I do want to say that
the words arus and the words GOI are
definitely models that were referred to
by several articles that were referring
to leaks from open eye and I think they
were actually on the information so this
is some kind of stuff that I didn't
really hear that much about but the
stuff that I did hear was pretty crazy
so um this arus and this goby thing
although you might not have heard a lot
about it of course it is like like a
kind of like half and half leak but like
I was saying this stuff is kind of true
so you can see here open AI dropped work
on a new arus model in rare AI setback
and this one actually just talks about
um how by the middle of open AI you know
scrapping an araus launch after it
didn't run as efficiently so there's
actually some references to this but a
lot of the stuff is a little bit
confusing but we're going to get on to
the main part of this story now I just
wanted to include that just to show you
that you know these names aren't made up
because if I was watching this video for
the first time and I hadn't seen some of
the prior articles before I'd be
thinking what on Earth is a r what on
Earth is GOI I've only heard about qar
so essentially let's just take a look
and it says the next stage of qstar
originally GPT 6 but since renamed
gpt7 originally for release in 2026 has
been put on hold because of the recent
lawsuit by Elon Musk if you haven't been
paying attention to the space
essentially Elon Musk just f a lawsuit
released a video yesterday um stating
that open ey have strayed far too long
from their goals and if they are
creating some really advanced technology
the public do deserve to have it open
source because that was their goal um
and essentially you can see here it says
qar GBC planned to be released in 2027
achieving full AGI and one thing that I
do want to say about this because
essentially they're stating that you
know they're doing this up to gpt7 and
then after gpt7 they do get to AGI one
thing that I do think okay and I'm going
to come back to this as well is that the
dates kind of do line up and I say kind
of because not like 100% because we
don't know but presuming let's just
presume okay because GPT 4 was released
in 2023 right um let's just say you know
every year release a new model okay um
that would mean that you know in 2024 we
would get gbt 5 in 2025 we get GPT 6 in
2026 we would get GPT 7 and in 2027 we
would get GPT 8 which is of course AGI
now one thing I do think about this that
is kind of interesting and remember I'm
going to come back to this so pay
attention Okay what I'm basically saying
is that if openi are consistent with
their year releases so for example if
they are going to release a new model
every year and if we continue at the
same rate like a new GPC every single
year which is possible him stating that
gp7 being the last release before GPT 8
which is Agi does actually kind of make
sense because once again and I know you
guys are going to hate this but if we
look at the trademarks okay remember
that they trademarked this around the
same time okay around that 2023 time
when all of this crazy stuff was going
on and I think it's important to note as
well is that like there's no gp8 you
might might argue that if they're going
to use all the GPT names why wouldn't
they just trademark GPT a and I think
maybe because like the document States
the model after gpt7 could be AGI and
I'm going to give you guys another
reason on top of that um another reason
is and I'm going to show you guys that
later on in the video but essentially um
open ey's timeline on super alignment
actually does coincide with this Theory
which is a little bit Co coincidental of
course like I said pure speculation
could be completely false open ey like I
said before can go ahead and completely
change their entire plans you know they
can go ahead and drop two models in one
year the point I'm trying to make is
that um certain timelines do align but
just remember this because I'm going to
come back to this because of some
documents stuff that you're going to see
in this document at the end of the video
so anyways um you know it says Elon Musk
caused a delay because of his lawsuit
this why I'm revealing the information
now because no further harm can be done
so I guess Elon musk's lawsuit has kind
of um you know if you wanted bought you
some time so he says I've seen many
definitions of AGI artificial general
intelligence but I will Define AGI
simply as an artificial general
intelligence that can do any
intellectual task a smart human can this
is how most people Define the term now
2020 was the first time that I was
shocked by an AI system so this is just
some um of course you know talk about
his experience with you know AI systems
I'm guessing the person who wrote this
but you know AGI if you don't know AGI
is like an AI system that can do any
task human can but one thing that is
important to discern is that you know
AGI there was a recent paper that
actually talks about the levels of AGI
and I think it's important to remember
that AGI isn't just you know one AI that
can do absolutely everything there are
going to be levels to this AGI system
that we've seen so far and in this paper
levels of AGI they actually talk about
how you know we're already at emerging
AGI which is you know emerging which is
equal or somewhat better than an
unskilled human so we are at level one
AGI and then of course we've got um you
know competent AGI which is going to be
at least 50% of the 50th percentile of
skilled adults and that's competent AGI
that's not yet achieved and then of
course we've got expert AGI which is
90th percentile of skilled adults which
is not yet achieved then we've got
virtuoso AGI which is not yet achieved
which is 99th percentile of all skilled
adults and then we've got artificial
super intelligence which is just 100% so
I think it's important to understand
that there are these levels to AGI
because once someone says AGI I mean is
it 90 9 can it do like half you know
it's like it's just it's just pretty
confusing but I think this is uh a
really good framework for actually
looking at the definition because trust
me it's an industry standard but it is
very very confusing so here's where he
basically says that you know um you know
GPT 3.5 which powered the famous chat
GPT and of course gpt3 which was the not
the successor but the predecessor of 3.5
it says you know these were a massive
step forward towards AGI but the note is
you know gbt2 and all chatbot since
Eliza had no real ability to respond
coherently so while such gpt3 a massive
leap and of course this is where we talk
about parameter count and of course he
says deep learning is a concept that
essentially goes back to the beginning
of AI research in the 1950s first new
network was created in the 50s y y y so
basically this is where he's giving the
description of a parameter and he says
you may already know but to give a brief
digestible summary it's a nalist to a
synapse in a biological brain which is a
connection between neurons and each
neuron in a biological brain has roughly
a thousand connections to other neurons
obviously digital networks OB vular to
biological brains basically saying that
you know of course we're comparing them
but different but um how many synapses
or parameters are in a human brain the
most commonly cited figure for synapse
count in the brain is roughly 100
trillion which would mean each neuron is
100 billion in the human brain has
roughly 1,000 connection and remember um
this number 100 trillion because it's
going to actually be a very very big
number that uh you do need to remember
so of course you can see here the human
brain consists of 100 billion urans and
over 100 trillion synaptic connections
okay and essentially this is trying to
you know um just pair the similarities
between parameters and synapses so
entially stating here that you know if
each neuron in a brain and trust me guys
this is just all going to come into
everything like I know you guys might be
thinking what is the point of talking
about this I just want to hear about qar
but just trust me all of this stuff it
does actually make sense like I've read
this a lot of times so I'm going to skip
some pages but the pages I'm talking
about now just trust me guys you're
going to want to read them it basically
says here if each neuron in a brain has
a th000 connections this means a cat has
roughly 250 billion synapses and a dog
has roughly 530 billion synapses synapse
count generally seems to predict to
intelligence with a few exceptions for
instance elephants techn have a higher
signups count than humans but yet
display lower intelligence of course
basically here's where he's actually
talking about how you know the simplest
explanation for larger signups accounts
with lower intelligence is a smaller
amount of quality data and from an
evolutionary perspective brains are
quote unquote trained on billions of
years of epigenetic data and human
brains evolve from higher quality
socialization communication data than
elephants leading to our Superior
ability to reason but the point he's
trying to make here is that you know
while there are nuances that you know
don't make sense synapse count is
definitely important and I think we've
definitely seen that um with the
similarities in the parameter size with
the explosion of llms and what we've
seen in these multimodal models and
their capabilities and it says again the
explosion in a capabilties since the
early 20110 has been a result of far
more computing power and far more data
gbt2 had 1.5 billion connections Which
is less than a mouse's brain and DBT 3
had 175 billion connections which is get
somewhat closer to a cat's brain and
obviously it's intuitively obvious that
an AI system the size of a cat's brain
would be superior to a system than the
size of a mouse's brain so so here's
where things start to get interesting so
he says in 2020 after the release of the
175 billion parameter gbt 3 many
speculated about the potential
performance of a Model 600 times larger
at 100 trillion parameters just remember
this number because this number is about
to just keep you know repeating in your
head and of course he says the big
question is is it possible to predict AI
performance by parameter count and as it
turns out the answer is yes as you'll
see on the next page and this is where
he actually references this article
which is called extrapolating GPT and
performance by lrien and it was not
score written in 2022 and basically it
talks about how as you scale up in
parameter count you approach Optimal
Performance so essentially this graph
seems to be illustrating the
relationship between neuron networks
measured by the number of parameters
which can be thought of as the strength
of connections between neurons and their
performance on various tasks and these
tasks included language related
challenges like translation read and
comprehension and question and answering
among others and the performance on
these task is measured in the vertical
axis higher values indicating better
performance and the graph shows that as
the number of parameters in increases
the performance on these tasks also
tends to but of course it does have
diminishing returns As you move right
because the curves actually do tend to
Plateau as they reach the higher
parameter counts of course the various
colors on this chart just essentially
represent different tasks and each dot
on those lines represents a neural
network model of a certain size and
certain parameter count being tested on
that you can see right down here this is
where the trained G you can see the gbt
performance so it says flop us TR at gp3
and then of course you can see right
here this is apparently the number of
synap in the brain and just remember the
number 100 trillion or 200 trillion
because it's going to be really
important so s it then says as Lan
Illustrated extrapolations show that air
performance inexplicably seems to reach
human level at the same time as a human
level brain size is matched with the
parameter count his count for the
synapse the brain is roughly 200
trillion parameters as opposed to the
commonly cited 100 trillion figure but
the point still stands 100 trillion
parameters is remarkably close to
Optimal by the way an important thing to
not is that although 100 trillion is
slightly suboptimal in performance there
is an engineering technique that openi
is using to bridge this cap and I'll
explain this towards the very end of
this document because it is crucial to
open ey is building and Lan's post is
one of many similar posts online it's an
extrapolation of Performance Based on
the jump between previous models and
open ey certain has much more detailed
metrics and they've come to the same
conclusion as lanon as I'll show later
in this document so if AI performance is
predictable based on parameter count and
100 trillion parameters is enough for
human level performance when will 100
trillion parameter AI model be released
in the future so here's where we go okay
it says that gbt 5 achieved Proto AGI in
late 2023 with an IQ of 48 now that is a
statement that um you know with the IQ
of 48 I genuinely don't know where
that's coming from I'm guessing that's
maybe just based on addictions and stuff
like that but I genuinely don't know
where this like in the beginning there
was this uh you know IQ thing where it
was like you know IQ for get IQ 96 delay
I I genuinely don't know where those IQ
values are coming from it doesn't really
state in this document unless I missed
it somewhere but um essentially this is
a point where things start to get super
interesting because this is where he's
referencing a whole bunch of sources but
it says that you know um of course Jimmy
apples did tweet a has been achieved
internally if you didn't watch the video
that I made on a has been achieved
internally Jimmy apples wasn't the only
one that tweeted this um Sam when
actually himself did actually post this
on Reddit and then he edited the comment
to say look we were just joking around
um and then of course this person this
is a guy who uh runs Runway he said that
I have been told that gbg 5 is scheduled
to complete training this December and
that op AI expects it to achieve AI
which means we will hotly debate whether
or not it actually will achieve AI which
means it will and of course you can see
um this guy is CEO of Runway and a bunch
of other companies and it's actually
funny enough he actually is followed by
Sam Alman so um this statement actually
wasn't like there weren't many
detractors of the statement which was
quite interesting considering that
that's just a bold statement about gbt 5
nonetheless it was a very interesting
statement anyways we continue on in this
document and it states the first mention
of a 100 trillion parameter model being
developed by open AI was in the summer
of 2021 mentioned offand in a wide
interview by the CEO of cerebrus Andrew
Feldman a company which is Sam Alman is
a major investor of and if you don't
know what the cerebrus company does uh
they produce crazy crazy clusters um and
you can see right here that they have
the fastest HPC accelerator on Earth you
can see that the latest GPU can theirs
is pretty pretty insane and I'm guessing
that they're looking to deploy these in
the future based on future AI systems
essentially this basically talks about
the first mention of an 100 trillion
parameter model being deployed whichin
the summer of 2021 and you can see that
it's mentioned in an offhand wide
interview by the CEO cerebrus like I
just talked about which Sam Alman is a
major investor of this is actually
pretty true samman actually has lested I
think it's between 50 million to 80
million um and I think open has actually
agreed to purchase some of the chips
some kind of deal but it says from
talking to opening ibt4 will be about
100 trillion parameters that won't be
ready for several years and remember
this was of course uh debunked if you
were in the space at the time if you
were actually paying attention to things
but anyways if we continue okay because
I want to get through some of this stuff
it says Sam alman's response to Andrew
Feldman at an online Meetup at ac10 it's
crucial to note that Sam Alman admits to
their plans for 100 trillion parameter
mod so this is an essentially an excerpt
from that blog post interview and it
says gb4 is coming but the current focus
is on the Codex and also where the
available computer is coming gb4 will be
a text model as opposed to multimodal
and it says okay this is where things
get interesting okay pay attention to
this it says 100 trillion perameter
model won't be gb4 and is far off
they're getting much more performance
out of smaller models maybe they may
never need such a big model and and they
then says it's not yet obvious how to
train a model to do stuff on the
internet and think long and very
difficult problems and a lot of work is
going to make it accurate until tell the
truth so basically this part is to look
at the early stages where um Sam Alman
is basically stating that you know they
do have plans for an 100 trillion
parameter model which is essentially
this brain-like AGI type system now
here's we where we talk about some more
leaks and of course things are very
speculative so of course like a said it
could be just speculation but it says an
AI researcher Igor made the claim a few
weeks later that gp4 was being trained
and would be released between December
and February again I will prove that he
really did have accurate information as
a credible Source this will be important
soon so you can see here he tweets that
open AI started to drain GPT 4 releas
his plan for December slfb then
essentially GW a famous figure in the AI
World he's an AI research and blogger he
messaged igle bov on Twitter in
September 2022 and this is the response
he received it's important to remember
the Colossal number of parameters text
audio images possibly video and
multimodal and this comes from a
subreddit called this is the way it will
be which is a private small sub
subreddit run by mathematicians with an
interest in AGI and a few AI enthusiasts
and it says they use this subreddit to
discuss topics deeper than what you'll
find in the main stream and you can see
here that he actually does talk about
how open AI started training gbt 4 and
the training will be completed in a
couple months and trust me guys I'm
going to get to the big bit but
essentially this is someone who had
early information on this model okay and
essentially a colossal number of
parameters it sounds like this guy was
referencing 100 trillion parameter model
as 500 billion parameter models and 1
trillion parameter models had already
been trained many times by the time of
this tweet in summer 2022 making models
of that size unexceptional and certainly
not colossal so these tweets from I'm
just going to say Ru because they
actually do make a similar claim which
is pretty interesting okay um so this is
where stuff gets even more interesting
okay because this guy okay just call him
rapu because I don't know how to say rxp
time but he says he also mentions an 125
trillion synapse gbt 4 however
incorrectly States the gbt 3's paramet
account as 1 trillion but essentially he
states that um you know it was far
behind the human brain containing 100
trillion synapses but what he did get
right was stating that it will be
introduced the public at the beginning
of 2023 and is able to write academic
pieces and articles that human
intelligence cannot distinguish so this
is another person that did have earlier
inside information now he does cite that
this is a weaker piece of evidence
because Rune is a fairly notable Silicon
Valley air researcher followed by CEO
Sam Alman and he did tweet at run I'm
guessing is gb4 100 trillion parameters
are rumored and then the guy thumbs it
up I'm not I'm not really sure what that
is referring to that much but
essentially the information from the
previous two people who had early
information about gbt 4 were then
basically sent to this person okay it
says in November 2022 I reached out to
an AI blogger named Alberto and his post
seemed to spread pretty far online so I
was hoping that I sent him some basic
gbt 4 he might be able to do a write up
so you can see they were actually
talking and he said of course the info
necessary isn't official but this is all
important just trust me because
essentially later on of this document
you'll see how the 100 trillion
parameter model being talked about now
is something that's been in the works
for a while and there are a few things
that do make sense so you can see here
that of course Alberto then goes ahead
to publish this where there this rumor
does start to spread and you can see
that then the 100 trillion parameter
leak went viral reaching millions of
people to the point that opening eyes
employees including CEO Sam Alman had to
respond calling it complete foolishness
called it factually Incorrect and of
course this guy of course did claim
responsibility for the leak because if
you remember at this time this was a
really really huge deal where people
were stating that this is going to be
really really huge but this isn't the
caveat it's not about them not getting
this wrong it's about the fact that GPT
4 is not going to be 100 trillion
parameters it's about that this document
is basically stating that in the future
GPT 8 whatever model it's going to be
the one that achieves AGI will actually
have this count and these guys knew this
from quite some time ago that's why
we're um essentially looking at this
kind of data in terms of the leaks and
stuff to kind of show you guys how we
actually got there so remember this
Eagle guy he was also stating that he
also heard about 100 trillion parameters
but he found that this was going to be
ridiculous so he decided not to include
it with his tweet about GPT 4 because
when he heard 100 trillion parameters he
was like wait that doesn't make sense
for GPT 4 because why would it be that
much bigger than gpt3 like it doesn't
make sense and then you can see this is
where you start to talk about you know
2022 where I became convinced that
opening I plan to release a one to2
trillion parameter subset of GPT 4
before releasing the full 100 trillion
parameter model GPT 5 so basically all
of these people said the same thing all
of these people who were early on GPT 4
somehow I'm not entirely sure stated
that this was all going to to be a model
in the future that would have 125
trillion parameters or over 100 trillion
parameters and essentially bringing it
back to Eagles leak you can see that
there were a couple of people that were
also using a beta version of GPT 4 now
of course it says the sources here are
varying credibility but they all
inexplicitly say the same thing gp4 was
being tested in October November of 2022
and according to the US military AI
researcher it was definitely being
trained in October which again lines up
with eagles's leap now essentially
what's crazy here is that it says
opening eyes of official position as
demonstrated by samman himself is that
the idea of 100 trillion parameter gbt 4
is complete false this is a half true
because gbt 4 is 100 trillion parameter
subset of the full 100 trillion
parameter model I'm not sure if I do
believe that statement because one of
the problems is is that of course GPT 4
isn't actually confirmed but you know
According to some articles it has been
confirmed that gbt 4 does have at least
1 trillion parameters I mean some people
have said that is 1.8 I mean some people
said it's 1.2 but here's where one of
the leaks that I do remember and this is
one one of the First videos I did make
on GPT 4 and it was because the CTO of
Microsoft Germany a week prior to the
official release of GPT 4 actually did
slip up and reveal that there exists a
GPT 4 which does have the ability to
process videos and it says I imagine he
was unaware of opening ey's decision not
to reveal the video capabilities of the
system and this completely proves that
gbt 4/5 was trained on not just text and
images but also video data and of course
we can infer that audio data was
included as well so he says we will
introduce gp4 next week I remember I
actually made this video if you go back
to the channel you can actually see this
and he says we will introduce GT4 next
week they will have multimodal models
that will offer completely different
possibilities for example videos and we
said the CTO called the llm a game
changer because they teach M to
understand natural language which then
understand in a statistical way what was
previously only readable and
understandable by humans in the meantime
the technology has come so far that it
basically Works in all language you can
ask a question in German and get an
answer in Italian and remember this was
actually a very very credible statement
because it was the CTO of Microsoft and
and we did actually get gbt 4 a week
later and the point again is that eagle
before said that GPT 4 was going to be
released in january/february and this
kind of claim was also cooperated by a
credible entrepreneur who stated that in
October 2022 gbt 4's release date would
between January and February 2023 now
some people are stating well gbt 4 was
released in March but of course you can
see right here although gbt 4 was
released in March 2023 slightly outside
the window I think it was done
intentionally by open ey to discredit
the leak and I I can't lie I actually do
agree with that because I've seen some
stuff you know tweeted by people like
Jimmy apples and stuff like that and I'm
pretty sure sometimes when leaks have
gotten pretty big open air have decided
against it because it just discredits
the leakers and I mean they're working
on some top secret stuff here now here's
where we get into the AGI debate it says
a note about robotics AI researchers are
beginning to believe that vision is all
that's necessary for optimal real world/
physical performance and in order to
give one example Tesla completely
ditched all censers and fully committed
div vision for their self-driving cars
the point is that training a humanized
AI model on on all the image and video
data on the internet will clearly be
more than enough to handle complex
robotic tasks that Common Sense
reasoning is buried in the video data
and just like it's buried in the text
data and the text focus gp4 is stally
good at Common Sense reasoning and it
does actually show an example of where
you know Tesla actually does remove its
ultrasonic sensors and then I'm going to
show you another slide where Tesla
actually do talk about how images all
they need SL video and you can see the
former head of Tesla AI where explains
they remove the sensors While others
differ that's Andre Kathy I believe and
this was another example if you remember
at the time and this is all going to tie
in So just pay attention essentially
palm e if you do remember this video
from around 2023 where essentially it
was AI system that learned mainly from
Vision combined with an large language
model and there were minimal robotics
data required on top of the language and
vision training and palm e was a 500
billion parameter model and it and it
asks the question that what happens when
robotics is trained on an 100 trillion
parameter model on all the data
available on the internet and this was a
pretty crazy system I'm not going to lie
to you guys like looking at pal how good
it was was it was definitely a very very
comprehensive system that could do a lot
of stuff I remember it was able to deal
with adversarial disturbances it was
able to you know get stuff that you
wanted it to do this was a pretty pretty
good system um they did upgrade this but
I'm surprised they haven't dropped any
updates recently because it was a really
good system for the time and of course
this is where they talk about the Tesla
update where it says you know Optimus
performed you know its first endtoend
learn successful graph today you can see
it below at 1X speed this was learned
from Human demonstrations no specific
task programming was done this means we
can now scale quickly to many tasks it
says join us before it's to late make
the AI happen with us it says if human
demonstrations are all that's needed for
advanced robotics performance and 100
trillion parameter model trained on all
the web would certainly be able to
achieve astonishing robotics performance
and I wonder what's going to happen in
the next couple of weeks cuz I'm not too
clued up on Robotics and how entirely
that is to trange but I do know that
next week or in fact two to three weeks
from now we're going to have a huge
robotics announcement where they're
basically you know one someone at one of
the leading robotics companies they
actually did state that we thought we
didn't have the data but now we do and
that they've made a breakthrough so I
wouldn't be surprised if soon we do get
a real update because someone said that
3 weeks from now someone ATL said you
know there going to be a huge update
that's going to completely change
robotics so before you actually you know
discredit this area I would say give it
like 3 weeks because we don't know now
essentially it says the image on the
left shows what aund what a trillion
parameter GPT 4 is capable of an image
recognition which is pretty crazy if you
haven't seen this demo before this is in
the gbt 4 paper where they talked about
how good this model is at understanding
things
and it's already clearer and written
more well than many humans would have
been able to come up with so it says so
again what happens when you train a
model 100 times larger than gbt 4 which
is the actual size of the human brain on
all the data available on the internet
and then we get to this slide where it
says important notice how the AI model
is able to generate multiple angles of
the same scene with physically accurate
lighting and in even some cases
physically accurate flu fluid and rain
and if you can generate images and
videos with accurate Common Sense
physics you have common sense reasoning
and if you can generate common sense you
understand common sense and I mean this
is once again one of those points where
it's delving into the argument of if an
AI system can accurately do lighting if
it can understand um you know physics
and rain and all that kind of stuff this
is meta's video thing it's basically
saying that does the AI have a common
sense World model in its head where it
can truly understand how the world you
know is and how it interacts and how you
know um like what kind of reasoning does
it use in his head to be able to get
this uh well okay and some people say
that you know some people say these AI
systems just patent recognition
it's just regenerating stuff it's seen
in this training data but some argue
that it's able to completely understand
the physical world that we live in and
it's got some kind of world model it's
got some kind of Common Sense thinking
algorithm whichever way you want to put
it so that it can completely get this
stuff correctly because it needs to be
able to if it wants to and I mean that's
something again that is really debated
on now here it gives us some talks about
someone that did give the early leaks
for GPT 4 again and what's crazy is that
they do talk about image and audio
generation would be trained in quarter 3
of 2023 and apparently If Video
generation training is simulation
simultaneous or shortly after this
actually does line up with Chen's claim
of gbt 5 being finished training in
December of 2023 so remember that guy
before this year of Runway stating that
gbt 5 would be finished training in
December was now around 3 months ago
that actually does line up with some of
the claims made here and this is also
someone that did have early inside
access so we can see clearly that there
are do people that do have inside access
now here's where we get into the crazy
bit because this is where um we talk
about long-term planning and of course
things do change but I think this is
going to be the most important part of
the video but it says here the open ey
president Greg Brookman stated that in
2019 following a$1 billion investment
from Microsoft at the time open ey
planned to build a human brain sized
model within 5 years and that was their
plan on how to achieve AI remember that
bit guys of plan to build a humaniz
brain model within 5 years and that was
their plan for how to achieve AGI you
can see um you know within 5 years and
possibly much faster with the aim of
building a system that can run run a
humaniz braid model and of course that's
why I said before the same number 100
trillion parameters is the reason that
number is being cited a lot and that's
why I'm guessing if open ey had
previously stated in 2019 that their
investments from Microsoft would
actually help them to try and build a
human a humanized brain within 5 years
you know leaks about 100 trillion going
viral and then it's spreading about GPT
4 could actually be the reason that
actually occurred because many people
were confused thinking gbd4 is going to
have 100 trillion but many people didn't
realize that this is actually the future
models which is what open have been
planning all along and I think that
actually does make sense like if we can
actually corroborate statements and
understand what's actually being said
from actual openai employees I think
those are the best chances we do have at
looking at what is realistic you can see
here it says both of these sources are
clearly referring to the same plan to
achieve AGI a human brain sized AI model
trained on images text and other data
due to be trained within 5 years of 2019
so by 2024 and it seems to line up with
all the other sources I've listed in
this document so essentially that's what
Greg Brockman did state which is a
pretty pretty hefty piece of information
and yeah that would actually line up
with 2024 and this is where we start to
get into the part where certain people
start to urge caution on AI and this is
the part where he starts to argue that
you know suddenly AI leaders are
starting to sound the alarm almost like
they know something very very specific
that the general public doesn't and I
would argue that yes is kind of true but
you know AI leaders have been stating
this from ages ago like Sam Alman has
been stating since 2015 AI is dangerous
and Elon mus has been stating the same
thing but I would argue as well that
certain people from Google did actually
leave recently um and we're going to get
into that now they actually did leave
fairly recently and I'm not surprised
but um they actually did really leave
recently and maybe it's just a
coincidence and part of it but it says
you know in this uncertain climate that
hassabis agrees to a interview stock
warning about his growing concerns I
would Advocate not moving for and
breaking things he says referring to an
old Facebook model and essentially their
old motto was you know release
Technologies into the world first and
then fix any problems that arose later
and of course you can't do that with
super intelligence and he says he says
AI is now on the cusp of being able to
make tools that could be dation urging
his competitors to proceed with caution
than ever before and of course Jeffrey
Hinton if you don't know this guy um by
industry standards The Godfather of aiir
that he actually did leave Google in
2023 because he wanted to actually talk
about the dangers of AI he said the idea
that this stuff could actually get
smarter than the people a few people
believe that Hinton said in the
interview but most people thought it was
a way off and I thought it was way off I
thought it was 30 to 50 years away or
even longer obviously I no longer think
that and he thinks super intelligence is
Coming Far quicker than we did think now
if you remember as well this is very
fascinating because at the time this was
something that was pretty crazy and it
says shortly after the release of gbt 4
the future of Life Institute a highly
influential nonprofit organization
concerned with the mitigating the
potential C catastrophic risks to the
world released an open letter on all AI
labs to P AI development for 6 months
why because they essentially stated that
you know including the currently being
trained GPT 5 and this is kind of crazy
I didn't actually see that okay it says
we call on all AI labs to immediately
pause Training Systems more powerful
than GPT 4 including the currently being
trained gbt 5 signatures include you
know all of these people that were like
El musk that even worked at Apple and
apparently the first release version of
the letter specifically said including
the currently being trained gbt 5 and
then apparently it was removed which is
pretty crazy and then of course there is
a quote from samman like I said again um
and this is pretty crazy because it says
you know do we have enough information
on the internet to create AGI and Sam
alman's blunt immediate response
interrupting the man asking the question
is yes he elaborates yes we're confident
there is we think about this and we
measure it quite a lot uh information in
the internet to create AGI so if you
contrast it with yes we have a
continuous video feed to our eyes and um
on the internet we only have like a
subset of that yeah we're confident
there is we think about this and measure
it quite a lot what gives you that
confidence is it the
size of the knowledge base is it
complexity is it one of the things that
I think open AI has driven in the field
um is that's been really healthy is that
you can treat scaling laws as a
scientific prediction you can do this
for compute you can do this for data but
you can measure at small scale and you
can predict quite accurately how it's
going to scale up how much data you're
going to need how much compute you're
going to need how many parameters you're
going to need when you know when the
generated data gets like good enough to
be helpful um the internet is like
there's a lot of data out there there's
a lot of video out there too few more
questions maybe so I mean you did see
from that clip how samman actually did
talk about it I mean he seems very very
very confident on that prediction in
order to get to AGI the kind of data
that they do need and then here's what
he says on Sam alman's Q&A he says that
you know first of all he seems highly
highly confident that exists enough data
on and AGI system confident to the point
that it makes one question if they've
already done it or in the process of
doing it which is definitely a bold
statement to state that they've you know
basically done it in 2022 in fact that
interview was actually from the early
stages of 23 which is quite fascinating
but then again um gb4 did finish
training at that time so they would
definitely be working on other AI
systems at that point now essentially
what he talks about here is that um and
I think this is you know probably the
thing that you should probably take away
from this video and it says as I
mentioned earlier in the document an 100
trillion parameter model is actually
slightly subtable but there is a new
scaling Paradigm openai is using to
bridge this Gap and it's based on
something called the G scaling laws
chinchilla was an AI model unveiled by
Deep Mind in early 22 and the
implication of the chinchilla research
paper was that current models are signif
trained and with far more computes
meaning more data we would see a massive
boost in performance the need to
increase parameters point is that while
an untrained or undertrained 100
trillion parameter model may be slightly
suboptimal if it were trained on vastly
more data it would be able to exceed
human level performance and the
chinchilla Paradigm is widely understood
and accepted in the field of machine
learning but just just to give a
specific example from openi president
Greg Brockman discusses this in an
interview on how openi realized their
initial scaling laws were flawed and
have since adjusted to take the
chinchilla laws into account regulations
to figure out like hey how big of a
model do you think you're going to need
lots of mistakes for sure um like a good
example of this is the scaling laws so
we we did this study to S to actually
start to really scientifically
understand how do models improve as you
push on various axes so as you pour more
Compu in as you pour more data in and
one conclusion that we had at one point
was that basically uh that there's you
know sort of a limited amount of of data
that you want to pour into these models
and that there's kind of this very this
very clear curve um and that one thing
that that I we realized only years later
was actually that we'd read the curves a
little bit wrong and you actually want
to be trading for way more tokens way
more data than anyone had expected and
that that uh you know there's definitely
these moments where these things that
just didn't quite click whereas like it
just didn't add up that we were training
for so little and that you know
something the conclusions that you drew
Downstream um but then then you realize
there was a foundational assumption that
was wrong and suddenly things make way
more sense I think it's a little bit
like you know physics in some sense
where like do you do you doubt physics
it's like I kind of do I think all of
physics is wrong right but like only so
wrong right it's like we clearly haven't
reconciled like Quantum and relativity
so there's like something wrong there
but that that wrongness is actually an
opportunity it's actually a sign of you
have this thing it's already useful
right it really like has affected our
lives and it's actually like pretty
great I'm very happy with what physics
has done but also there's fruit and so I
think that that for me that's always
been the feeling that there's something
here and that you know if we do keep
pushing and somehow the scaling laws all
Peter out right they suddenly drop off a
cliff and we can't make any further
progress like that would be the most
exciting time in this field because we
would have finally reached the limit of
Technology we would have finally finally
learn something and then we would
finally have a picture of what the next
thing to do is and yeah you can clearly
see that Greg Brockman discusses how
their initial scaling laws were flawed
and of course a lot of people were
stating at this time that training a
compute optimal 100 trillion parameter
model would have cost billions of
dollars and just isn't feasible well
Microsoft invested $10 billion into
openi in early 2023 so I guess it's it
isn't that ridiculous of a possibility
after all the only question I do have
but then again I don't really have that
question anymore after reading the
lawsuit from yesterday was that you know
of course Microsoft does get access to
only pre AGI Tech but then again open AI
is the one that gets to determine
whether the technology is Agi or not
part of me even wonders if you know
Microsoft will even exist or even some
of these companies want AGI exist
because you know AI could potentially
make competition irrelevant that's
another question for another video but
essentially Alberto Romero worked about
deep min's chinchilla scaling
breakthrough and chinchilla showed us
that despite being vastly smaller than
gbt 3 and deep mind's own gopher it
outperformed them as a result of being
trained vastly on more data just to
reiterate this one more time although a
100 trillion model is predicted to
achieve slightly suboptimal performance
open AI is well aware of the chinella
scaling laws as is pretty much everyone
in the AI field and as they're training
Q Star as a 100 trillion parameter model
that is compute optimal and trained on
far more data than they really intended
they have the funds to do it now through
Microsoft and this will result in a
model that is far far exceeds the
performance of what they had initially
planned for their 100 trillion parameter
model and 100 trillion parameters
without the chinchilla scaling laws
equal roughly human level but slightly
suboptimal but 100 trillion parameters
multimodal with chinchilla scaling laws
taken into account what on Earth do we
get and I think um I'm not that glued up
on the chinella scaling laws of have
been being completely honest I wasn't
aware of this but what I do know is that
if this statement is true and if the
research is up to date because I do know
that a research is a very complex field
and it is a very Dynamic field and
research paper every single day will be
changing how future system are trained
and maybe there's a research paper that
was released you know just 20 minutes
ago or something there are literally
like 160 papers released every single
day which is absolutely insane but this
is a true fact that there were so many
research papers released every single
day the AI space isn't Dynamic enough
and these chinchilla scaling laws are
true and if they do now have the funds
to actually train something that is
going to be an 100 trillion parameter
model I'm guessing that this might have
been what potentially sparked that
letter that kind of breakthrough that
you know I guess you could say got Sam
out when fired and I'm guessing that
maybe something like this was
potentially the ignition to spark a lot
of stress at opening eye because they
actually did State Sam when in the
interview said look everyone at Open Eye
stressed because we're building super
intelligence I actually made a video on
that because I was like that's a crazy
statement to say um and of course at
around that time you know there was so
much drama and around that time they did
you know trademark the models and of
course you know trademarks could
absolutely nothing but I just find that
you know everything happening around
that time I just don't think that that's
a coincidence now what is crazy as well
is uh this is where they actually do
talk about super alignment it says our
goal is to build roughly human level
automated alignment researcher we can
then use vast amounts of computer scale
our efforts and of course open AI has
planned to build human level AI by 2027
and then scale up super intelligence and
this has been delayed because Elam mus
lawsuit be coming up but short and
essentially he does talk about this and
I don't think this is that crazy um
essentially this guy is an open AI
researcher who worked there in the
summer of 2022 he joined there for one
year I'm not sure he works there anymore
but essentially he wrote a letter to
himself and essentially he said that you
know the company he's working on and
this is the kind of note that he wrote
to himself he said there's an AI company
building an AI that fills giant rooms
eats a Town's worth of electricity and
has recently gained an astounding
ability to converse like people it can
write essays or poetry on any topic it
can Ace college level exams it's daily
gaining new capabilities that Engineers
who tent to the AI can't even talk about
in public yet those Engineers do have a
sit in the cafeteria and debate the
meaning of what they're creating what
will it learn to do next week which jobs
might render it obsolete should they
slow down or stop so as to not tickle
the tail end of the Dragon but wouldn't
that mean that someone else probably
someone with less Scruples would wake
the dragon first is then ethical
obligation to tell the world about this
is then obligation to tell it less I AMU
are spending a year working at my job
well your job to developed the
mathematical theory on how to prevent
the AI and it successors from where that
essentially means destroying the world
and essentially he's referring to the
qstar multimodal 125 trillion parameter
B so essentially this document basically
just tries to say that look every year
we're going to get a GPT update till
2027 and the reason 2027 is the date is
because that actually does coincide with
opening eyes deadline to create super
alignment and they actually state that
they want to do this within 4 years and
since it was 2023 when they said this if
they do manage to get super alignment
done within 4 years that would be
2027 and considering they trademarks all
the way up to gpt7 that would mean that
if a new GPT model is released every
single year accounting for GPT 5 GPT 6
and gpt7 considering that's three more
years that takes us exactly to 2027 when
super alignment should be done and then
once we have you know I guess you could
say the system that will solve super
linman and we can actually solve that
then they can actually safely realize
that look we can safely train an AGI
level system or this 125 trillion
parameter model qar whatever it is
because then it would actually be safe
to do so so I'm guessing that kind of
does make sense with the iterative
deployment that we did see before I'm
guessing that if I'm being honest with
you guys maybe I did miss some key
things in this document maybe there's
some stuff that I'm going to miss but I
do think that certain things do make
sense like if they are iteratively
deploying things that does mean that you
know we're not going to get everything
that they do have they could have liter
no crazy crazy models and do remember
that samman in that interview he did
actually talk about how they're able to
predict the capabilities of future
models by training in a system which is
much less compute intensive and if you
remember this was something that I did
retweet and this was something that I
did talk about and I'm guessing that
whatever breakthrough that they had with
whether it was qar whether they realized
they just need a lot more comp I think I
think the elephant in the room is that
over the next couple of years we're
going to get iteratively better systems
which open air are going to release well
well after they've trained the models
because they stated that the society
needs time to adapt if you looked at the
Sora paper they did State one of their
employees in a deleted tweet stated that
you know we're going to release that
just to make sure that people can
reassess their timelines on what a ai's
capabilities are so I'm guessing that
they just wanted to release that to tell
us what's capable but the point is is
that they know where these a systems are
going to go because they have
predictable scaling and they've
accurately predicted GPT 4's capability
and I'm guessing if they've accurately
predicted all the way the capabilities
to GPT 5 6 and 7 even though during that
time things could really change because
ai's development field is so rapid I do
think that some of this stuff kind of
does make sense considering the fact
that they want super alignment solved in
the next four years and that would align
with 2027 when if we're continuing on
the release of a new llm model or
multimodal AI system each year which is
what they've already Trad mark it kind
of would make sense that we do get an
AGI system at that level one question I
do have is what does Sam need the 7
trillion for which is already we know to
be likely the AGI type system and I'm
guessing that if we're going to train
something that's potentially of course
you know really really compute intensive
you know at first I was thinking that
something compute as intensive as GPT 4
which was really really compute
intensive which is why they don't even
give us the message cap but with these
chinchilla laws now being four times
less I still think that they're going to
need a ridiculous amount of compute
because of course there is that compute
overhang which samman talked about which
essentially just means that there isn't
enough GP to go around there isn't
enough to actually provide the compute
but I'm guessing that with a 7 trillion
whatever Sam Alman has talked about in
these private interviews whatever he's
going to be you know pitching to these
investors I'm guessing that potentially
this could work now some of the caveat
that I do have about this is that of
course some of the information is just
unverifiable like you're not really able
to verify some of this stuff but I think
from this what we can take is that they
probably do know where AGI is in terms
of being able to predict the
capabilities and based on the statements
that they have said it does look like we
could be getting a model that is is
going to be a larger increase in
parameter count because it does seem to
be something that opening ey did State
and they did previously state that they
were going to build something that does
have the same amount of size as a human
brain however there were some caveats to
this cuz recently uh Google's AI boss
actually did say that scale only gets
you so far but then again samman is
trying to raise $7 trillion so I'm
guessing that maybe if scale isn't what
gets you so far Sam Alman wouldn't be
trying to raise $7 trillion if they
could just do it with the computer that
they did have at and so I'm guessing
that they do need scale because they've
already predicted it with less comput
and I'm just going to leave with that
but let me know what you guys thought
about this this is this wasn't meant to
be that long but I did want to cover
absolutely everything and I just wanted
to make this information available to
you I don't know whether or not this is
all going to be possible like I said
opening ey could go out and they could
change everything the truth is
regardless of all of this interesting
information and regardless of all of
this speculation the only people that
know what's going on is opening eye and
the people that work there but let me
know what you guys think about this do
you think this is going to be there do
you think this information is completely
false do you think this guy's got it
completely wrong do you think he's got
it completely right I'd love to know
your thoughts down in the comment
section below
5.0 / 5 (0 votes)
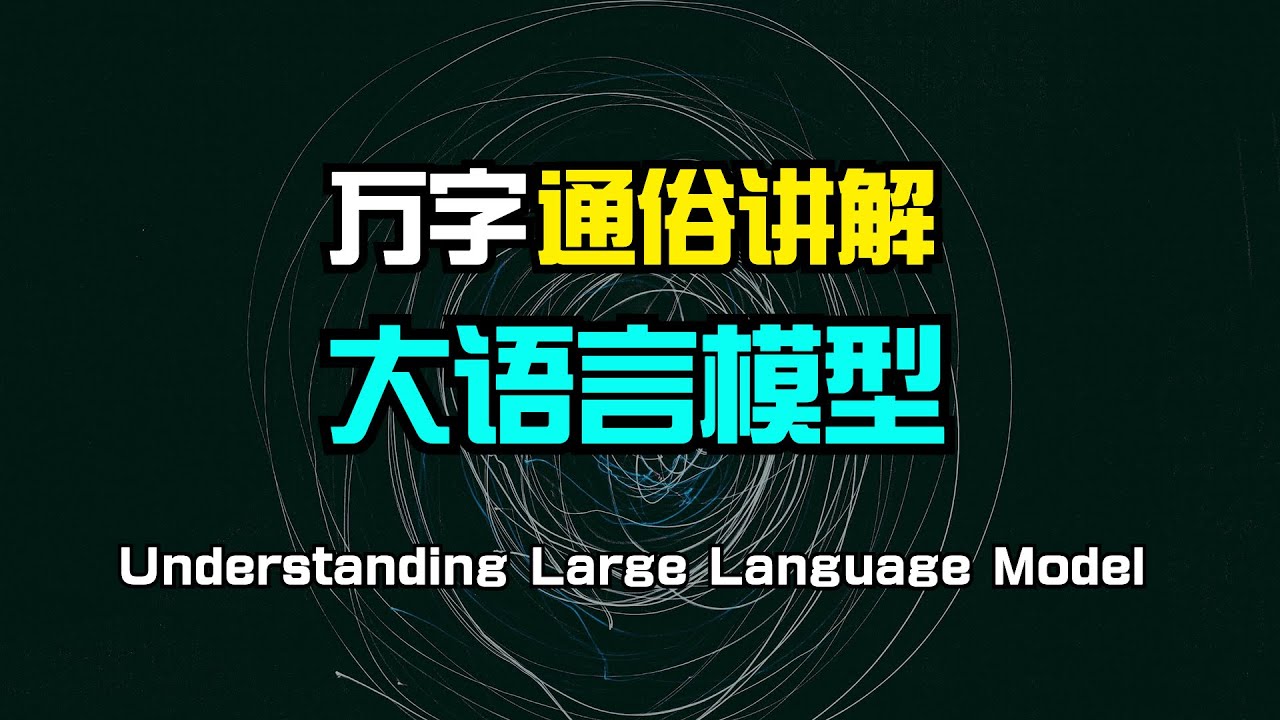
【人工智能】万字通俗讲解大语言模型内部运行原理 | LLM | 词向量 | Transformer | 注意力机制 | 前馈网络 | 反向传播 | 心智理论
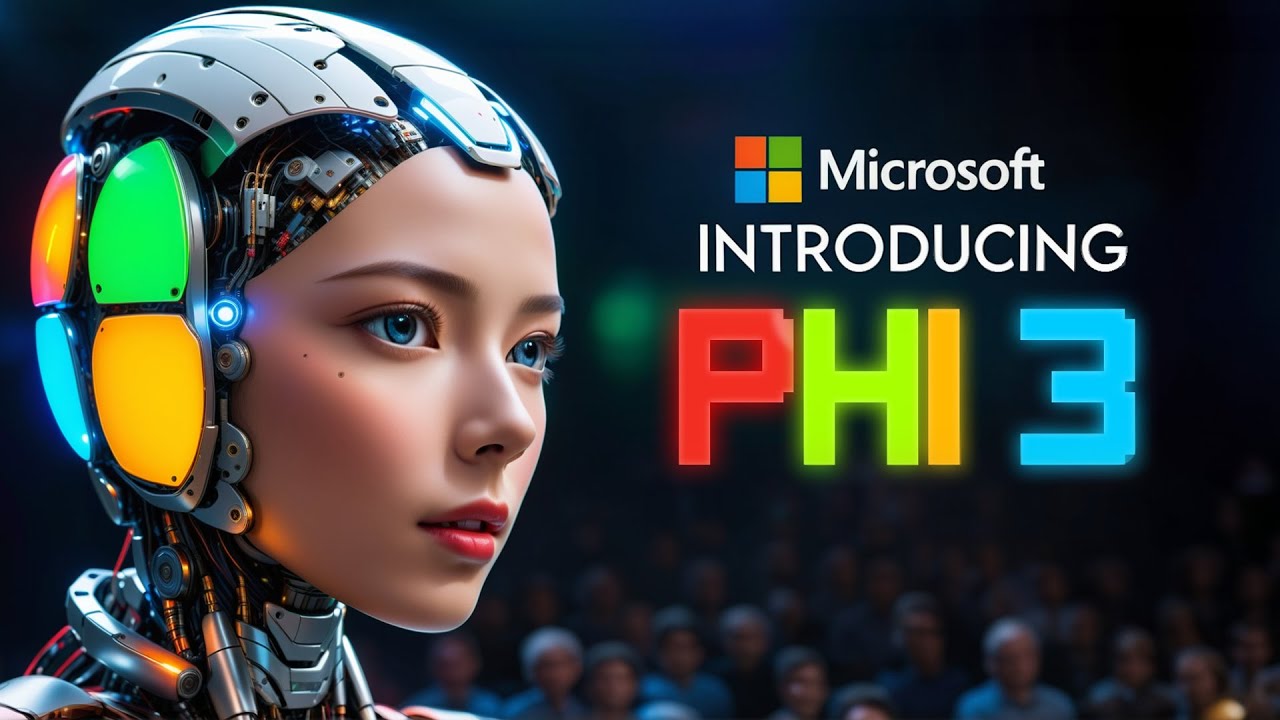
Microsoft's New PHI-3 AI Turns Your iPhone Into an AI Superpower! (Game Changer!)
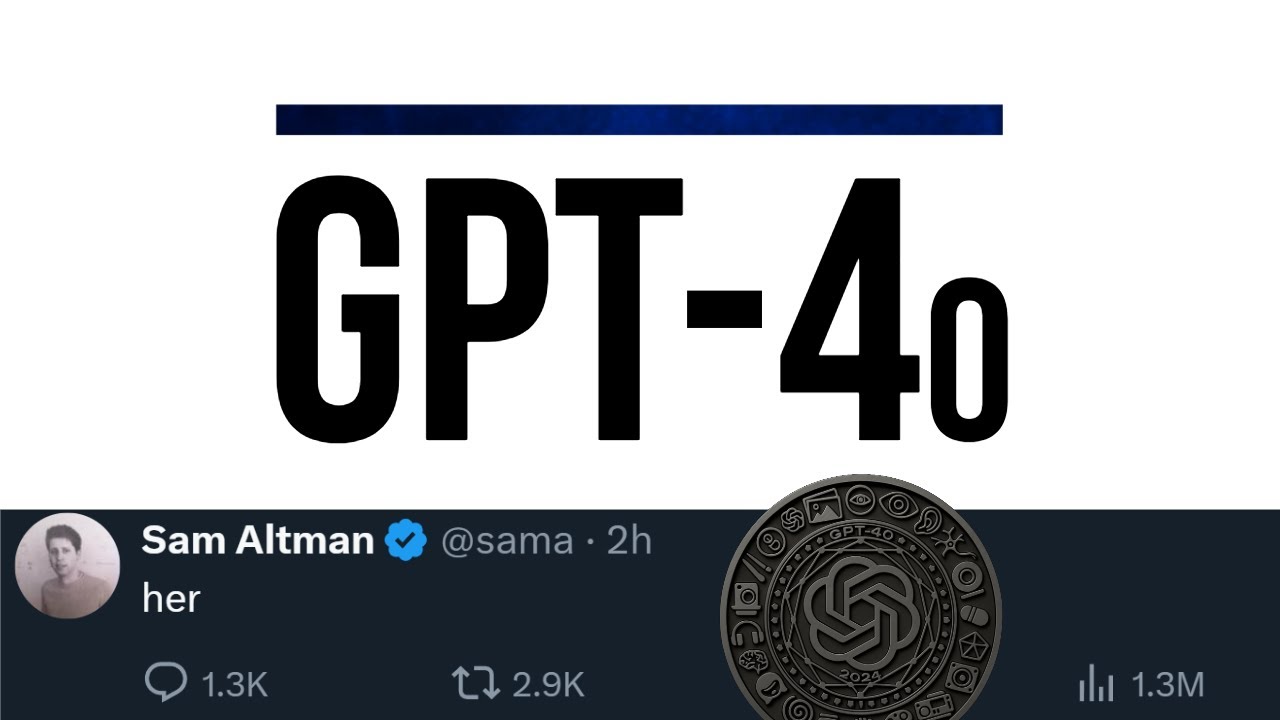
GPT-4o - Full Breakdown + Bonus Details
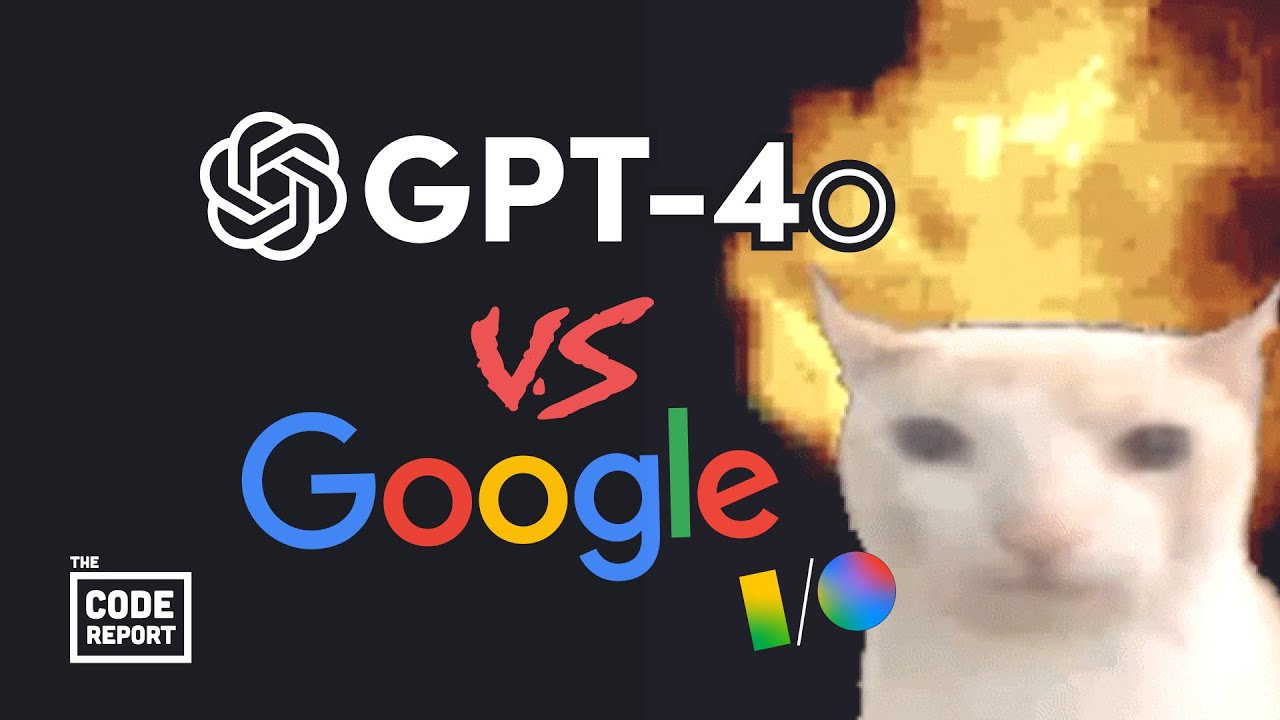
Another glorious battle for AI dominance… GPT-4o vs Google I/O
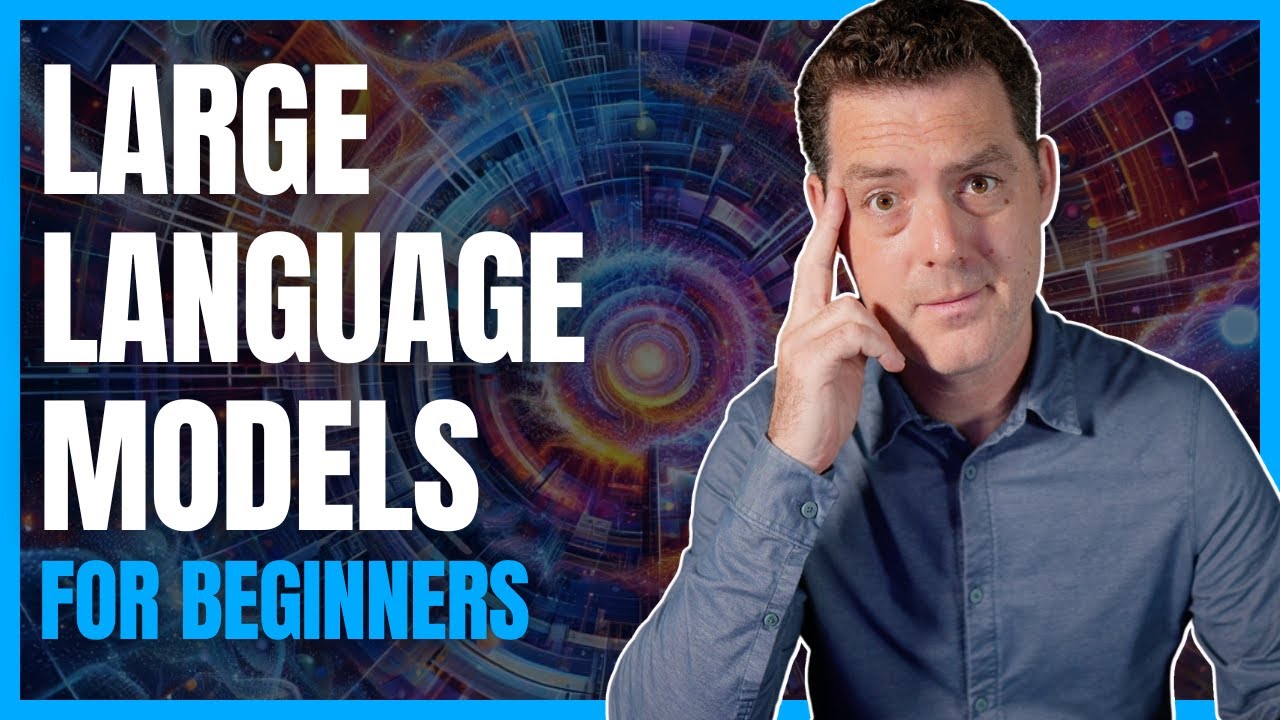
Simple Introduction to Large Language Models (LLMs)
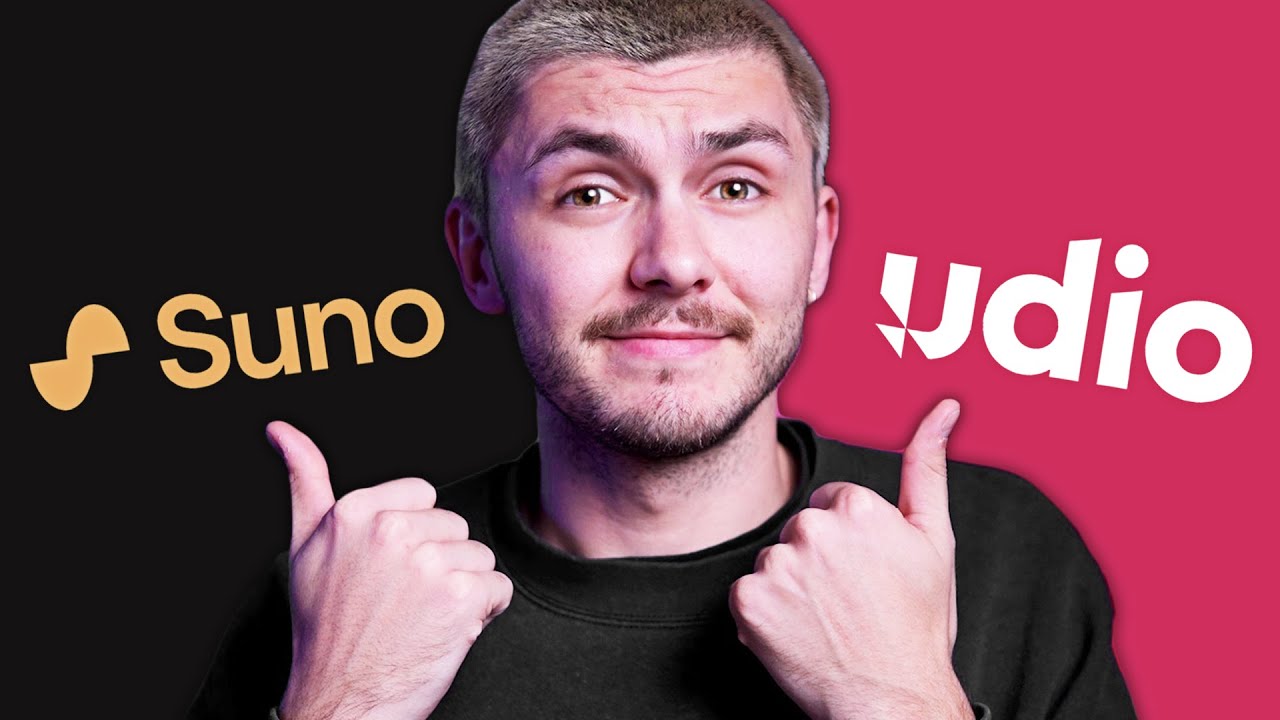
Best AI Music Generator in 2024 - SUNO vs UDIO